System reliability analysis of soil slopes using shear strength reduction and active-learning surrogate models
Arabian Journal of Geosciences(2022)
摘要
Although the shear strength reduction method (SRM) tends to give a more realistic prediction of a slope’s failure mechanism and of its associated factor of safety ( FS ) than the limit equilibrium method (LEM), it has had few applications in system reliability analyses of soil slopes due to its high computational cost. Active-learning surrogate model–based approaches provide a rational way to alleviate such computation burden through significantly reducing the required number of deterministic analyses (i.e., evaluation of FS ). However, such approaches have rare combinations with SRM for slope reliability analyses. In this paper, improved versions of three mainstream active-learning surrogate models (ASMs)—active-learning support vector machine (ASVM), active-learning radial basis function (ARBF), and active-learning Kriging (AK)—are employed to perform a comparative study considering their model efficiency and model stability, by which the ASM shown optimal combinatorial properties with SRM, can be singled out. During the reliability analyses, Monte Carlo simulation (MCS) is employed with the ASMs to estimate the system probability of failure ( P f,s ) of soil slopes. Three soil slopes taken from the literature are employed to assess the performance of the suggested procedures. Results show that the introduced ASMs provide good estimates of P f,s for soil slopes with different numbers of layers and of random variables, and that they often outperform other alternative methods, particularly for layered soil slopes. Among the ASMs considered, the ARBF shows the best performance in both computational efficiency and model stability.
更多查看译文
关键词
Layered soil slope, System reliability, Shear strength reduction method, Active-learning surrogate model
AI 理解论文
溯源树
样例
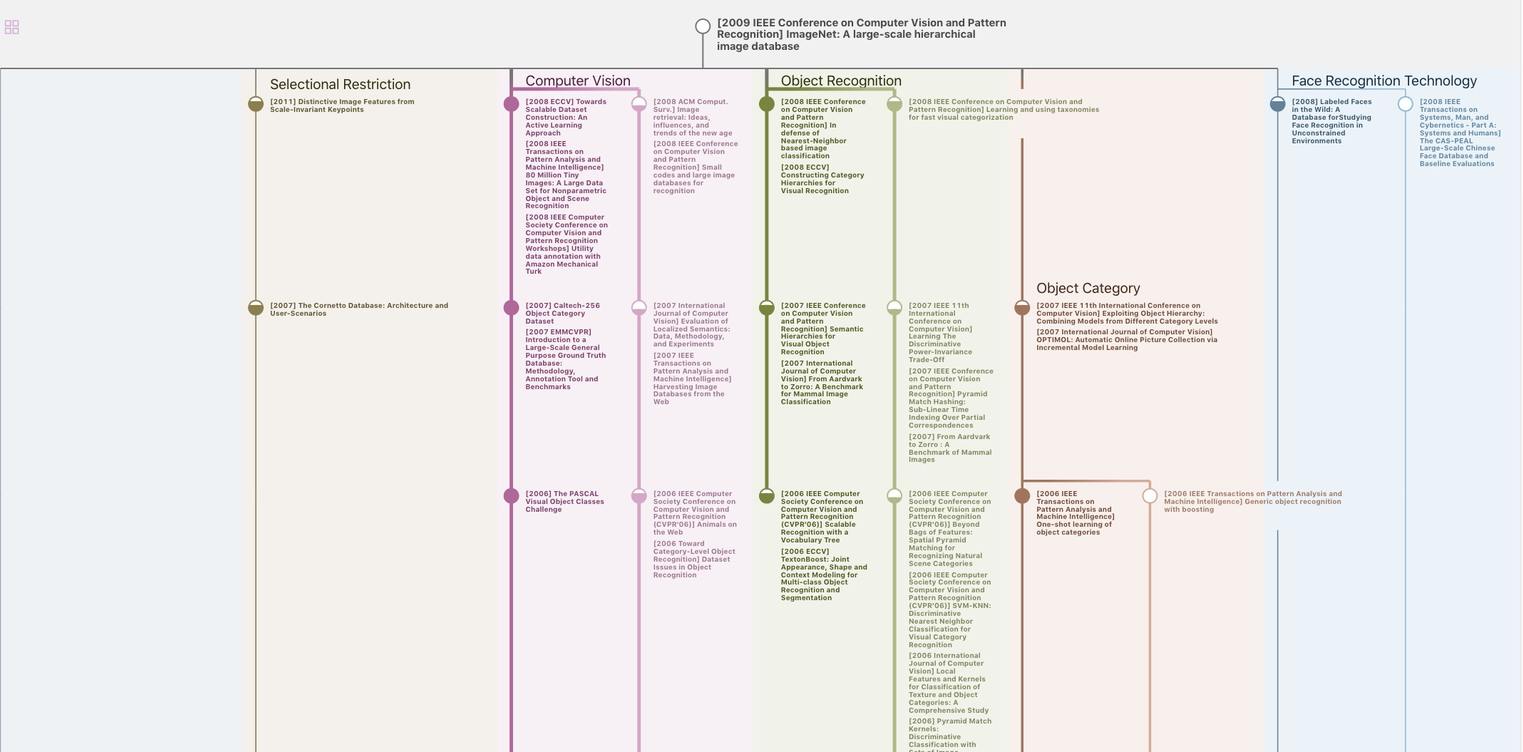
生成溯源树,研究论文发展脉络
Chat Paper
正在生成论文摘要