Inferring context with reliable collaborators: a novel similarity estimation method for recommender systems
Applied Intelligence(2022)
摘要
Additional context information is vital for context-aware recommender systems. The whole paradigm of context-aware recommender systems is built upon the availability of contextual features. Apart from the significance of context, we highlight a key issue for existing context-aware recommendation paradigm that if the user environment did not provide contextual features such as time, location, or companion due to privacy constraints or if the data collection system is unable to record contextual attributes due to legal or technical concerns then the existing context-aware recommendation paradigm has no uniform mechanism to deal with this situation. In this research, we address these challenges and propose a novel item-context similarity (ICS) model capable of adaptively generating reliable collaborators for a subject user on a subject item. Additionally, ICS is fused into a weighting model called contextually reliable collaborators (CRC) that considers the current item context, the nonlinear relationship between candidate collaborators and the asymmetry between rating preferences of users to finally generate rating prediction. Experiments show that neighbors computed through ICS are more reliable than the classical similarity estimation methods and the ICS-based CRC model has outperformed state-of-the-art approaches.
更多查看译文
关键词
Context-aware recommender systems, Collaborative filtering, Similarity estimation, Reliable recommendation, Privacy protection
AI 理解论文
溯源树
样例
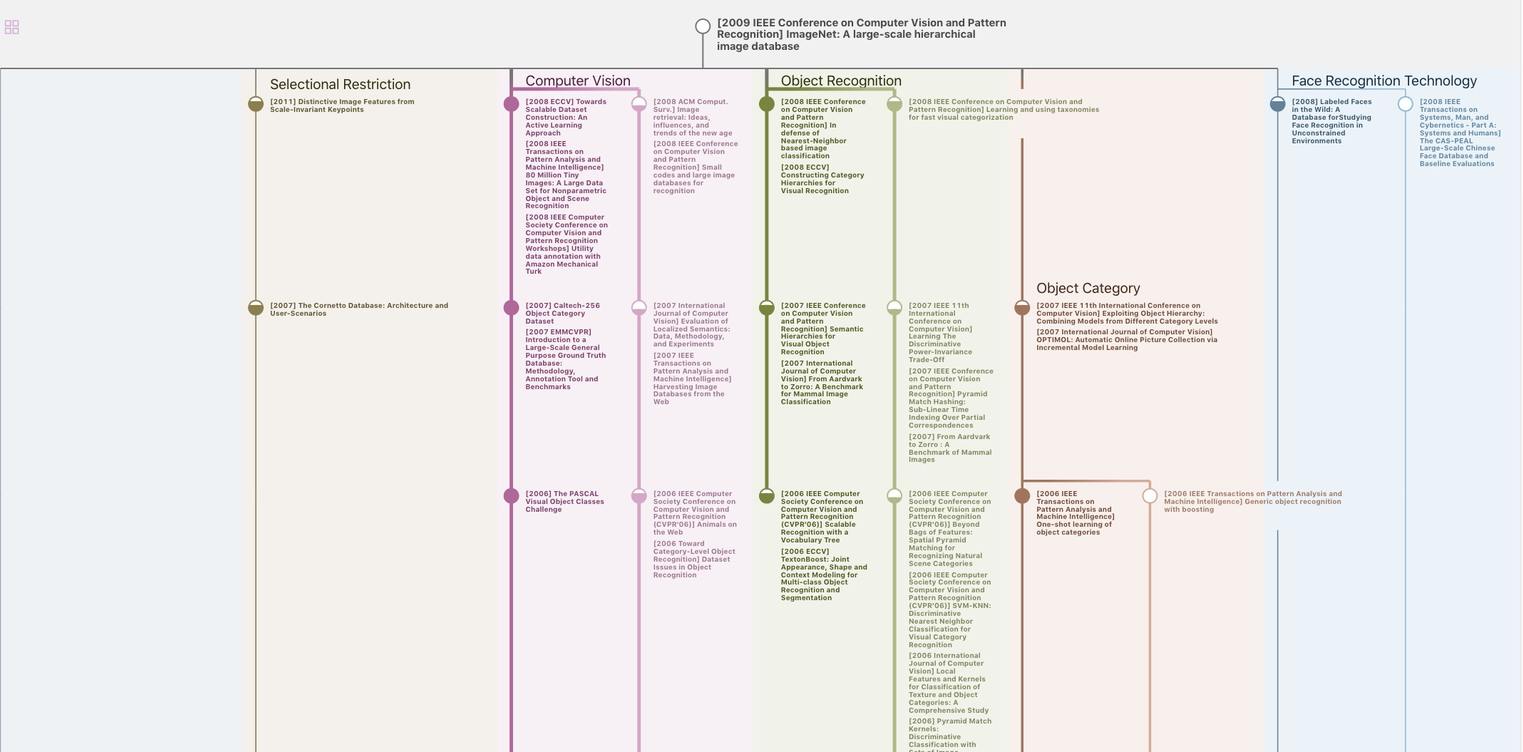
生成溯源树,研究论文发展脉络
Chat Paper
正在生成论文摘要