Exploiting Read Current Noise of TiOx Resistive Memory by Controlling Forming Conditions for Probabilistic Neural Network Hardware
IEEE Electron Device Letters(2022)
摘要
Conductance variations of resistive random-access memory (RRAM) are significant challenges that hinder the accurate inference of neural network (NN) hardware. In this study, we exploit the read noise of the RRAM as an active computational enabler for implementing probabilistic NN. As electrical characteristics of RRAM are directly related to the properties of conductive filament (CF), we statistically explore read current of TiO
x
-based RRAM with different forming conditions and explain the results by linking the CF model. In addition, an array mapping scheme to transfer weights to one transistor-one RRAM (1T1R) array is experimentally demonstrated. Through NN simulations, we verify that the probabilistic NN shows promising results on nonlinear classification problem avoiding overconfidence compared with deterministic NN.
更多查看译文
关键词
Bayesian neural networks,filamentary RRAM,neuromorphic,probabilistic computing,synaptic device
AI 理解论文
溯源树
样例
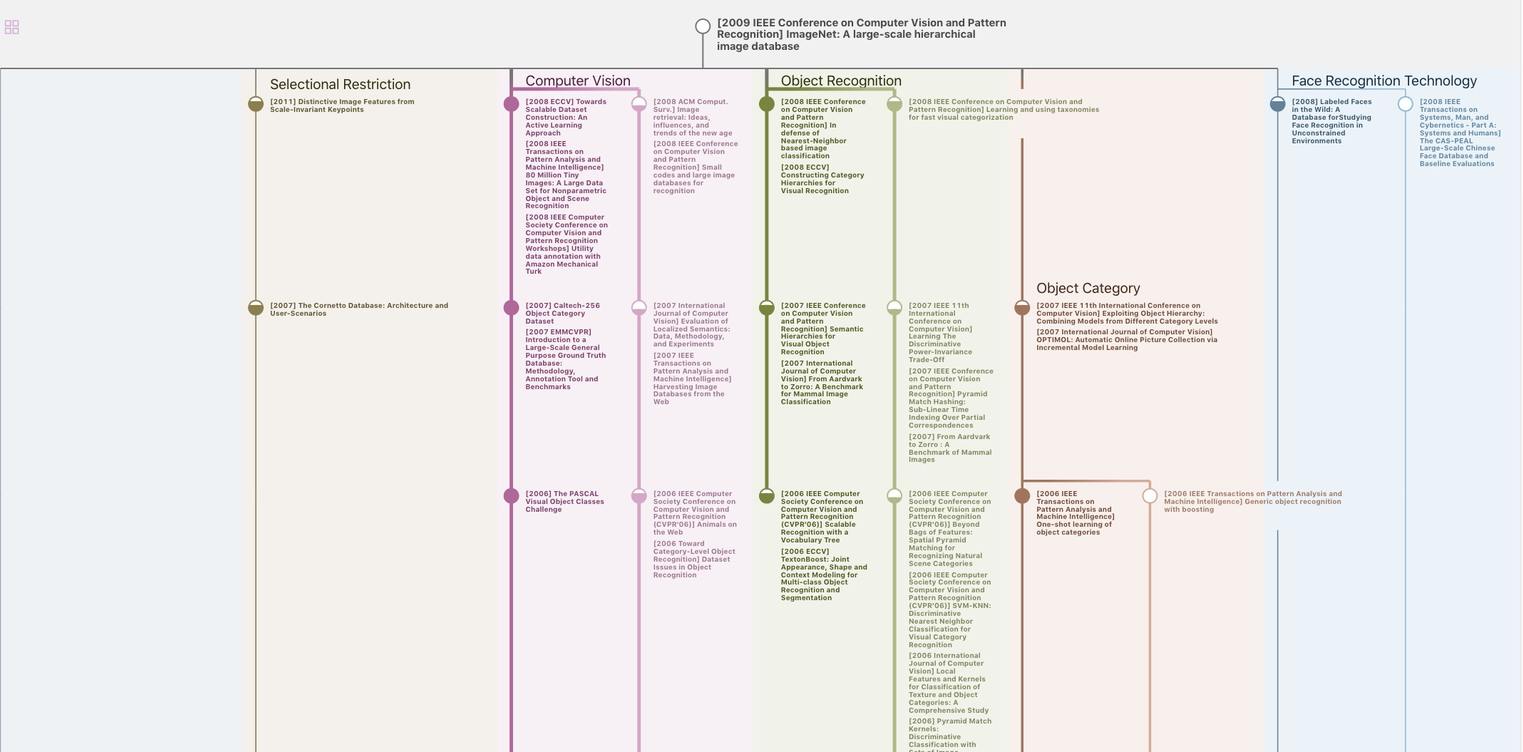
生成溯源树,研究论文发展脉络
Chat Paper
正在生成论文摘要