Comparative Analysis Between Macro and Micro-Accuracy in Imbalance Dataset for Movie Review Classification
Proceedings of Seventh International Congress on Information and Communication Technology(2022)
摘要
Classification for multi-class dataset provides exciting and explorative domain to be studied in data science domain. And yet, the challenges of measuring the accuracy of multi-class performance rise an issue worth detailed research to be explored. Due to multi-class accuracy may be lower due to imbalance dataset, this paper aimed to analyze the usage of macro and micro-accuracy in classifying text data with multi-class label. This research focused on text data of movie reviews being classified by three multi-class classifier which are Naïve Bayes (NB), Support Vector Machine (SVM), and Random Forest (RF). We set five performance measure to be analyzed; recall, precision, f-score, sensitivity and specificity with regards of micro and macro-accuracy. We successfully yielded a significant result of comparative analysis where average micro-accuracy (87.3%) produced 14.8% higher than macro-accuracy (72.5%) for imbalance dataset. Result also shown a significant gap between balanced and imbalanced dataset. For further analysis, the flexibility of class label in multi-class may be studied to obtain the changing of learning behavior of the classifier as future work.
更多查看译文
关键词
Multi-class classification, Macro and micro-accuracy, Text classification
AI 理解论文
溯源树
样例
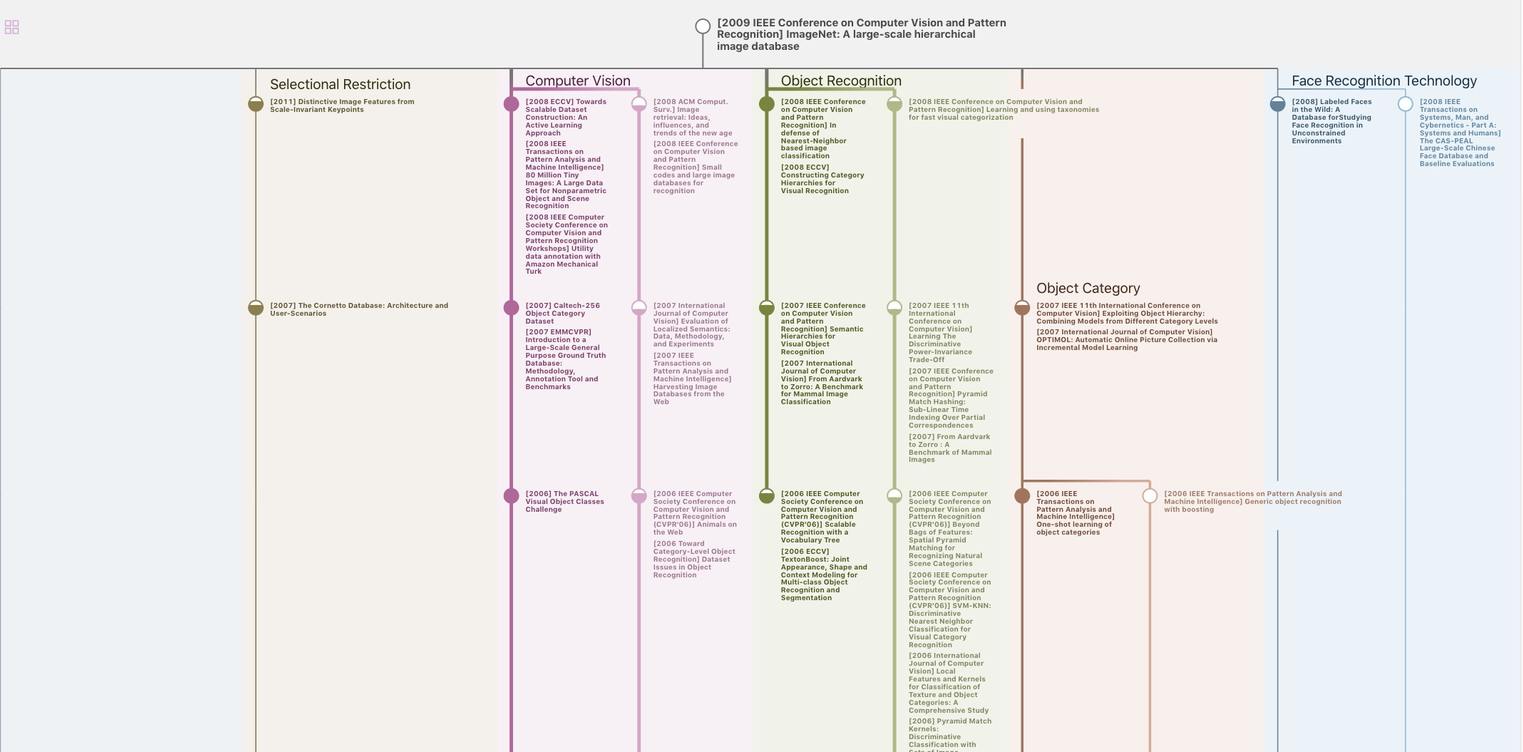
生成溯源树,研究论文发展脉络
Chat Paper
正在生成论文摘要