A Comparison of Algorithms for Bayesian Network Learning for Triple Word Form Theory
Computational Intelligence and Data Analytics(2022)
摘要
The triple word form (TWF) theory provides a formal framework for understanding the cognitive processes involved in reading and spelling. On the basis of this theory, spelling errors can be classified into different types, and by understanding the relationships between these error types, one can draw inferences about the difficulties students face while spelling. This paper examines data from 210 second-grade, bilingual students in Kerala, South India. These students participated in a spelling test, and their spelling errors on the test were classified according to the TWF theory. Bayesian networks were used to understand the relationships between the error types. This paper compares three algorithms that were used to study the structure of the Bayesian networks: a score-based algorithm, a constraint-based algorithm, and a hybrid algorithm. Using tenfold cross-validation, it was found that among these three algorithms, the score-based algorithm performed best in terms of expected loss.
更多查看译文
关键词
Triple word form theory,Cognitive spelling model,Bayesian network,Network structure learning,Cross-validation
AI 理解论文
溯源树
样例
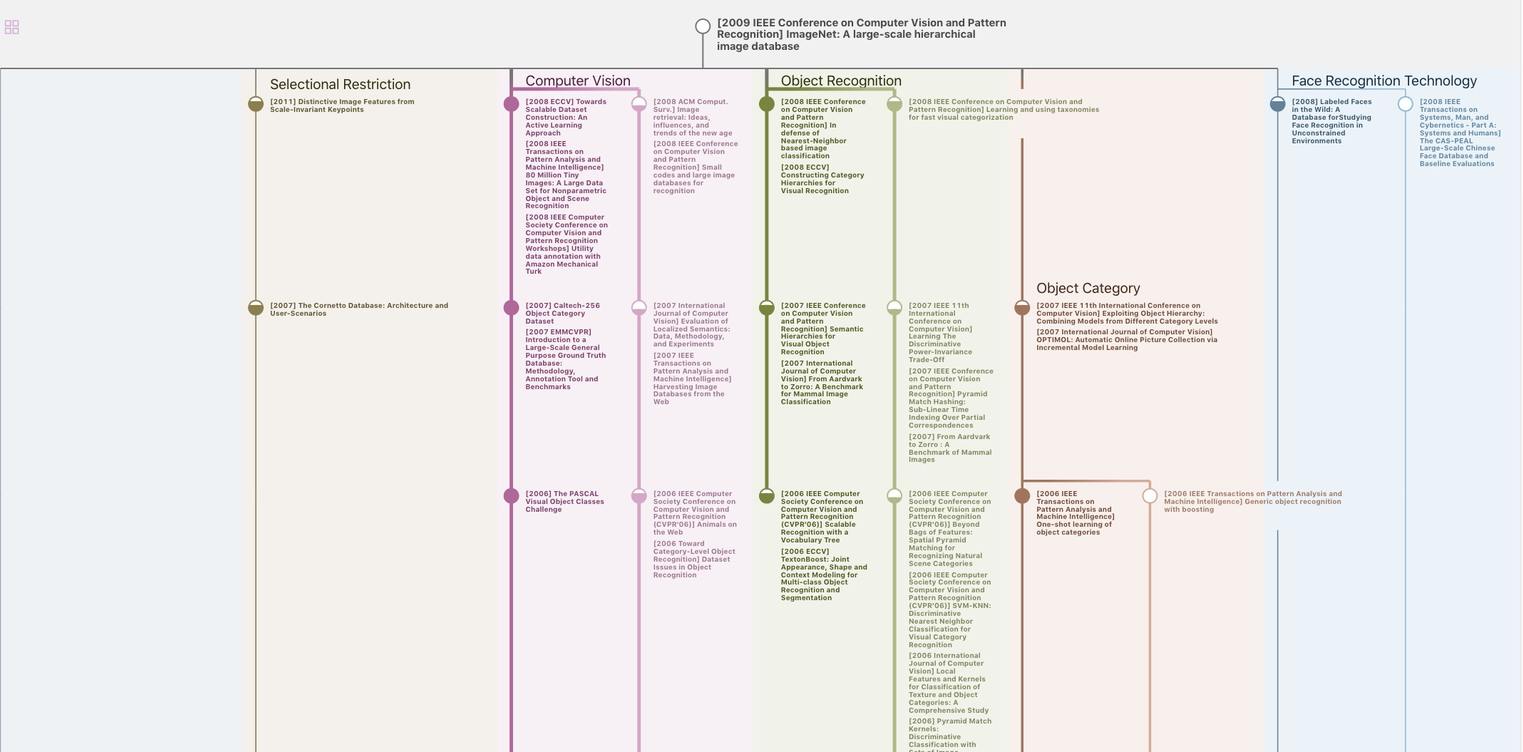
生成溯源树,研究论文发展脉络
Chat Paper
正在生成论文摘要