FlashMAC: A Time-Frequency Hybrid MAC Architecture With Variable Latency-Aware Scheduling for TinyML Systems
IEEE Journal of Solid-State Circuits(2022)
摘要
With the widespread of deep neural networks (DNNs) in diverse applications, tiny platforms such as Internet-of-Things devices are starting to adopt DNNs. Due to their extreme energy and form factor constraints, conventional digital-only implementations of multiply-and-accumulate (MAC) acceleration faced fundamental limitations. To that end, the investigation into mixed-signal computing architectures is growing rapidly. Motivated by the flash ADC, this article proposes FlashMAC architecture that can natively support multibit multiplication. In addition, through fusing time- and frequency-domain computing methods without power-hungry oscillators, it enables low latency accumulation with low power consumption. As a result, the proposed time-frequency hybrid architecture achieves high energy efficiency with the support for complex DNN models requiring higher precision. To enhance the robustness of PVT variation of the mixed-signal architecture, a frequency calibration loop is integrated. In addition, motivated by the data-dependent performance of the FlashMAC architecture, variable latency-aware scheduling is proposed. The FlashMAC does not skip MAC operations as zero-skipping architectures do, but the latency of the operation can be lower when operands are smaller in magnitude. Tackling the issue through software and hardware co-optimization, loose synchronization architecture and magnitude-aware weight reordering increase the DNN benchmark performance by achieving higher utilization of the parallel FlashMAC array. The proposed features are integrated into a test chip which is fabricated in 65-nm logic CMOS technology. The silicon chip achieves 56.52 TOPS/W peak energy efficiency and a peak operating frequency of 90 MHz. Tested with the VGG16 benchmark trained on the Imagenet dataset, it achieved 17.04-ms latency while showing 11.15 TOPS/W energy efficiency. As a result, compared to the previous state-of-the-art, the proposed FlashMAC achieved 3.15
$\times $
higher normalized energy efficiency.
更多查看译文
关键词
Analog computing,deep learning (DL),frequency-domain computing,mixed-signal multiply-and-accumulate (MAC),time-domain computing,TinyML
AI 理解论文
溯源树
样例
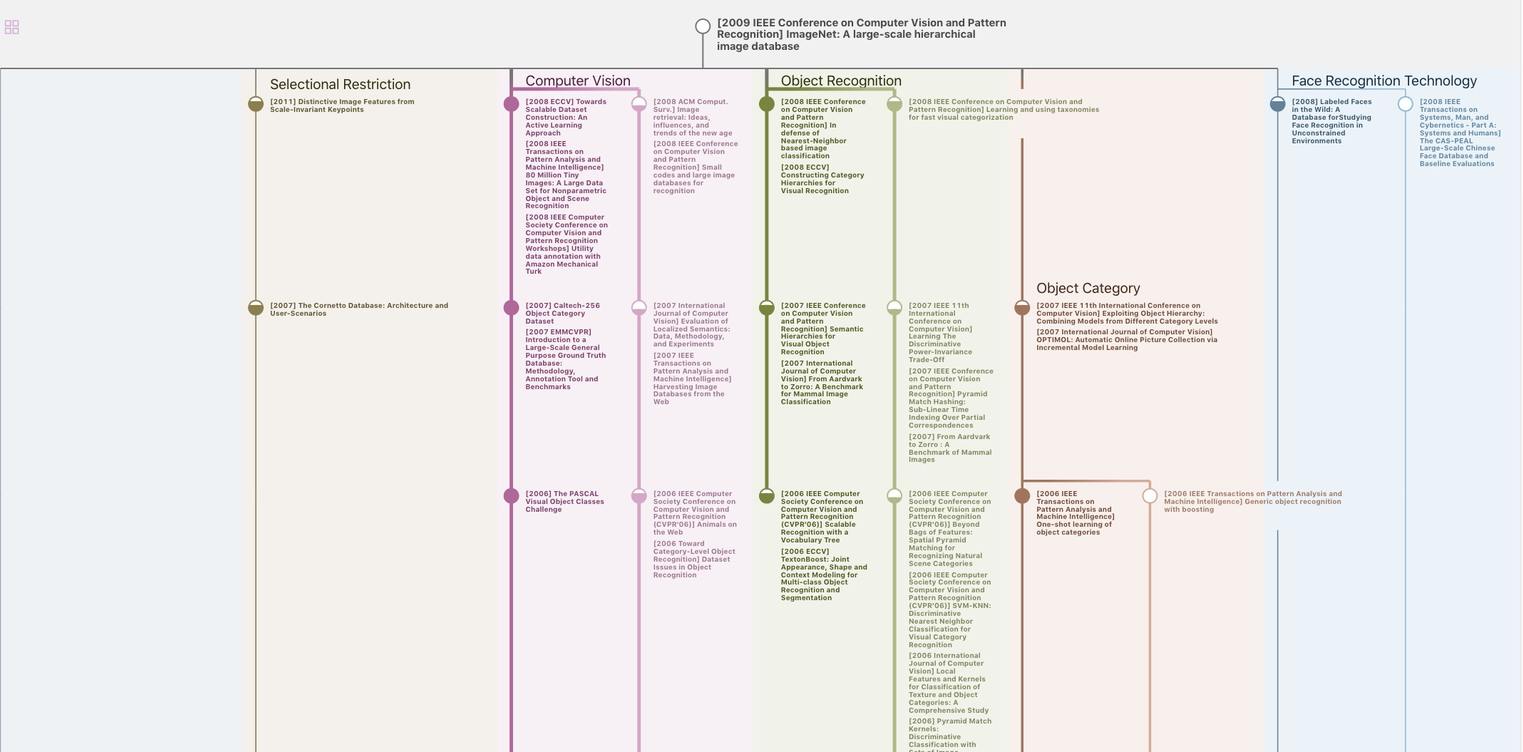
生成溯源树,研究论文发展脉络
Chat Paper
正在生成论文摘要