A novel feature-based model for zero-shot object detection with simulated attributes
Applied Intelligence(2021)
摘要
Zero-shot object detection (ZSD) has recently been proposed for detecting objects whose categories have never been seen during training. Existing ZSD works have some drawbacks: (a) the end-to-end methods sacrifice the mean accuracy precision (mAP) on seen classes; (b) the feature-based methods could avoid the above problem but suffer from simple feature construction. Thus, in this paper, we present a succinct but effective feature-based ZSD model whose feature construction naturally leverages the deep feature embedding of the detector itself as the visual features of the detected objects. The features we utilize, named “Detection Feature” (DetFeat), contain not only visual representations but also context and position information, which provide more discriminative information for seen and unseen objects. Additionally, we simulate the construction of the attributes defined by human experts to generate the specific label embedding for the ZSD task, named “Simulated Attributes” (Simu-Attr). We find that Simu-attr promotes better alignment between visual and semantic space for alleviating the problem of the semantic gap. Extensive experiments show that our approach improves the detection performance on unseen classes while maintaining the high detection performance on seen classes. On the challenging COCO dataset, we surpass the best existing transductive ZSD TL-ZSD with about 1% on unseen class and about 10% on seen class using mAP as metric.
更多查看译文
关键词
Zero-shot,Object detection,Feature embedding,Deep learning
AI 理解论文
溯源树
样例
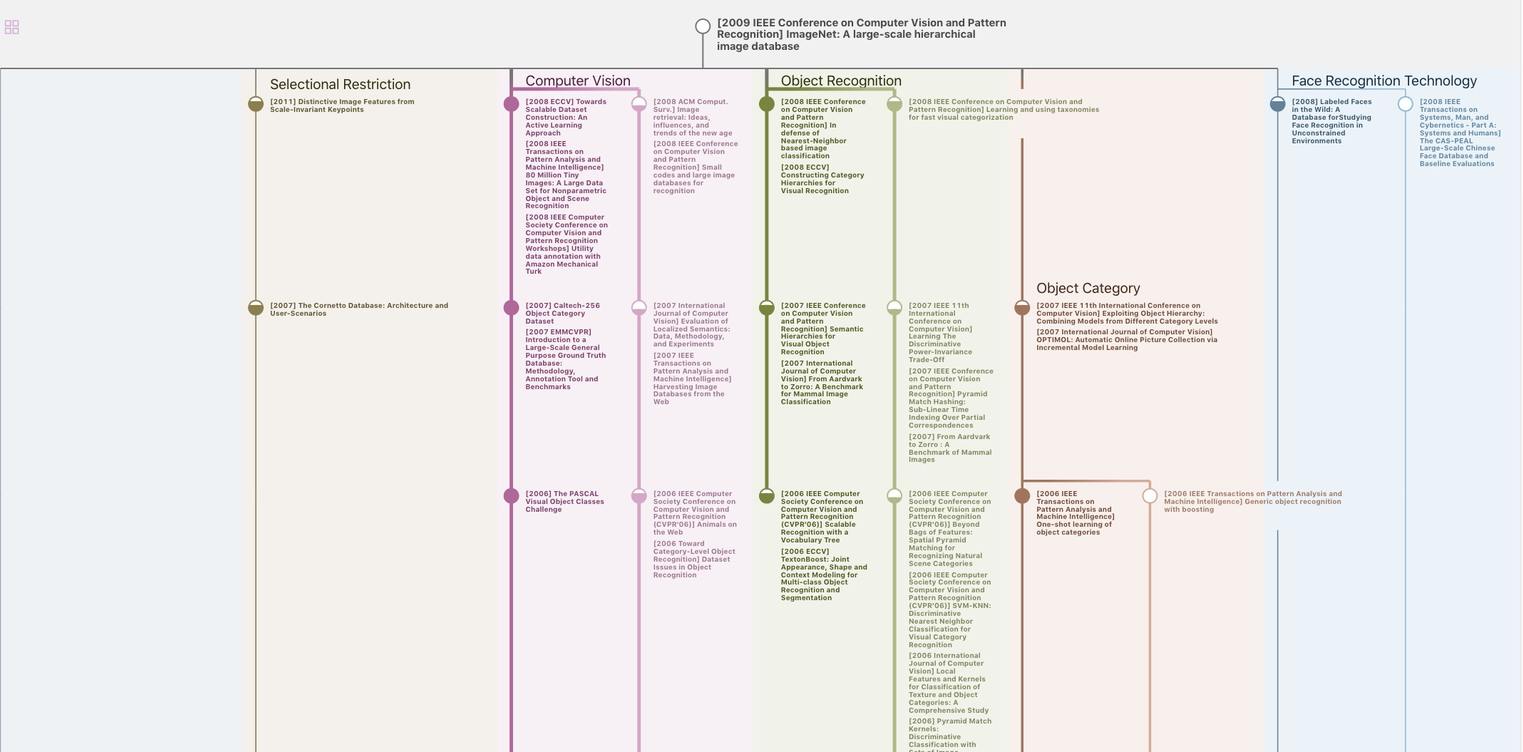
生成溯源树,研究论文发展脉络
Chat Paper
正在生成论文摘要