Identification of Vascular Cognitive Impairment in Adult Moyamoya Disease via Integrated Graph Convolutional Network
Medical Image Computing and Computer Assisted Intervention – MICCAI 2022(2022)
摘要
As one of the common complications, vascular cognitive impairment (VCI) comprises a range of cognitive disorders related to cerebral vessel diseases like moyamoya disease (MMD), and it is reversible by surgical revascularization in its early stage. However, diagnosis of VCI is time-consuming and less accurate if it solely relies on neuropsychological examination. Even if some existing research connected VCI with medical image, most of them were solely statistical methods with single modality. Therefore, we propose a graph-based framework to integrate both dual-modal imaging information (rs-fMRI and DTI) and non-imaging information to identify VCI in adult MMDs. Unlike some previous studies based on node-level classification, the proposed graph-level model can fully utilize imaging information and improve interpretability of results. Specifically, we firstly design two different graphs for each subject based on characteristics of different modalities and feed them to a dual-modal graph convolution network to extract complementary imaging features and select important brain biomarkers for each subject. Node-based normalization and constraint item are further devised to weakening influence of over-smoothing and natural difference caused by non-imaging information. Experiments on a real dataset not only achieve accuracy of
$$80.0\%$$
, but also highlight some salient brain regions related to VCI in adult MMDs, demonstrating the effectiveness and clinical interpretability of our proposed method.
更多查看译文
关键词
Vascular cognitive impairment, Moyamoya disease, Functional magnetic resonance imaging, Graph convolution network, Brain biomarkers
AI 理解论文
溯源树
样例
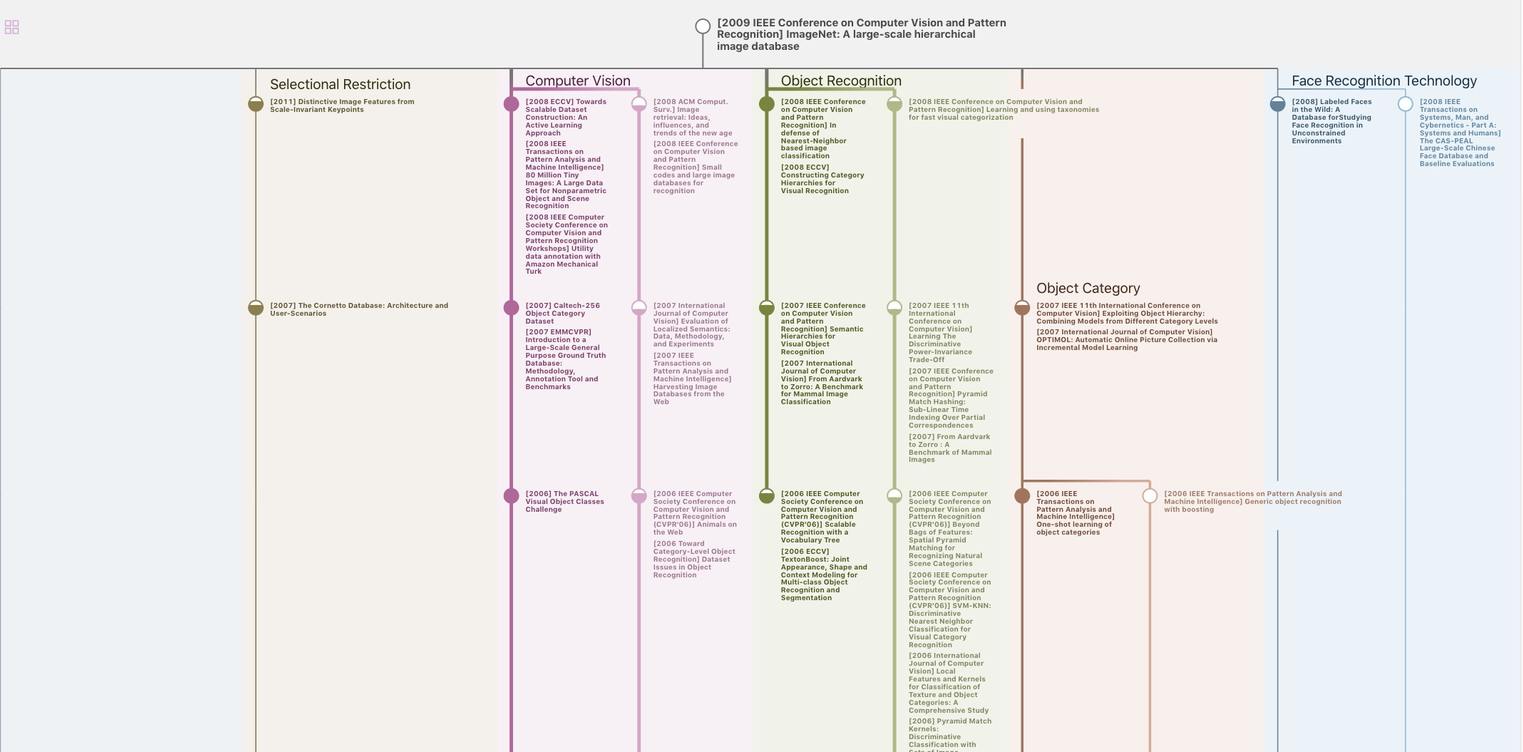
生成溯源树,研究论文发展脉络
Chat Paper
正在生成论文摘要