Assessing the Performance Gain on Retail Article Categorization at the Expense of Explainability and Resource Efficiency
KI 2022: Advances in Artificial Intelligence(2022)
摘要
Current state-of-the-art methods for text classification rely on large deep neural networks. For use cases such as product cataloging, their required computational resources and lack of explainability may be problematic: not every online shop can afford a vast IT infrastructure to guarantee low latency in their web applications and some of them require sensitive categories not to be confused. This motivates alternative methods that can perform close to the mentioned methods while being explainable and less resource-demanding. In this work, we evaluate an explainable framework consisting of a representation learning model for article descriptions and a similarity-based classifier. We contrast its results with those obtained by DistilBERT, a solid low-resource baseline for deep learning-based models, on two different retail article categorization datasets; and we finally discuss the suitability of the different presented models when they need to be deployed considering not only their classification performance but also their implied resource costs and explainability aspects.
更多查看译文
关键词
Multi-label text classification, Representation learning, Resource awareness, Explainability
AI 理解论文
溯源树
样例
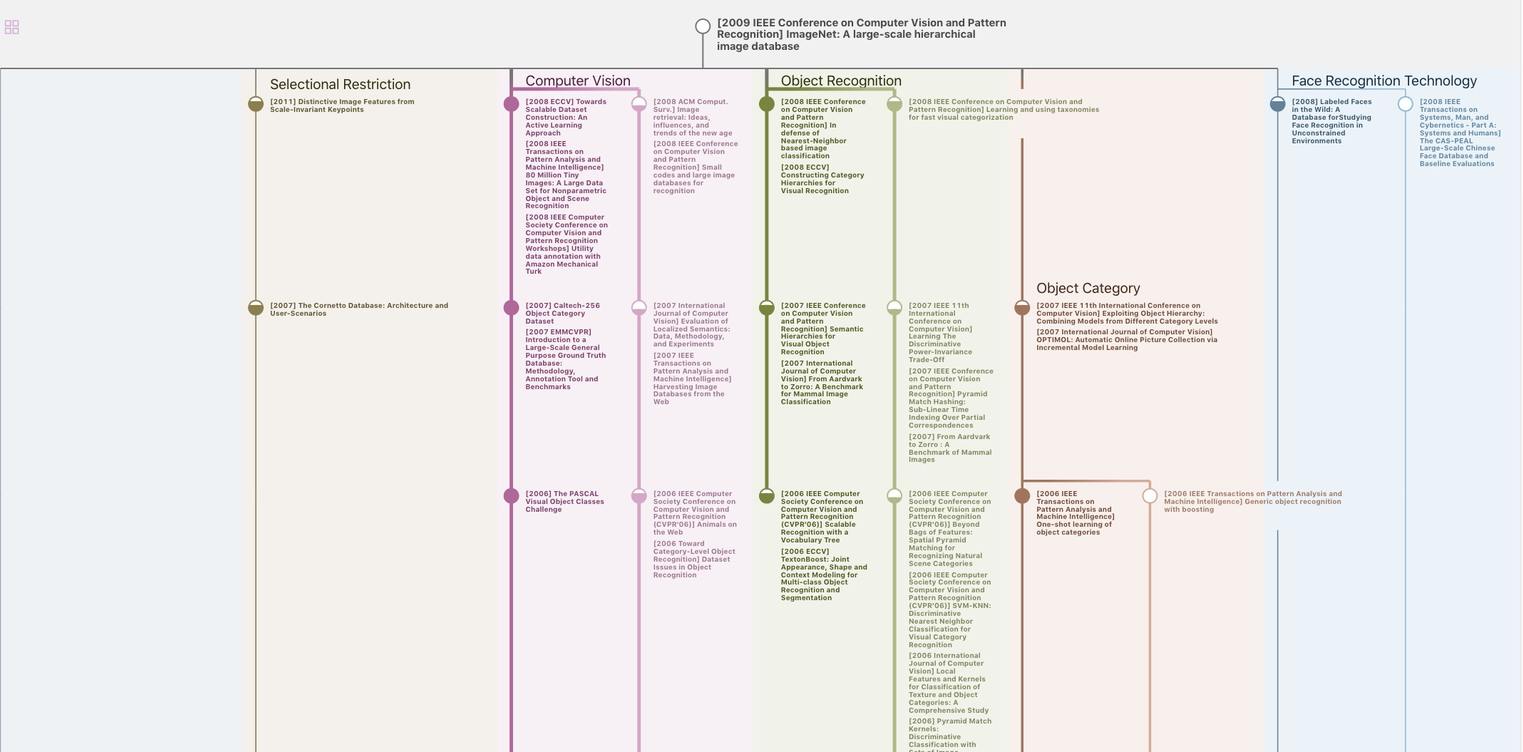
生成溯源树,研究论文发展脉络
Chat Paper
正在生成论文摘要