TR-ICANet: A Fast Unsupervised Deep-Learning-Based Scheme for Unconstrained Ear Recognition
Arabian Journal for Science and Engineering(2022)
摘要
Recently, several deep learning schemes have been considered for recognizing ear print. However, deep-based schemes are known to be data-hungry and they may require a significant deal of time to perform features learning. In this work, we propose a simple yet efficient and computationally fast CNN-like network, which we refer to as TR-ICANet, for recognizing ear print. We demonstrate that TR-ICANet can achieve higher performance than that of CNN with actually a lower computational budget and processing time. Firstly, we suggest applying a CNN-based image normalization to reshape images into a unified format, where CNN is used to detect ear landmarks and PCA is applied for geometrical normalization of scale and pose. TR-ICANet starts with an unsupervised learning process to learn convolutional filters using Independent Component Analysis (ICA). Those filters are used as feature detectors for the input images. This is followed by binary hashing and block-wise histogramming processes to generate local image features. Then, a Tied-Rank (TR) normalization is applied to eliminate bursts among the output features. We consider a soft-max average fusion scheme, in which CNN-like and deep networks are incorporated to improve recognition outcomes. Experiments conducted on the public AWE database show that TR-ICANet outperforms several relevant methods.
更多查看译文
关键词
Biometrics,Ear recognition,Deep-learning,ICA,AWE
AI 理解论文
溯源树
样例
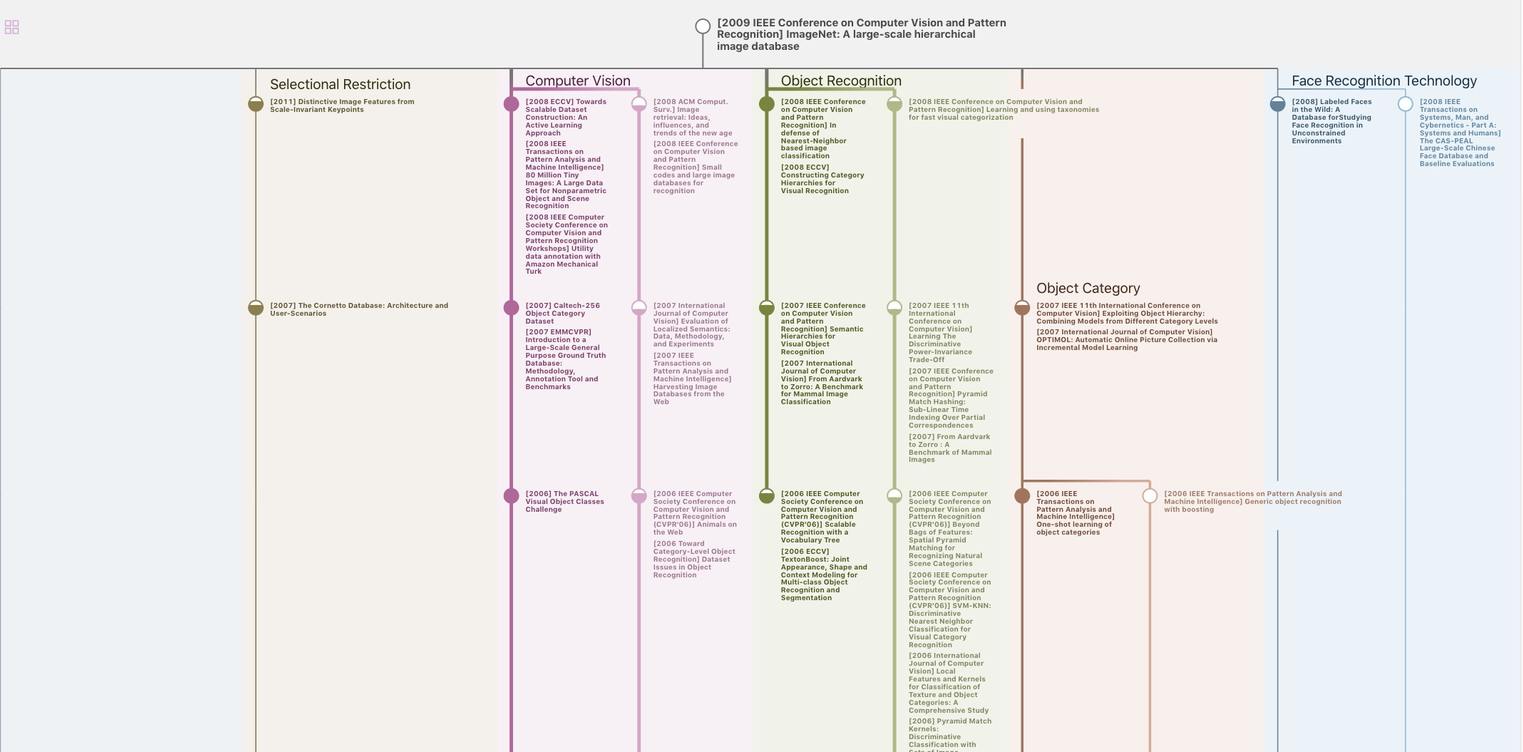
生成溯源树,研究论文发展脉络
Chat Paper
正在生成论文摘要