Multi-scale Interest Dynamic Hierarchical Transformer for sequential recommendation
Neural Computing and Applications(2022)
摘要
Existing sequential recommendation methods focus on modeling the temporal relationships of users’ historical behaviors and excel in exploiting users’ dynamic interests to improve recommendation performance. However, these methods rarely consider the existence of multi-scale user behavior sequences (e.g., temporal, location, and material scales), and sometimes user multi-scale interests play a decisive role in predicting final user preferences. To investigate the influence of multi-scale interests on user preferences, we study to develop a Multi-scale Interest Dynamic Hierarchical Transformer Model ( MIDHT ) to fine-grain modeling of users’ interests. Specifically, the proposal includes: First, the neighbor attention mechanism determines whether two neighboring items merge or not. Second, we generate the block mask matrix based on the above judgment results. Third, we compute the implicit representation of the current layer using the dynamic block mask matrix and the self-attention mechanism. Last, the dynamic block mask matrix of all layers to infer the corresponding hierarchical structure. Thorough experiments are implemented to show the features of MIDHT under different component settings. Furthermore, experimental results on three real-world datasets show that MIDHT significantly outperforms the state-of-the-art baselines on different evaluation metrics.
更多查看译文
关键词
Sequential recommendation,Multi-scale interest,Users’ behaviors,MIDHT
AI 理解论文
溯源树
样例
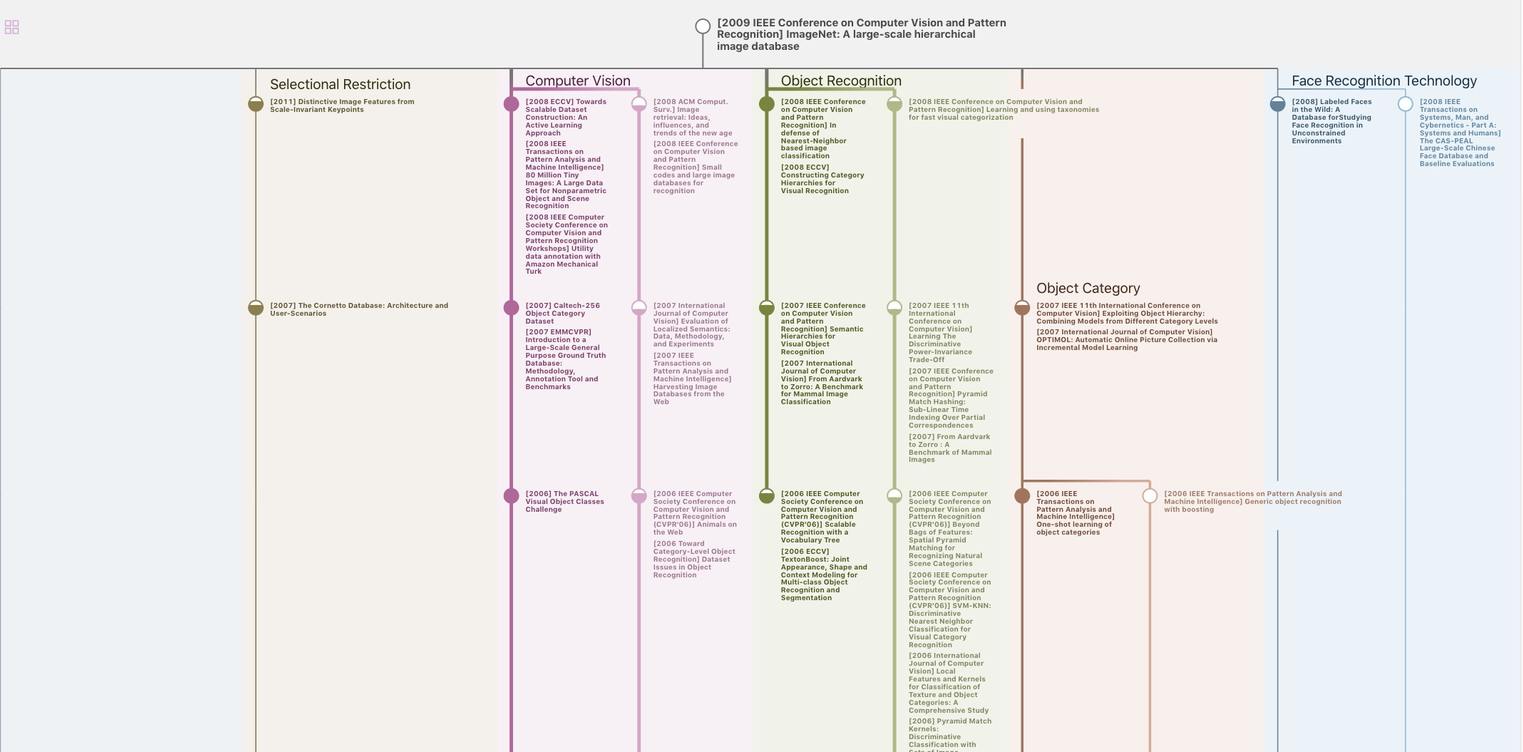
生成溯源树,研究论文发展脉络
Chat Paper
正在生成论文摘要