HNOP: Attack Traffic Detection Based on Hierarchical Node Hopping Features of Packets
Computational Science – ICCS 2022(2022)
摘要
Single packet attack, which is initiated by adding attack information to traffic packets, pose a great threat to cybersecurity. Existing detection methods for single packet attack just learn features directly from single packet but ignore the hierarchical relationship of packet resources, which trends to high false positive rate and poor generalization. In this paper, We conduct an extensive measurement study of the realistic traffic and find that the hierarchical relationship of resources is suitable for identifying single packet attacks. Therefore, we propose HNOP, a deep neural network model equipped with the hierarchical relationship, to detect single packet attacks from raw HTTP packets. Firstly, we construct resource node hopping structure based on the “Referer” field and the “URL” field in HTTP packets. Secondly, hopping features are extracted from the hopping structure of the resource nodes by G_BERT, which are further combined with the lexical features extracted by convolution operation from each node of the structure to form feature vectors. Finally, the extracted features are fed to a classifier, mapping the extracted features to the classification space through a fully connected network, to detect attack traffic. Experiments on the publicly available dataset CICIDS-2017 demonstrate the effectiveness of HNOP with an accuracy of 99.92% and a false positive rate of 0.12%. Furthermore, we perform extensive experiments on dataset IIE_HTTP collected from important service targets at different time. At last, it is verified that the HNOP has the least degraded performance and better generalization compared to the other models.
更多查看译文
关键词
Deep learning, Malicious traffic detection, Hopping features, Hierarchical relationship
AI 理解论文
溯源树
样例
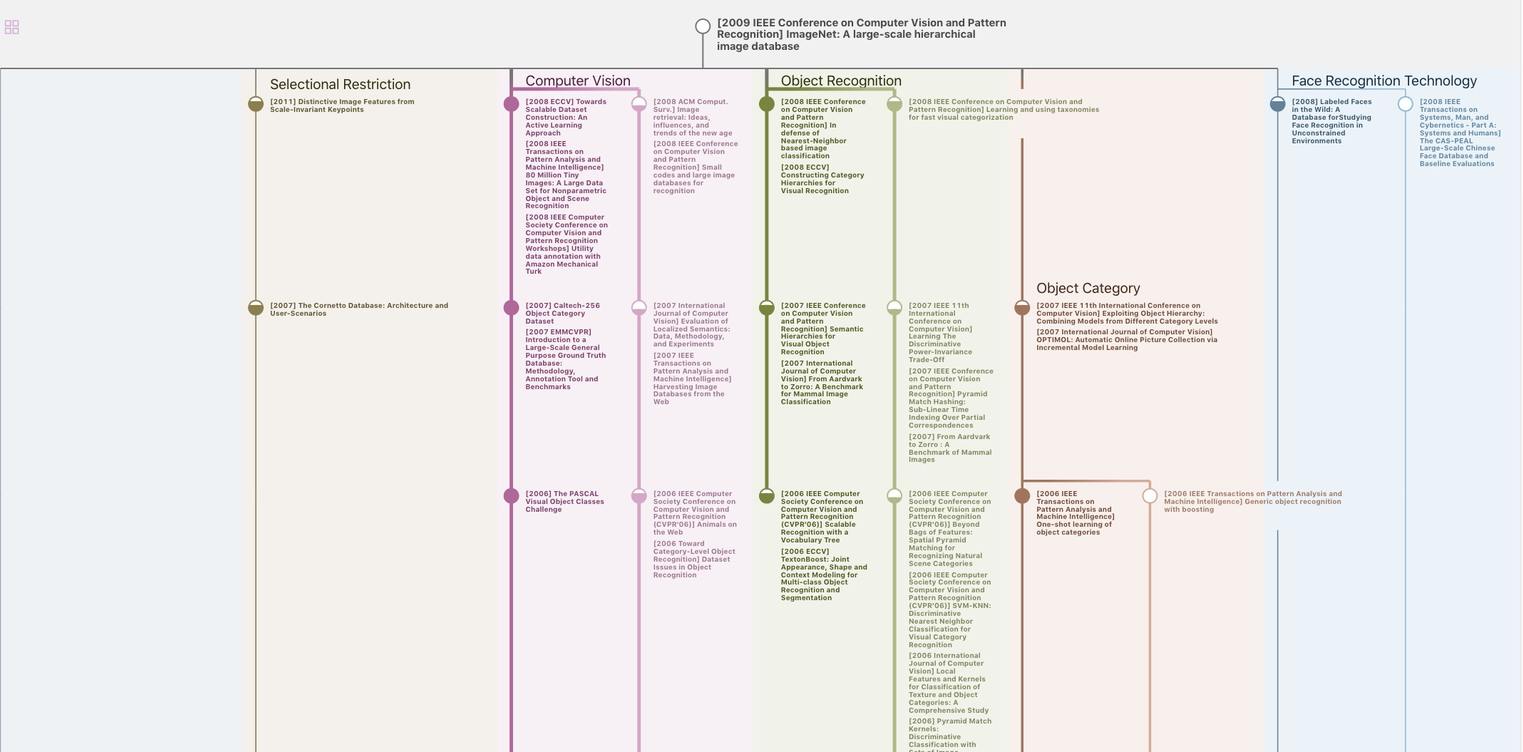
生成溯源树,研究论文发展脉络
Chat Paper
正在生成论文摘要