Pre-training Models Based Knowledge Graph Multi-hop Reasoning for Smart Grid Technology
Proceedings of 2021 5th Chinese Conference on Swarm Intelligence and Cooperative Control(2022)
摘要
With the continuous improvement of power technology, smart grid technology has been widely used in the power industry, effectively improving the reliability of power supply. The smart grid technology can help to store a large amount of data and provide basic data analysis but it cannot give a systematic representation method. While digging internal connections and knowledge, the smart grid technology seems helpless so it cannot provide auxiliary decision for the implementation of smart grid to achieve true ‘intelligence’. To solve this problem, we propose the pre-training models based knowledge graph multi-hop reasoning model which based on the knowledge graph of distribution network defects under smart grid technology. The model divides into two parts: the pre-training model and the multi-hop reasoning model. The former part is natural language pre-training model for extracting the entities related to the problem to be solved from the knowledge graph, and extracting the knowledge subgraph formed by these entities in the original knowledge graph. The later part is the neural network model which uses the knowledge subgraph extracted from the natural language pre-training model for relational reasoning to form the solution path of the problem and obtain the strongly correlated entities of the solved problem. To verify the model’s performance, we train four kinds of multi-hop reasoning models and test them in different-hop questions for transformer defect analysis of smart grid. Experimental results show that the proposed model can guarantee high accuracy under different reasoning difficulties which can effectively promote the auxiliary decision-making of smart grid.
更多查看译文
关键词
Smart grid technology, Knowledge graph, Natural language pre-training models, Multi-hop reasoning
AI 理解论文
溯源树
样例
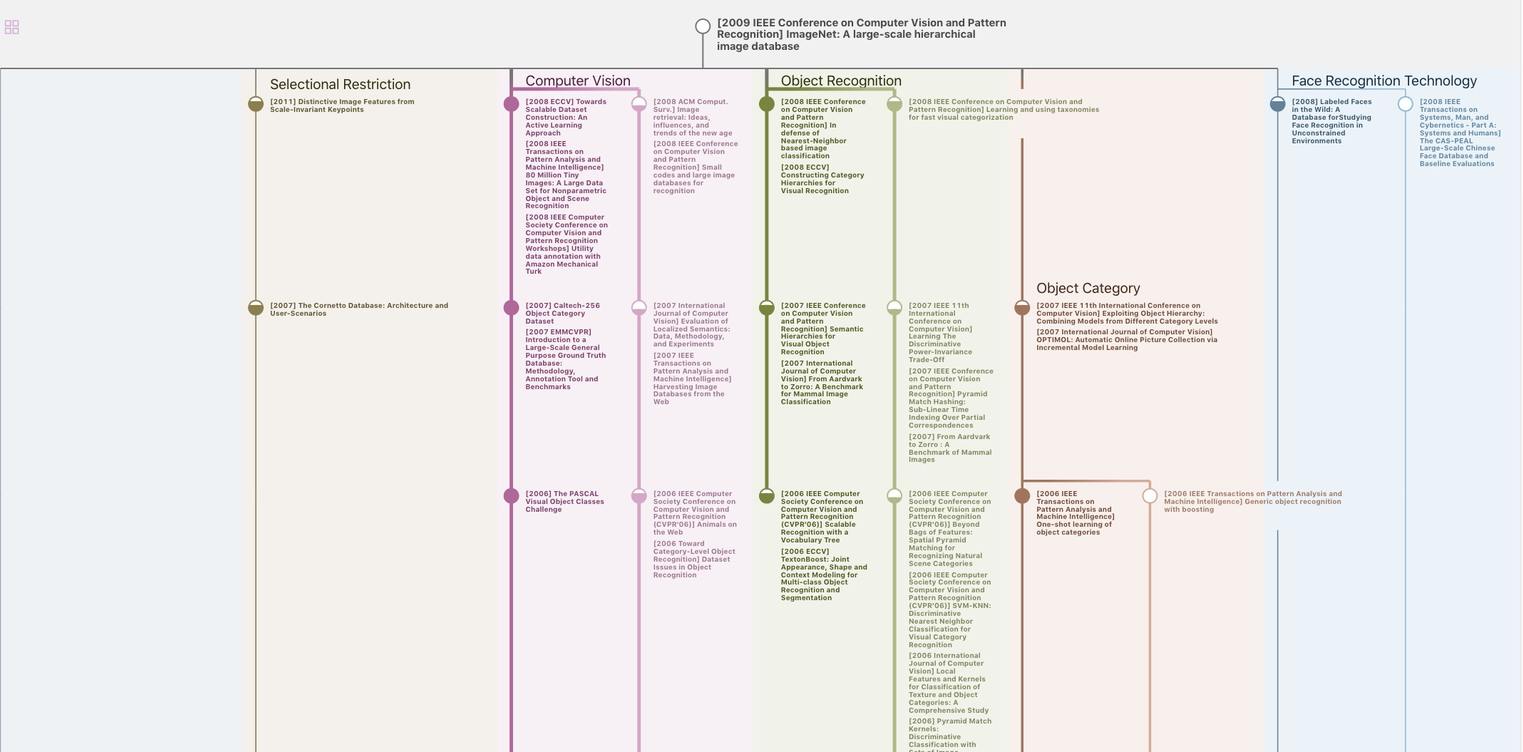
生成溯源树,研究论文发展脉络
Chat Paper
正在生成论文摘要