Efficient computation of expected motif frequency in uncertain graphs by exploiting possible world marginalization and motif transition
Social Network Analysis and Mining(2022)
摘要
Finding building blocks buried in real-world networks is an important task not only in network science but also in biology, chemistry, sociology, and other fields. Many attempts have been conducted to efficiently search for these building blocks under different settings. In this study, we take up the challenge of counting motifs from uncertain graphs, i.e., computing the expected frequency of motifs. In general, analysis for uncertain graphs is computationally expensive even with a small graph because there exists a vast number of possible worlds and a large number of sample graphs are needed to accurately cover the possible worlds. To alleviate such inefficiency coming from sampling which many existing studies rely on, we propose an analytical computation method that gives an exact expected frequency and is not based on costly sampling. The key idea of our method is to marginalize the probability of each possible world on a candidate motif, which can drastically reduce the number of possible worlds. We introduce matrices on the number of transition patterns and the transition probabilities among motifs to achieve further acceleration if the edge-existence probability is uniform and constant. We conduct experimental evaluations in the task of computing the frequency significance of directed 3- and 4-node and undirected 4-node motifs under both the uniform and non-uniform probability settings. The results confirm that the proposed method is effective and efficient. The sampling-based state-of-the-art method needs 2–4 orders of magnitude more time than the proposed method to achieve the same accuracy. In addition, the accelerated version of our method can achieve further acceleration, i.e., it runs about 1 order of magnitude faster than the general version of ours under the uniform probability setting.
更多查看译文
关键词
uncertain graphs,motif frequency,efficient computation
AI 理解论文
溯源树
样例
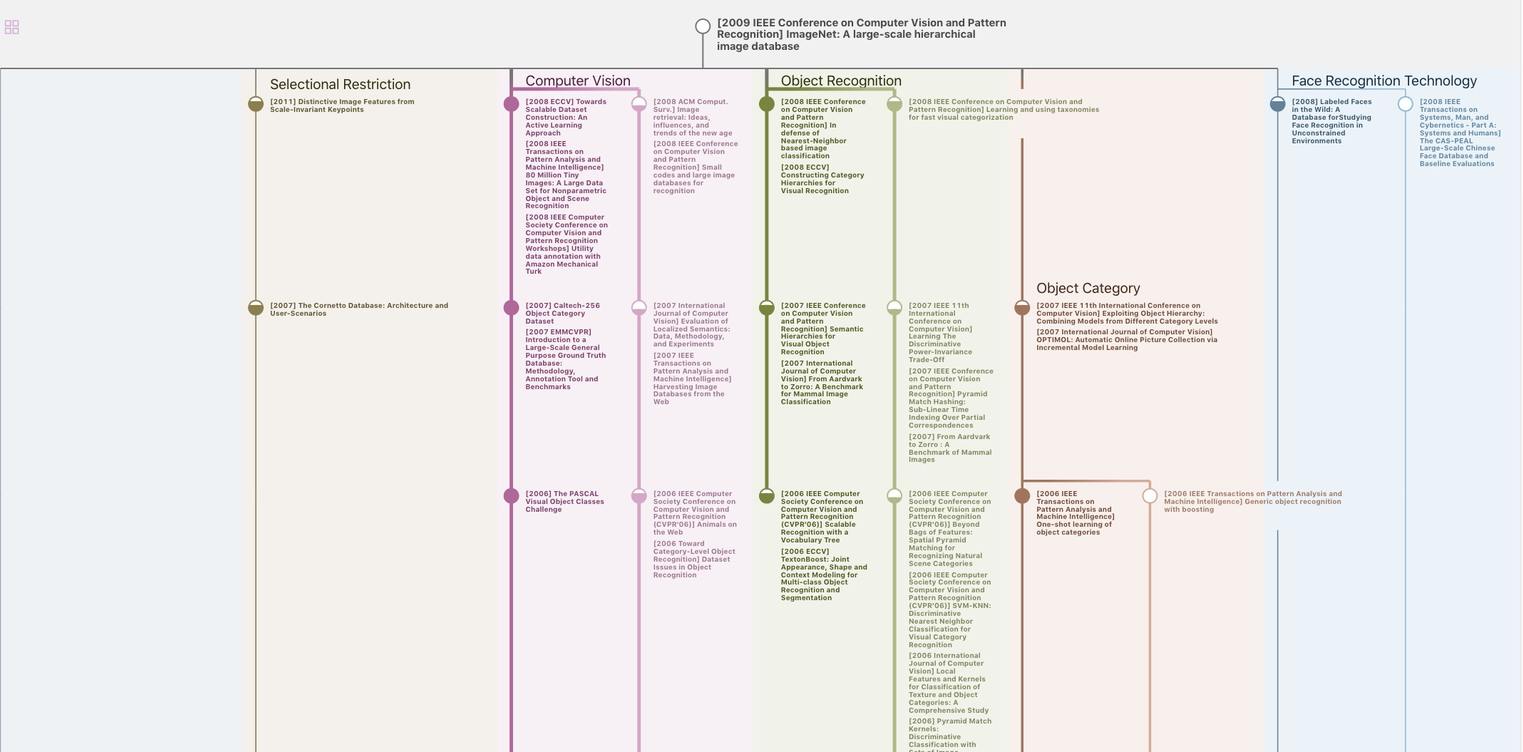
生成溯源树,研究论文发展脉络
Chat Paper
正在生成论文摘要