Multi-modal Universal Embedding Representations for Language Understanding
Frontiers in Cyber Security(2022)
摘要
In recent years, machine learning has made good progress in Computer Vision (CV), Natural Language Processing (NLP), and Vision + Language (V + L). However, most existing pre-training models just focus on single-modal (i.e., using only linguistic or visual features for training) or multi-modal (i.e., using both linguistic and visual features for training) scenarios, and can only use single-modal data or limited multi-modal data. This means that models for different scenarios need to be pre-trained separately, which requires a lot of computing resources and time. In this paper, we propose a universal method to train a general pre-training model to solve the problems in different scenarios and modalities. Moreover, we find that the model pre-trained with multi-modal data performs better in the single-modal downstream tasks. We use the General Language Understanding Evaluation (GLUE) benchmark for single-modal tasks to evaluate our model, which outperforms Bidirectional Encoder Representations from Transformers (BERT) in four tasks. For Vision + Language (V + L) tasks, we test our model on downstream tasks such as Visual Question Answering (VQA) and achieve similar performance to the current top-level model.
更多查看译文
关键词
Single-modality, Multi-modality, Pre-training, Fine-tuning, General learning method
AI 理解论文
溯源树
样例
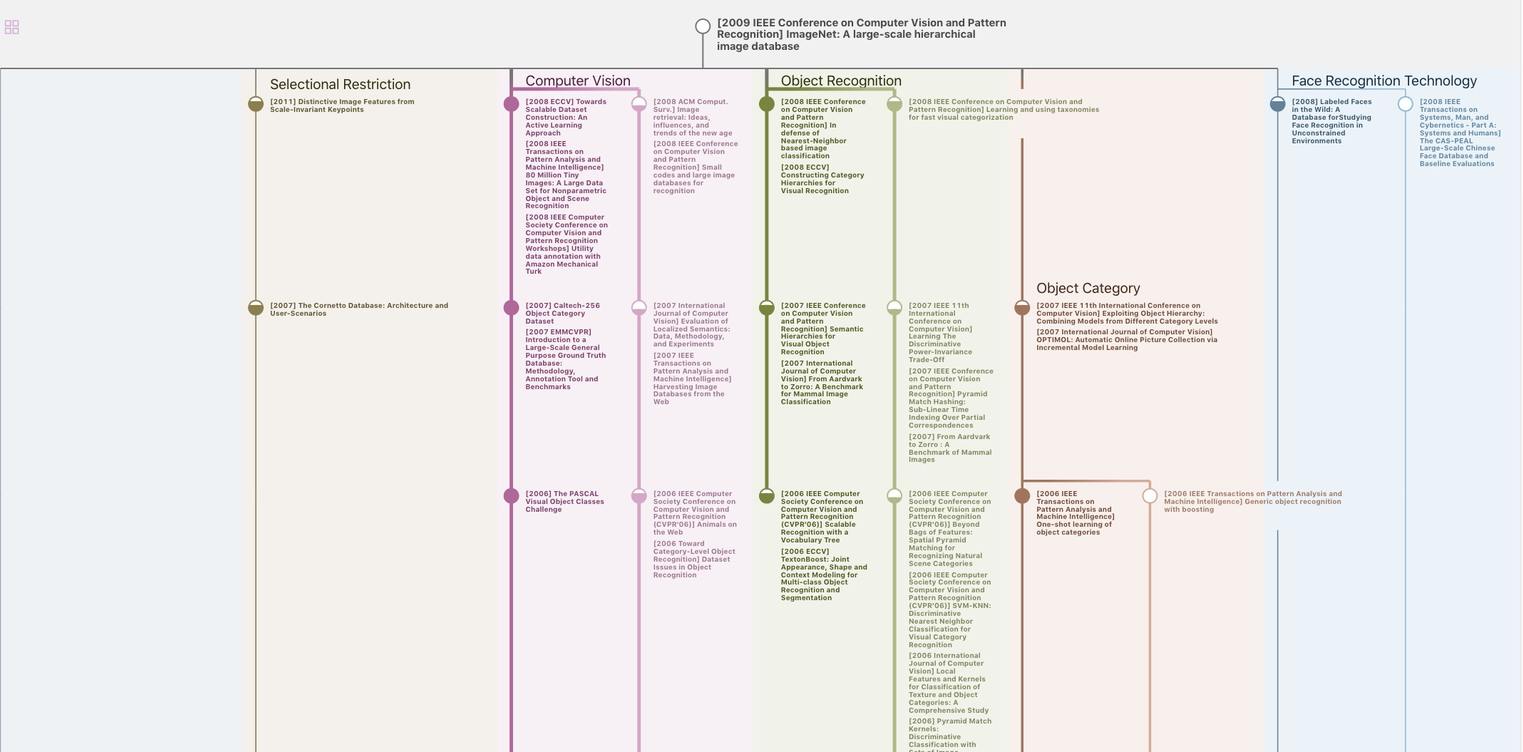
生成溯源树,研究论文发展脉络
Chat Paper
正在生成论文摘要