A Novel Sequence Tensor Recovery Algorithm for Quick and Accurate Anomaly Detection
IEEE Transactions on Network Science and Engineering(2022)
摘要
Anomalous traffic detection is a vital task in advanced Internet supervision and maintenance. To detect anomalies accurately, various data representations, such as vectors, matrices, and tensors, have been adopted to model traffic data. Among them, tensor-based methods outperform others due to their capability of capturing comprehensive correlations between complex network traffic. However, existing tensor-based algorithms remain certain shortcomings, such as working offline, cannot timely detect traffic anomalies, and high computation costs. To conquer the aforementioned deficiencies, we propose a novel
sequence tensor recovery
(STR) algorithm in this paper, which utilizes the results of historical tensor decomposition to achieve quick and accurate anomaly detection with low consumption when traffic data series arrive. Furthermore, we propose a
dynamic sequence tensor recovery
(DSTR) algorithm to improve anomaly detection accuracy by better capturing the variation over time of the comprehensive correlation of traffic data hidden in the tensor structure. The experimental results on two real traffic traces, Abilene and G
$\grave{E}$
ANT, indicate the proposed STR and DSTR algorithms are superior to the state-of-the-art algorithms in terms of accuracy and computation cost.
更多查看译文
关键词
Network security,Sequence traffic monitor,Online anomaly detection,Tensor recovery
AI 理解论文
溯源树
样例
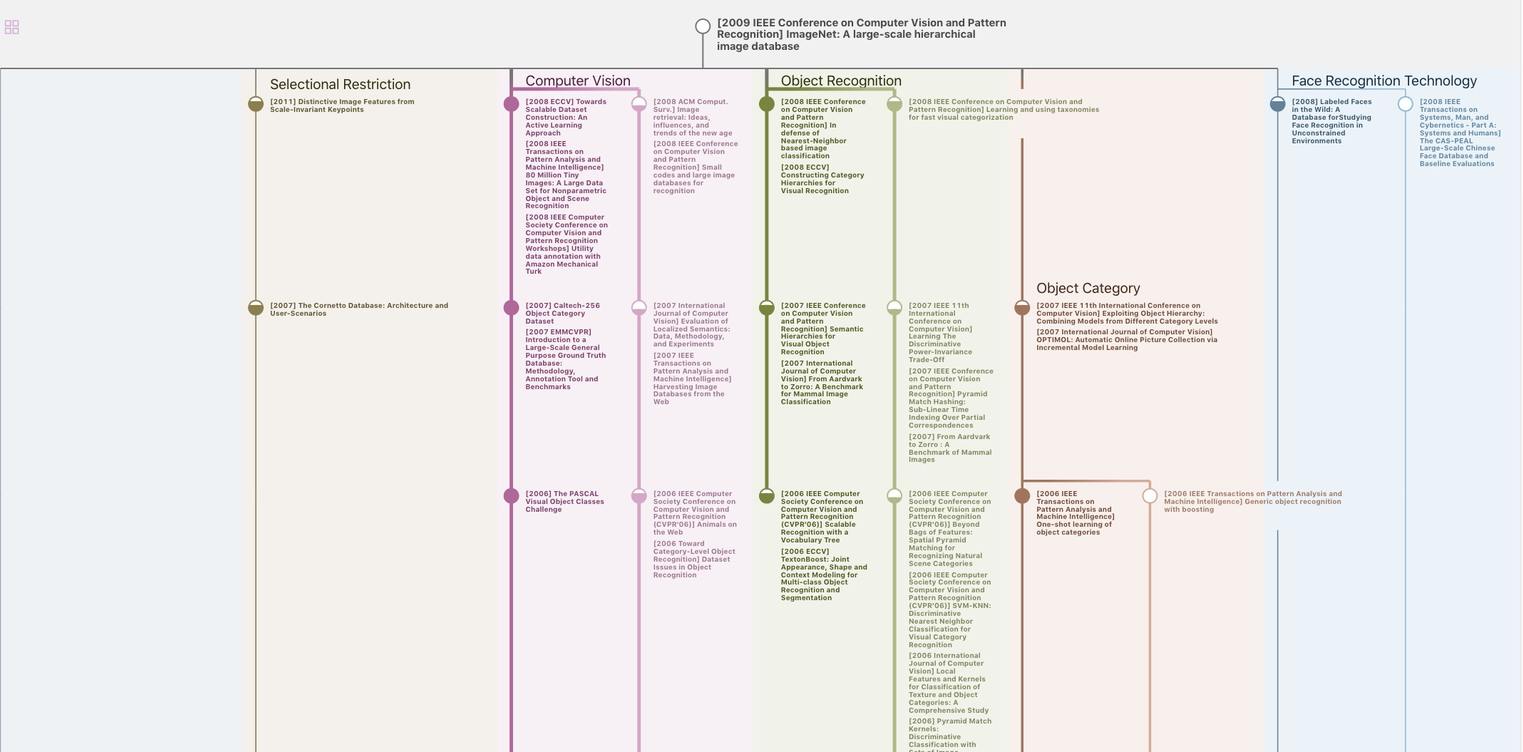
生成溯源树,研究论文发展脉络
Chat Paper
正在生成论文摘要