Towards Refined Classifications Driven by SHAP Explanations
Machine Learning and Knowledge Extraction(2022)
摘要
Machine Learning (ML) models are inherently approximate; as a result, the predictions of an ML model can be wrong. In applications where errors can jeopardize a company’s reputation, human experts often have to manually check the alarms raised by the ML models by hand, as wrong or delayed decisions can have a significant business impact. These experts often use interpretable ML tools for the verification of predictions. However, post-prediction verification is also costly. In this paper, we hypothesize that the outputs of interpretable ML tools, such as SHAP explanations, can be exploited by machine learning techniques to improve classifier performance. By doing so, the cost of the post-prediction analysis can be reduced. To confirm our intuition, we conduct several experiments where we use SHAP explanations directly as new features. In particular, by considering nine datasets, we first compare the performance of these “SHAP features” against traditional “base features” on binary classification tasks. Then, we add a second-step classifier relying on SHAP features, with the goal of reducing false-positive and false-negative results of typical classifiers. We show that SHAP explanations used as SHAP features can help to improve classification performance, especially for false-negative reduction.
更多查看译文
关键词
Interpretable machine learning, SHAP Explanations, Second-step classification
AI 理解论文
溯源树
样例
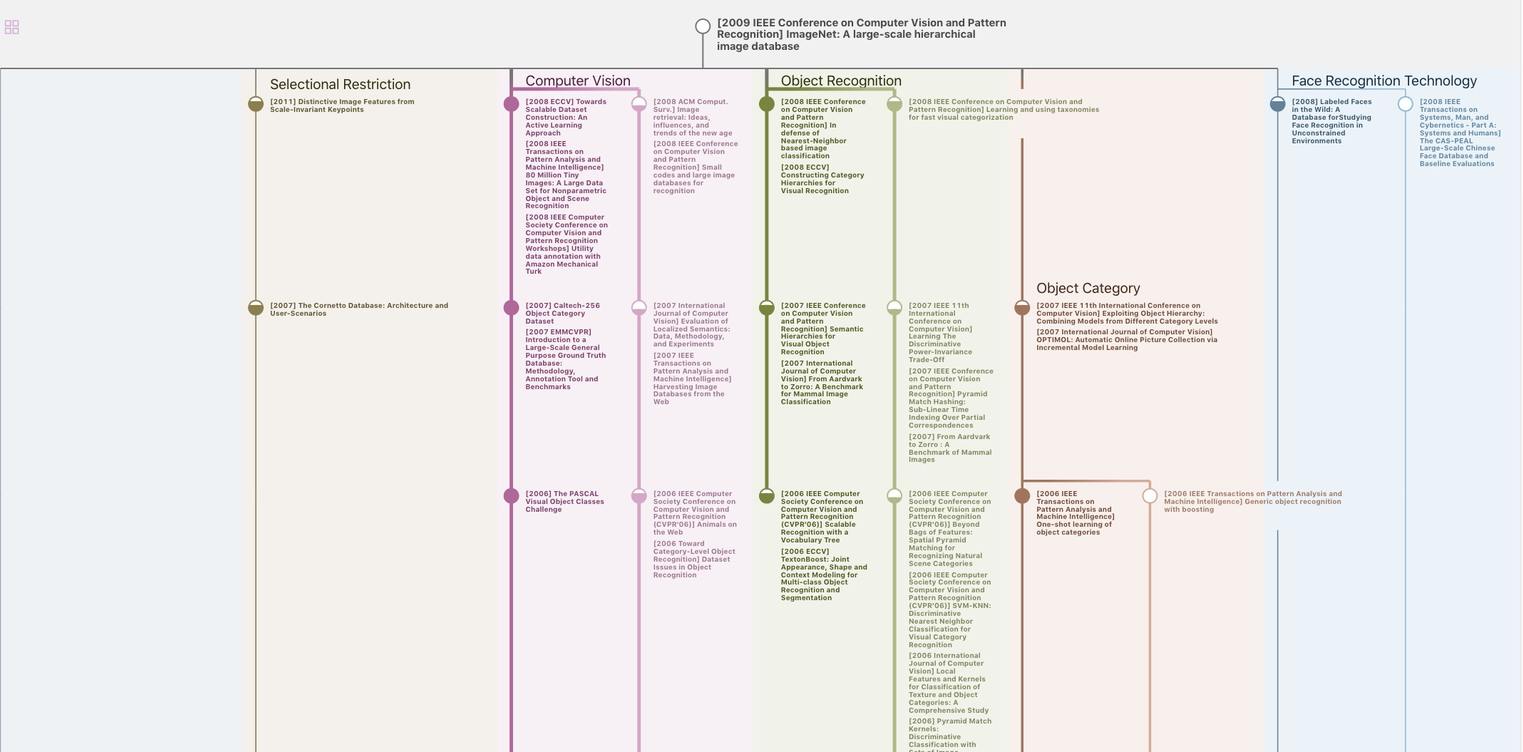
生成溯源树,研究论文发展脉络
Chat Paper
正在生成论文摘要