Image-to-Video Translation Using a VAE-GAN with Refinement Network
Intelligent Computing Theories and Application(2022)
摘要
With the development of deep learning technology, various techniques for image processing have emerged in the field of computer vision in recent years, and have excellent performance in a variety of application scenarios. In contrast to the prediction task of predicting video with multiple consecutive frames before and after the input to predict the missing images in the middle, the task of image-to-video generation proposed in this paper does not require multiple consecutive frames, but rather the directional content generation of images by inputting the first frame image with the embedding vector of motion features, and to address some of the existing problems, this paper innovates the network architecture to solve the generated video problems such as incoherence, frame loss and blurring. For multiple image-to-video translation tasks, we propose a VAE-RGAN network with a further refinement network. We add a refinement network and use new identity matching loss and connected feature matching loss to eliminate VAE and GAN’s respective shortcomings and enhance the visual quality of the generated videos. Weizmann datasets have been the subject of a wide range of qualitative and quantitative experiments. We draw the following conclusions from this empirical study: (1) Compared with state-of-the-art approaches, our approach (VAE-RGAN) exhibits significant improvements in generative capability; (2) Experiments shows that our designed VAE-RGAN structure achieves better results and the refinement network significantly improves the problems of a blur.
更多查看译文
关键词
Video generation, Variational autoencoder, Generative Adversarial Network, Refinement network
AI 理解论文
溯源树
样例
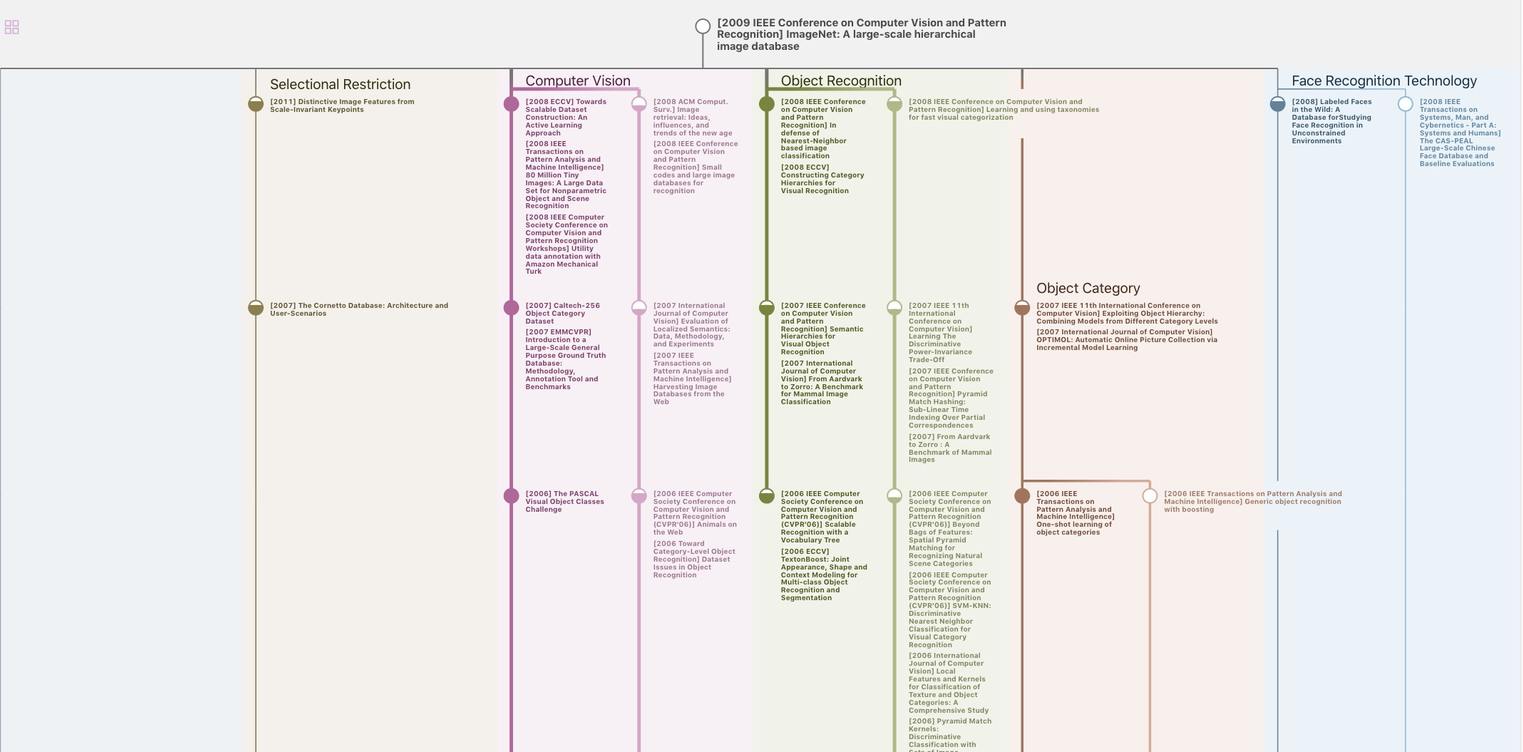
生成溯源树,研究论文发展脉络
Chat Paper
正在生成论文摘要