Anomaly Detection Using Feature Selection and Ensemble of Machine Learning Models
Computational Methods and Data Engineering(2022)
摘要
Vulnerabilities have increased in cyberspace. This is due to the technology growth and the huge amount of data communicated between various endpoints of a network. Intrusion Detection System (IDS) plays a major role in identifying malicious traffic in the network. Intrusion Detection is much challenging because of the raw network traffic it contains with a large number of attributes that add to the complexity of the model. Several Machine Learning (ML) models have been built to solve these issues. The problem even after the new technologies’ introduction is the lack of datasets, classifiers work best for one problem and serve the least for the other set of problems, decision algorithms are not framed effectively. To overcome these problems, proposed method uses an ensemble approach on classifiers to provide a better solution for feature selection parameters. Our model outperforms the accuracy and detection rates compared to the individual classifiers. Decision Tree (DT), Logistic Regression (LR), and Support Vector Machine (SVM) values are given to the Random Forest (RF) ensemble classifier. Experiments are performed using CICIDS 2017 dataset. The proposed approach has accuracy of 98%, recall 97%, precision 100%, and F-score 98% compared to the individual models.
更多查看译文
关键词
Ensemble classifiers, Intrusion detection system, Machine learning, Network traffic, Optimizer
AI 理解论文
溯源树
样例
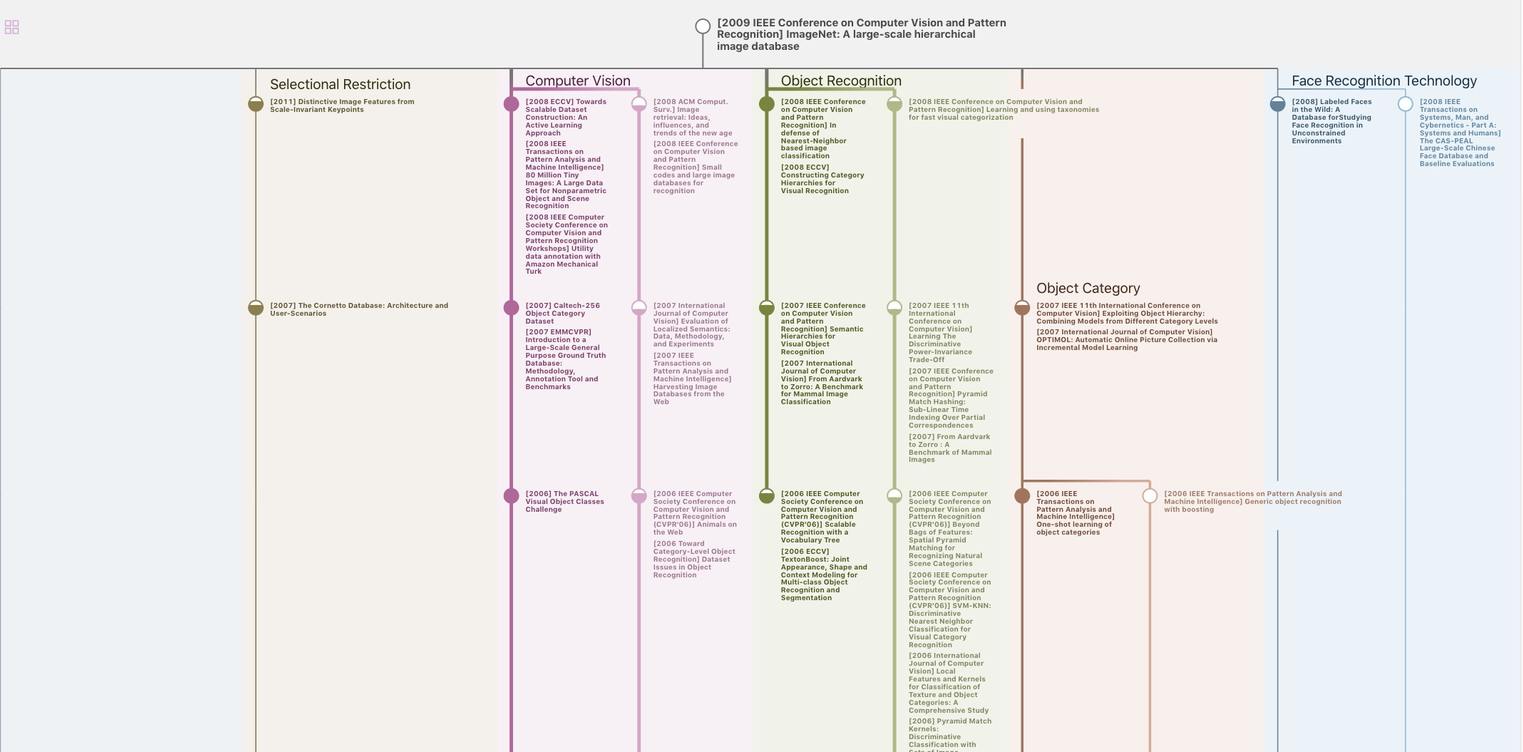
生成溯源树,研究论文发展脉络
Chat Paper
正在生成论文摘要