An improved traffic flow forecasting based control logic using parametrical doped learning and truncated dual flow optimization model
Wireless Networks(2022)
摘要
Traffic data-based object position forecasting is an exciting research area in feature recognition and analytic data systems. This recognition system is deployed in many applications to ensure forecasting, position identification, and prediction accuracy. Several methods of data processing techniques are available in the existing works, but it limits the issues like reduced accuracy, classification efficiency, and increased error rate. This paper intends to develop a new pattern extraction-based classification technique for traffic feature recognition to solve these problems. At first, the input test data is preprocessed by arranging the users with a proper cluster index for representing the data matrix with the signal pattern as in the data table, which is considered a feature matrix for the classifier. After that, the block separation is performed to extract the most valuable patterns from the optimized data by using Truncated Dual Flow Optimization (TDFO) using a Parametrical Doped Learning (PDL) based classifier to recognize the information on the extracted feature vectors. During experimental analysis, the performance of the proposed technique is validated by using various evaluation measures. Also, it is compared with some other existing methods for proving the superiority of the proposed pattern extraction-based classification system.
更多查看译文
关键词
Traffic geometrical features,User cluster,Parametrical Doped Learning (PDL) classifier,Location Forecasting and Truncated Dual Flow Optimization (TDFO)
AI 理解论文
溯源树
样例
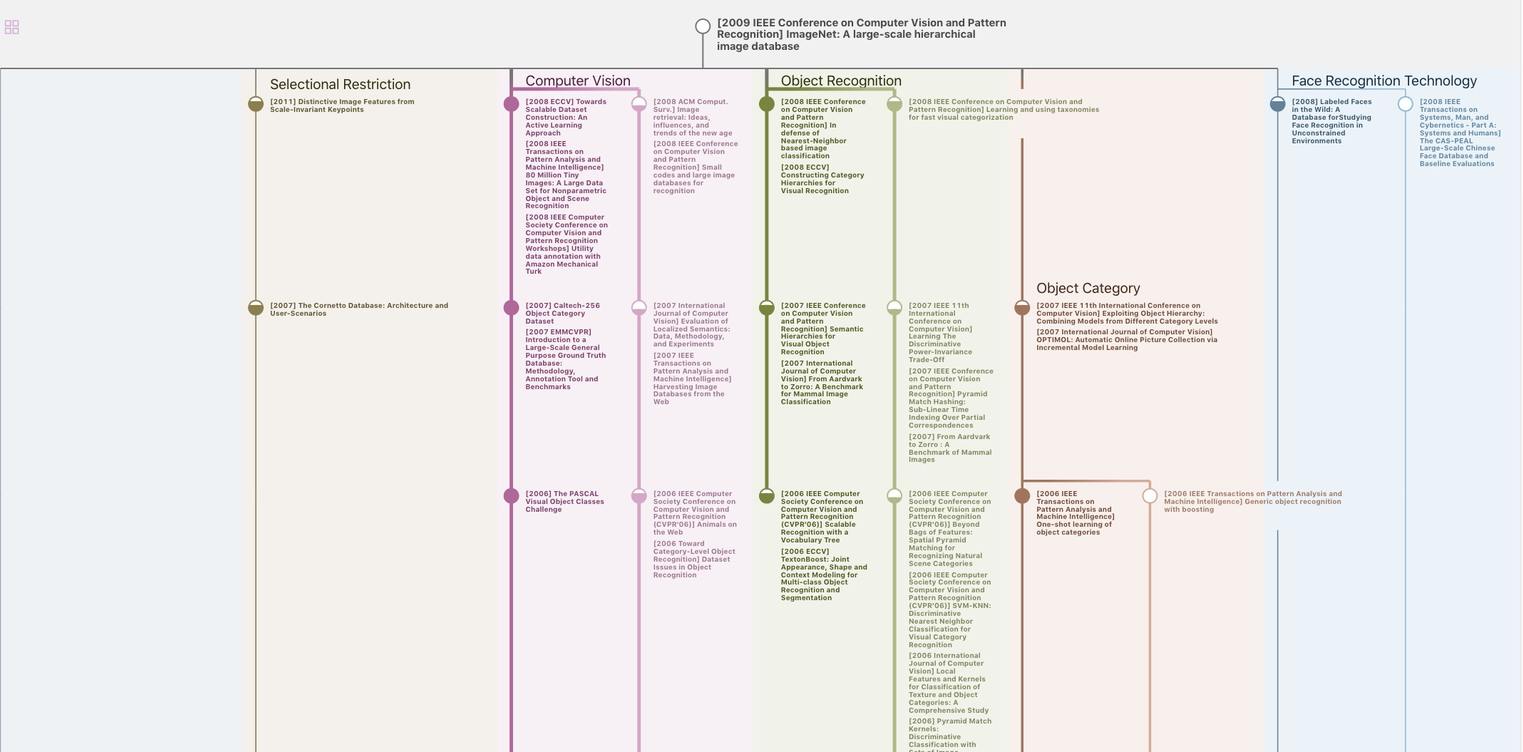
生成溯源树,研究论文发展脉络
Chat Paper
正在生成论文摘要