3D CNN-based Identification of Hyperdensities in Cranial Non-contrast CT After Thrombectomy
Bildverarbeitung für die Medizin 2022(2022)
摘要
To restore blood flow after an ischemic stroke due to large vessel occlusion, thrombectomy is a common treatment method. The postoperative noncontrast computed tomography (CT) often shows hyperdense regions which are due to hemorrhagic transformation or contrast staining. Since further treatment decisions depend on the presence of hyperdensities, their reliable detection is necessary. The Deep Learning based approach presented in this study can support radiologists in this task. The dataset consists of 241 postoperative volumetric noncontrast CTs. They are labeled by a binary classification regarding the presence or absence of a hyperdensity. A shallow 3D CNN architecture and a preprocessing pipeline were proposed. A part of the preprocessing is windowing the CTs to enhance contrast. Different windowing thresholds were defined based on knowledge regarding the CT values of brain tissue and hyperdensities. Using the proposed window with a level of 50 HU and a width of 60 HU, the network achieved an accuracy of 89% on the test data. Without windowing, an accuracy of only 46%was achieved. The present study demonstrates the importance of appropriate preprocessing and how domain knowledge can be included to optimize it. The results indicate that reducing the input information in a meaningful way accentuates relevant features in the images and enhances the network performance.
更多查看译文
关键词
hyperdensities,cnn-based,non-contrast
AI 理解论文
溯源树
样例
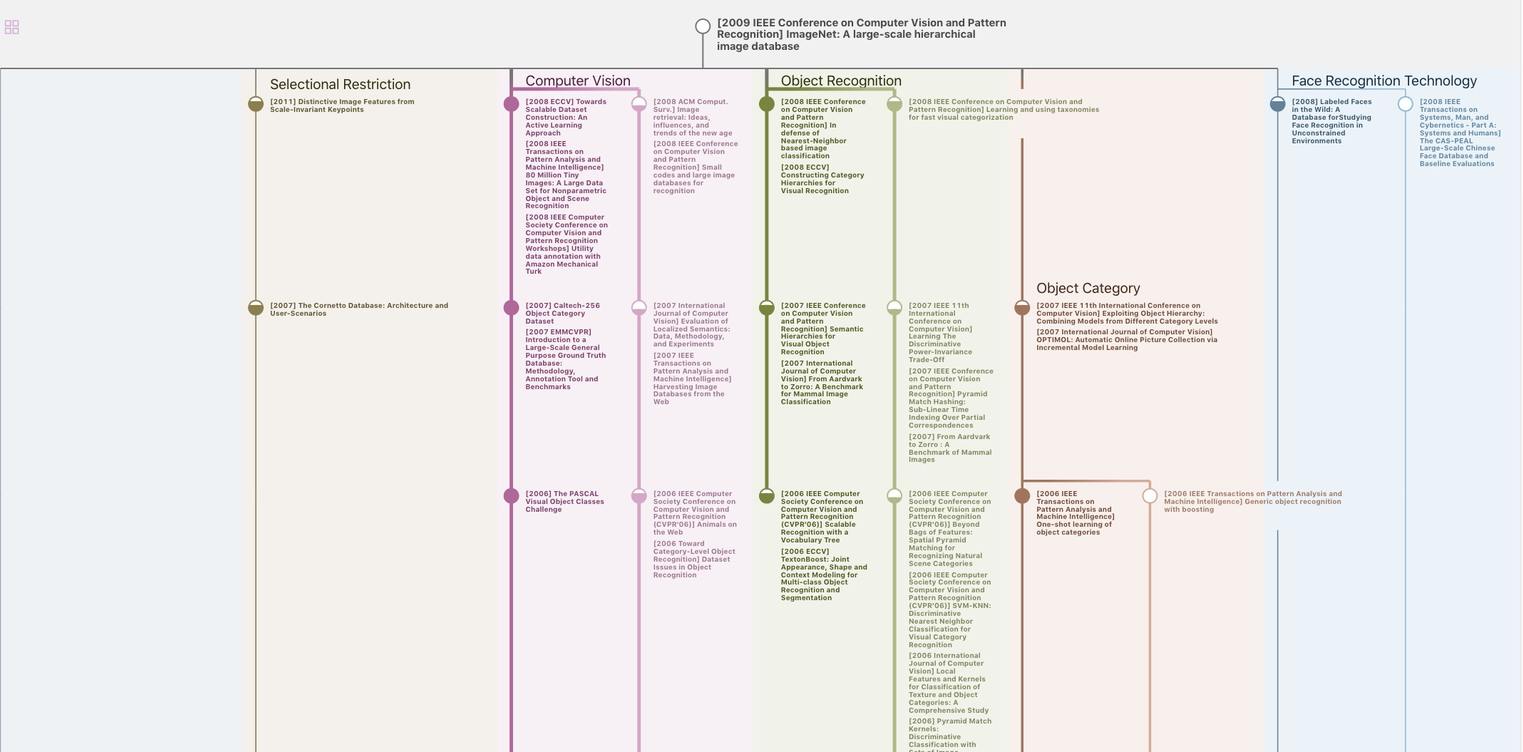
生成溯源树,研究论文发展脉络
Chat Paper
正在生成论文摘要