MR-LGC: A Mobile Application Recommendation Based on Light Graph Convolution Networks
Computer Supported Cooperative Work and Social Computing(2022)
Abstract
With the rapid growth of the number and types of mobile applications, how to recommend mobile applications to users accurately has become a new challenge. Graph convolution neural networks is a typical technique to facilitate mobile application recommendation. However, non-linear activation, feature transformation, and other operations in the existing mobile application recommendation based on graph convolution neural networks, which are used to model and characterize high-order interaction relationships between users and mobile applications, increase the difficulty of model training and lead to over-smoothing effects, reducing recommendation performance. To solve this problem, this paper proposes a mobile application recommendation method based on light graph convolution networks. In this method, firstly, a bipartite graph is used to model the interaction between users and mobile applications. Then, light graph convolution networks is utilized to smooth the features on the graph and extract the high-order connection between users and mobile applications, and three convolution layers are exploited to generate the feature representations of users and mobile applications. Finally, the inner product is used to predict the user’s preference for different mobile applications and complete the recommendation task. “Shopify app store”, a real dataset of Kaggle, is used to perform many groups of comparative experiments, and the experimental results show that the proposed method is superior to other methods.
MoreTranslated text
Key words
Mobile applications,Recommendation system,Light graph convolution networks,High-order connectivity
AI Read Science
Must-Reading Tree
Example
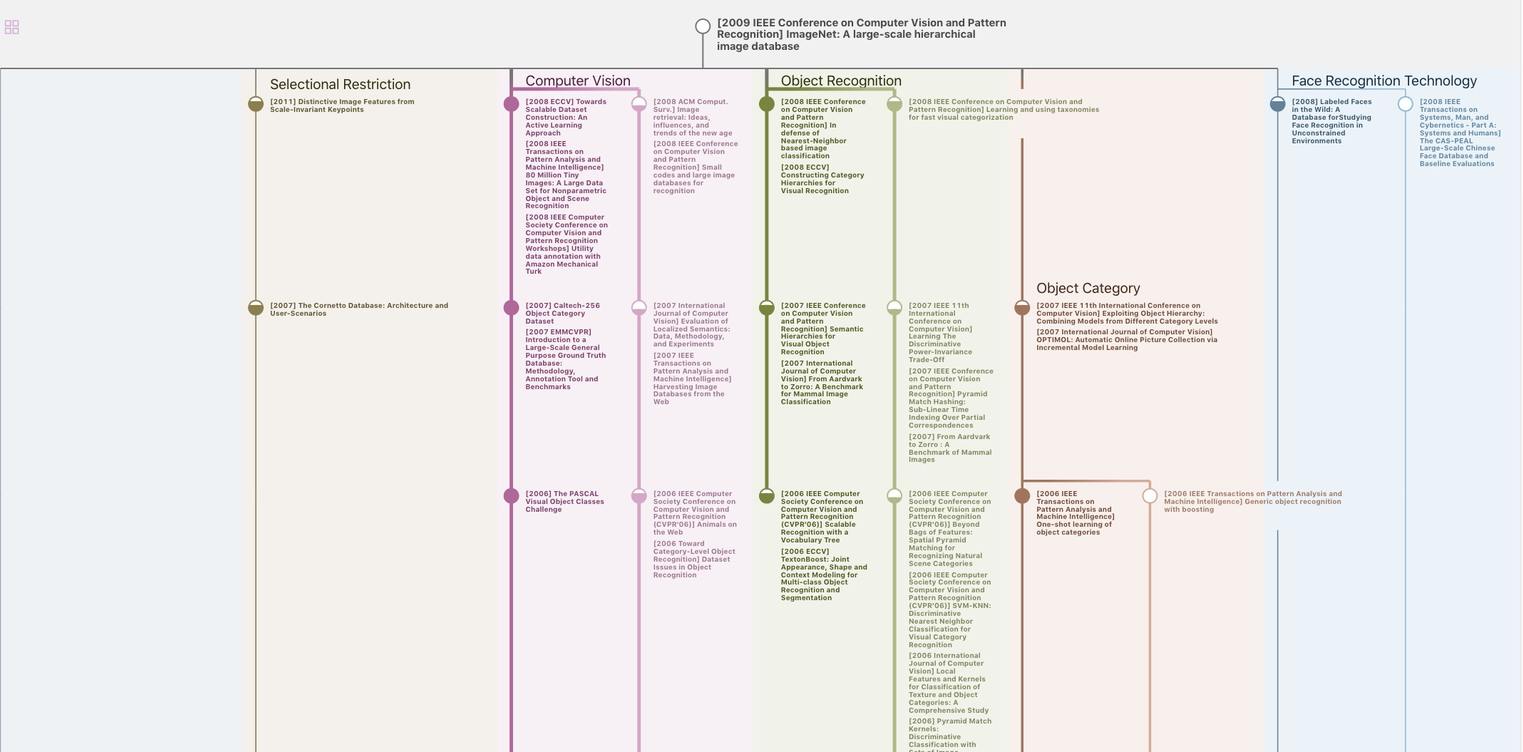
Generate MRT to find the research sequence of this paper
Chat Paper
Summary is being generated by the instructions you defined