Modeling Information Diffusion With Sequential Interactive Hypergraphs
IEEE Transactions on Sustainable Computing(2022)
摘要
In online social networks, numerous users generate and distribute tremendous content simultaneously, and how information spreads in online society has attracted critical attention. Recent studies introduce deep learning to help mine the complex diffusion pattern and forecast the diffusion trend. However, there are two limitations of the state-of-art methods. On the one hand, existing models only focus on the internal influence within the diffusion flow but ignore the external influence from the dissemination of other contents. On the other hand, the dynamics of user interest are barely considered, while the changes of user preference for contents also have a considerable impact on future diffusion. To address these issues, we introduce the hypergraph structure and a sequential framework to model complex interactions in social networks. Then, we propose dual-channel hypergraph neural networks to tackle the diffusion prediction problem, denoted by HyperINF. Specifically, in the user channel, we build sequential user interactive hypergraphs and learn the dynamic user representation, and in the diffusion channel, we construct a diffusion interactive graph to capture the cross-diffusion relation. At last, we consider the social relation to help make the prediction. Experimental results on three datasets suggest the effectiveness and practicability of the proposed framework.
更多查看译文
关键词
Social networks,information diffusion prediction,hypergraph
AI 理解论文
溯源树
样例
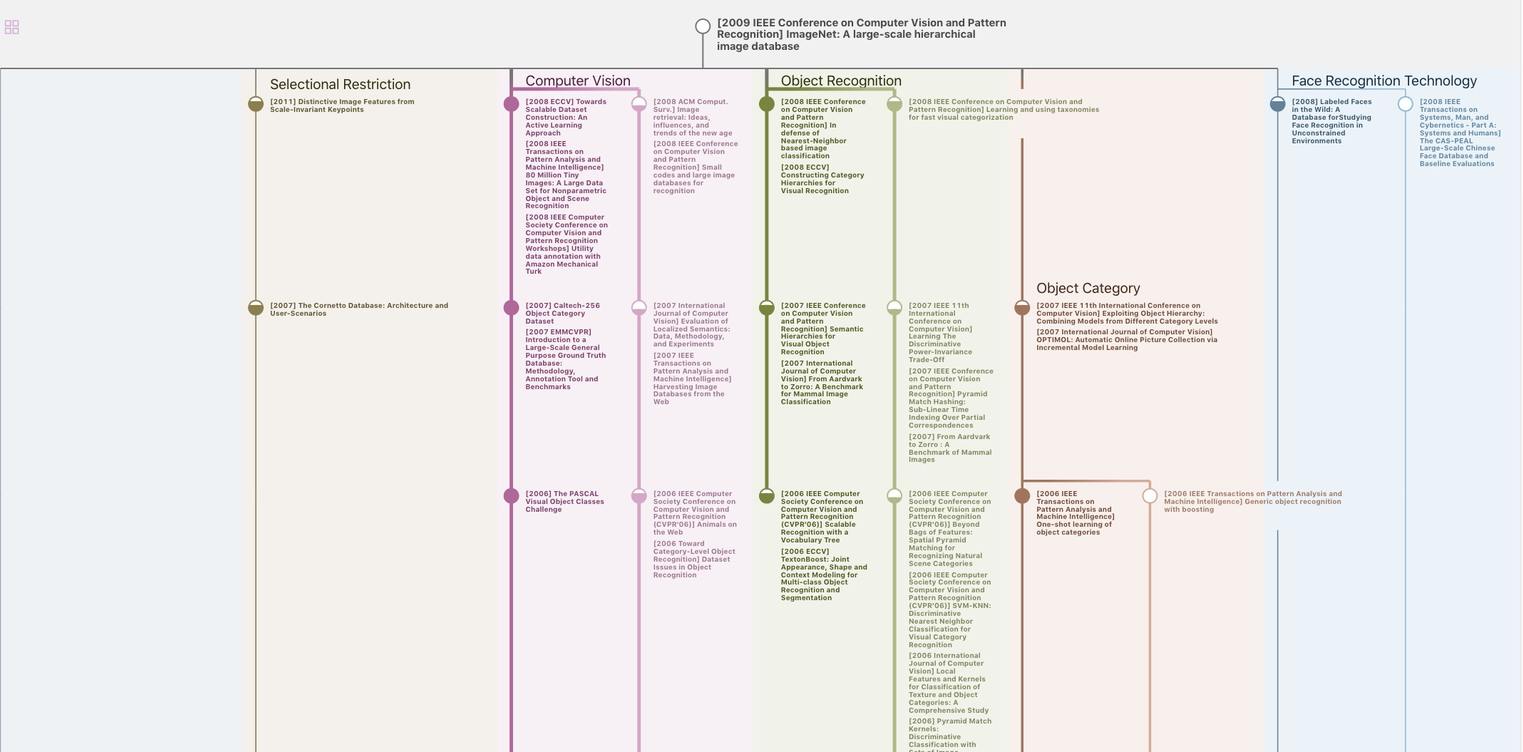
生成溯源树,研究论文发展脉络
Chat Paper
正在生成论文摘要