Towards Better Personalization: A Meta-Learning Approach for Federated Recommender Systems
Knowledge Science, Engineering and Management(2022)
摘要
Recommender systems (RSs) seek to suggest items that may be of interest to users by primarily modeling their historical interactions. In today's context, due to privacy and security constraints, it is becoming increasingly attractive to implement RSs based on federated learning frameworks, in which users' private data need not be uploaded to a central server. However, two major limitations remain unaddressed by existing works about federated RSs. First, canonical federated learning only develops a common output for all users and does not adapt the model to each user. This is an important missing feature, especially given the heterogeneity of the distribution of historical interaction data across users. Second, many current advanced recommendation algorithms, such as self-attention-based methods, have not been applied to federated RSs due to mobile devices' limited storage and computing power. To address these issues, we propose a personalized variant of the well-known Federated Averaging algorithm, where the Model-Agnostic Meta-Learning (MAML) framework is applied to provide a more personalized recommendation model for each user. Meanwhile, we investigate a sparse attention mechanism for sequential recommendation that significantly reduces the time complexity and memory usage when computing attention scores. Extensive experiments on six benchmark datasets reveal that our method consistently outperforms other federated RSs.
更多查看译文
关键词
Recommender system,Sequential recommendation,Attention mechanism,Federated learning,Meta-learning
AI 理解论文
溯源树
样例
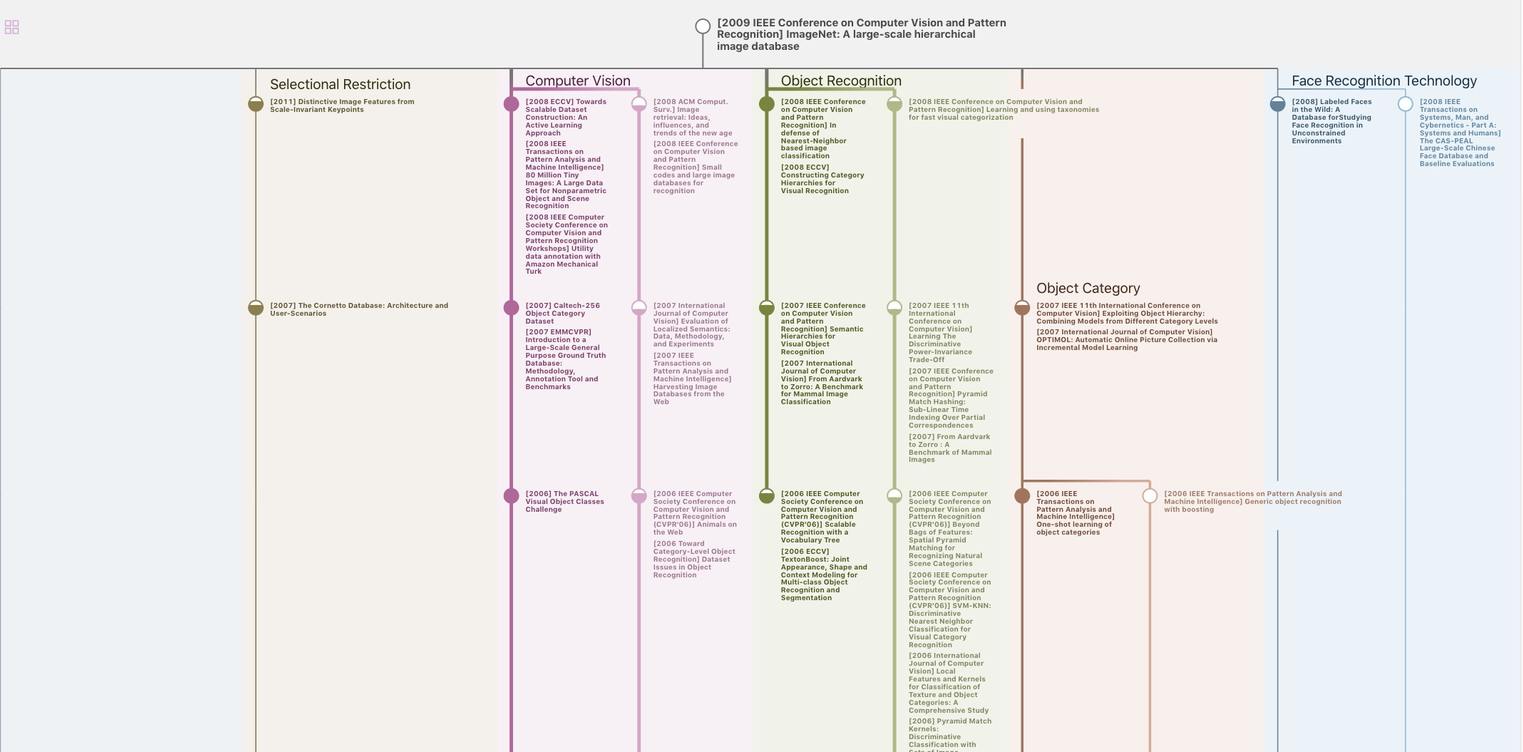
生成溯源树,研究论文发展脉络
Chat Paper
正在生成论文摘要