Asymmetry and Architectural Distortion Detection with Limited Mammography Data
Medical Image Learning with Limited and Noisy Data(2022)
摘要
Detection of the asymmetry (AS) and architectural distortion (AD) on mammograms is important for early breast cancer diagnosis. However, this is a challenging task because there are very limited mammography data containing these two lesions. In this paper, we tackle this problem by presenting a novel transfer learning framework of Supervised mass-Transferred Pre-training (STP) followed by Supervised Constrained Contrastive Fine-tuning (S
$$\mathrm C^2$$
F). While STP can leverage the commonly available mass data to help with detecting the rarely available AS and AD as pre-training, S
$$\mathrm C^2$$
F can depart the mass, AS, and AD in the embedding space as far as possible with a carefully designed constrained contrastive loss. In addition, a novel detection network - AsAdNet, is proposed for the AS and AD detection. The validation results on the largest-so-far AS and AD dataset show state-of-the-art (SOTA) detection performance.
更多查看译文
关键词
Mammogram, Asymmetry, Architectural distortion, Contrastive learning, Transfer learning, Detection
AI 理解论文
溯源树
样例
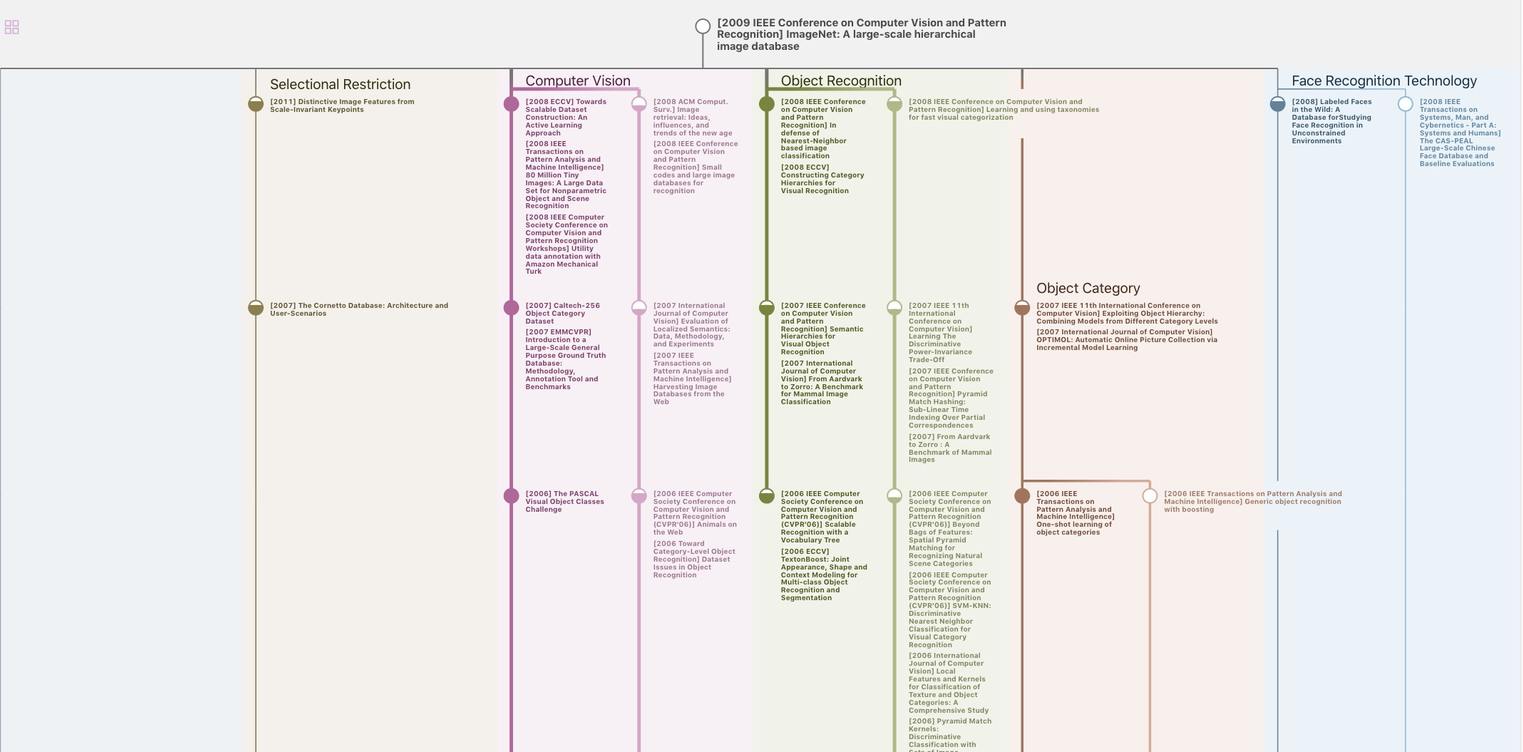
生成溯源树,研究论文发展脉络
Chat Paper
正在生成论文摘要