Augmented Feature Generation Using Maximum Mutual Information Minimum Correlation
Data Management, Analytics and Innovation(2022)
摘要
With size of datasets varying un-uniformly in sample size and feature length, to optimize the feature set usually different methods such as filter, wrapper methods are used. However, with different machine learning techniques though either feature reduction is used, or feature extraction is used, both have its own merits and de-merits. The proposed work proposes a hybrid model that tries to combine the feature extraction and feature reduction techniques thereby using both linear and non-linear techniques to take the best parts of both methods. After the initial ensemble is created still the feature set is further optimized by using the concept of entropy and information gain. Using mutual information, on further analysis the best non-redundant feature sets are selected after considering a specific threshold and using this as a testing tool the datasets are again analyzed to check the working accuracy. The model performance is found to be effective even using reduced feature sub-set. Also, it has been found apart from excelling in classification accuracy, the model has been successful in maintaining the range of the metric irrespective of the input size.
更多查看译文
关键词
Feature reduction, Feature extraction, Mutual information, Correlation, Classification
AI 理解论文
溯源树
样例
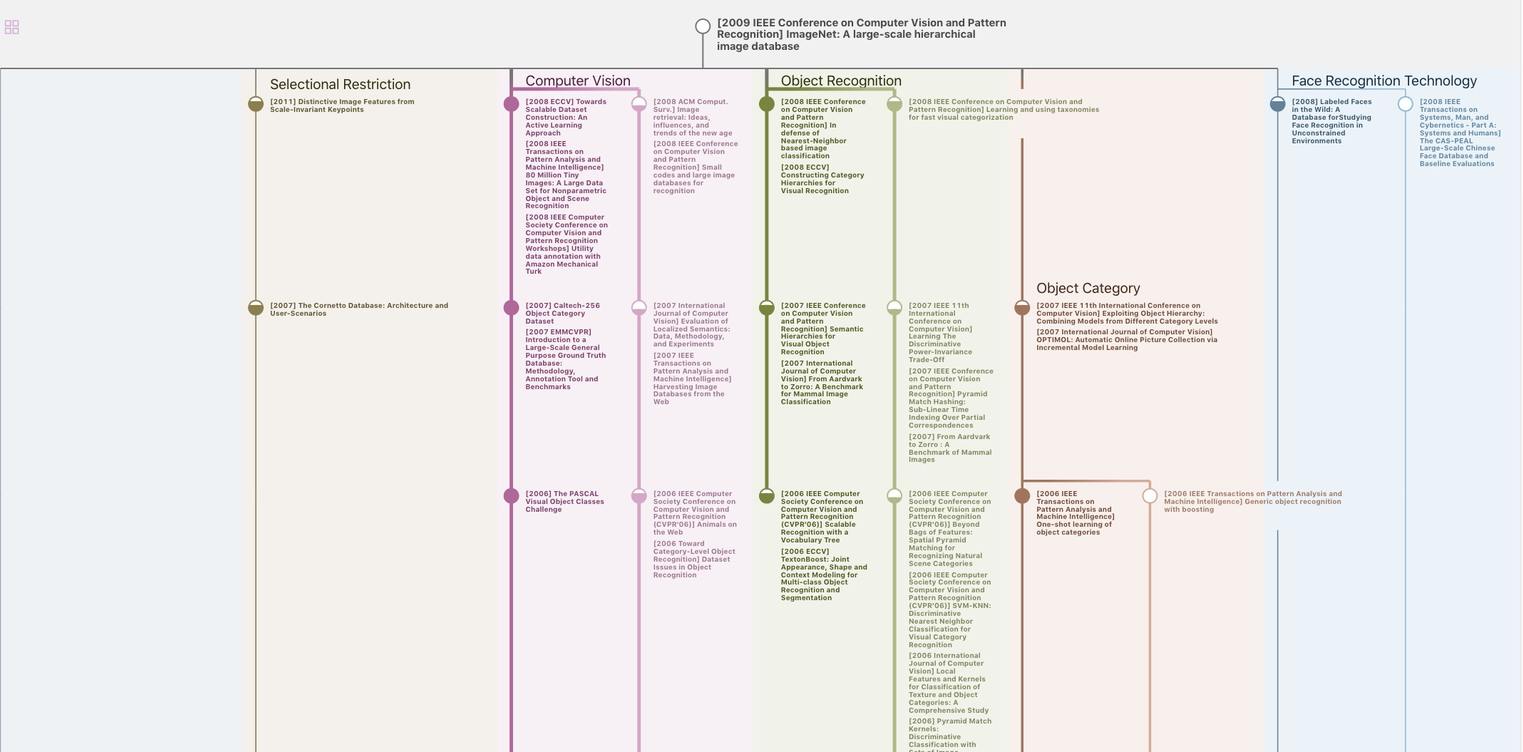
生成溯源树,研究论文发展脉络
Chat Paper
正在生成论文摘要