How to Compare Various Clustering Outcomes? Metrices to Investigate Breast Cancer Patient Subpopulations Based on Proteomic Profiles
Bioinformatics and Biomedical Engineering(2022)
摘要
Breast cancer is a highly diverse disease. With the state-of-the-art methods of molecular studies, novel subgroups of breast cancer can be revealed. The proper identification of subtypes is crucial for treatment choice. Hence, further investigation of breast cancer subtypes is promising in terms of therapy tailoring. We applied various machine learning approaches to the set of protein level measurements to detect subpopulations of breast cancer patients. Those methods involved various dimensionality reduction techniques combined with clustering. The outcomes of those approaches depended on the algorithms involved and on their parameters. Hence, we proposed the methodology to compare the results of clustering algorithms when the proper number of groups is unknown. The used metrices based on the effect size measurements and allowed for the selection of the best machine learning approach. The values of the proposed pooled d measure varied from 1.6847 for the worst method to 2.0568 for the best one. The highest value was obtained for the custom DiviK approach. Potentially, the metrices can also serve for the proteomic characterization of differences between subtypes and the identification of novel biomarkers.
更多查看译文
关键词
Breast cancer, Machine learning, Proteomics, Clustering, Dimensionality reduction
AI 理解论文
溯源树
样例
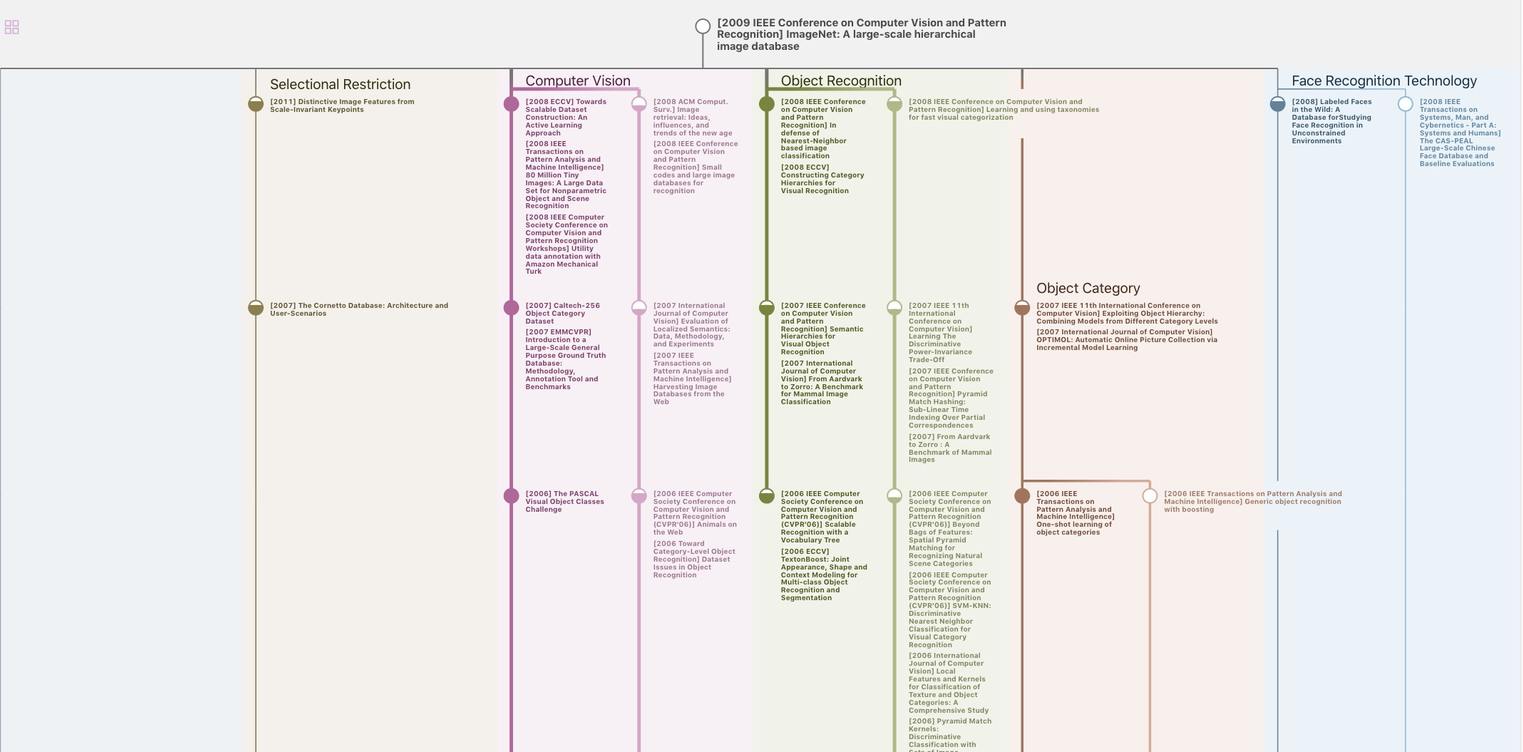
生成溯源树,研究论文发展脉络
Chat Paper
正在生成论文摘要