Hyperspectral image classification based on clustering dimensionality reduction and multi-scale feature fusion
Machine Vision and Applications(2022)
摘要
Hyperspectral images (HSI) contain rich ground object information, which has great potential in classification. However, the large amount of data and noise also pose a challenge to HSI classification. In this paper, a new framework based on band selection and multi-scale structure features is proposed, which mainly consists of the following steps. Firstly, the spectral dimension of the HSI is reduced with the clustering average method based on information divergence. Secondly, the detailed multi-scale structure features of HSI are extracted by using multi-parameter relative total variation. Thirdly, in order to reduce noise and highlight structural features, bilateral filtering is used to fine-tune the extracted structural features. Finally, the improved quantum particle swarm optimization algorithm is proposed to optimize the parameters of SVM. A lot of experiment results on two hyperspectral datasets show that the proposed method performs better than several state-of-the-art methods.
更多查看译文
关键词
Hyperspectral image,Dimensionality reduction reduction,Multi-scale structural features,Quantum particle swarm optimization,SVM
AI 理解论文
溯源树
样例
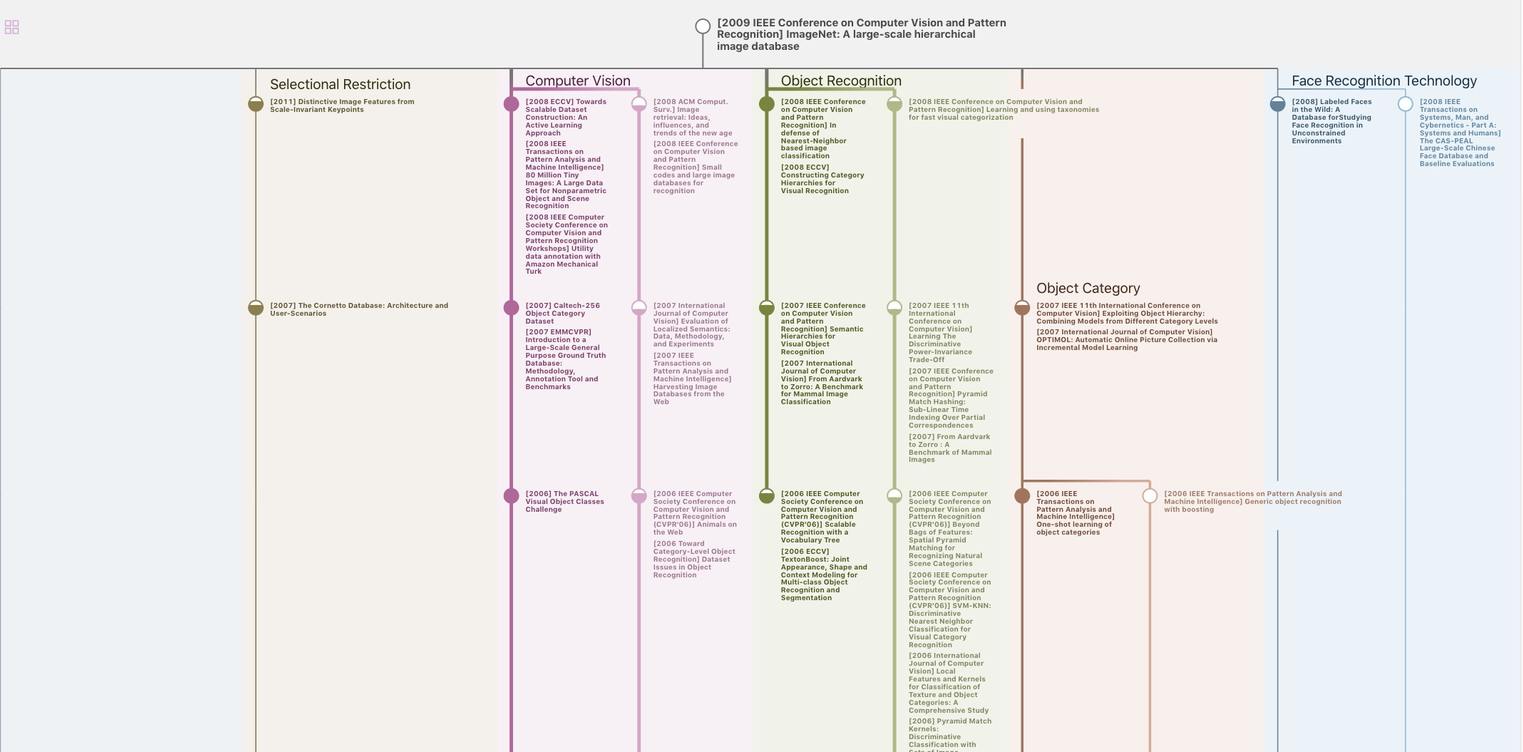
生成溯源树,研究论文发展脉络
Chat Paper
正在生成论文摘要