Structure Learning in Predictive Processing Needs Revision
Computational Brain & Behavior(2022)
摘要
The predictive processing account aspires to explain all of cognition using a single, unifying principle. Among the major challenges is to explain how brains are able to infer the structure of their generative models. Recent attempts to further this goal build on existing ideas and techniques from engineering fields, like Bayesian statistics and machine learning. While apparently promising, these approaches make specious assumptions that effectively confuse structure learning with Bayesian parameter estimation in a fixed state space. We illustrate how this leads to a set of theoretical problems for the predictive processing account. These problems highlight a need for developing new formalisms specifically tailored to the theoretical aims of scientific explanation. We lay the groundwork for a possible way forward.
更多查看译文
关键词
Predictive processing, Structure learning, Bayesian inference, Model expansion
AI 理解论文
溯源树
样例
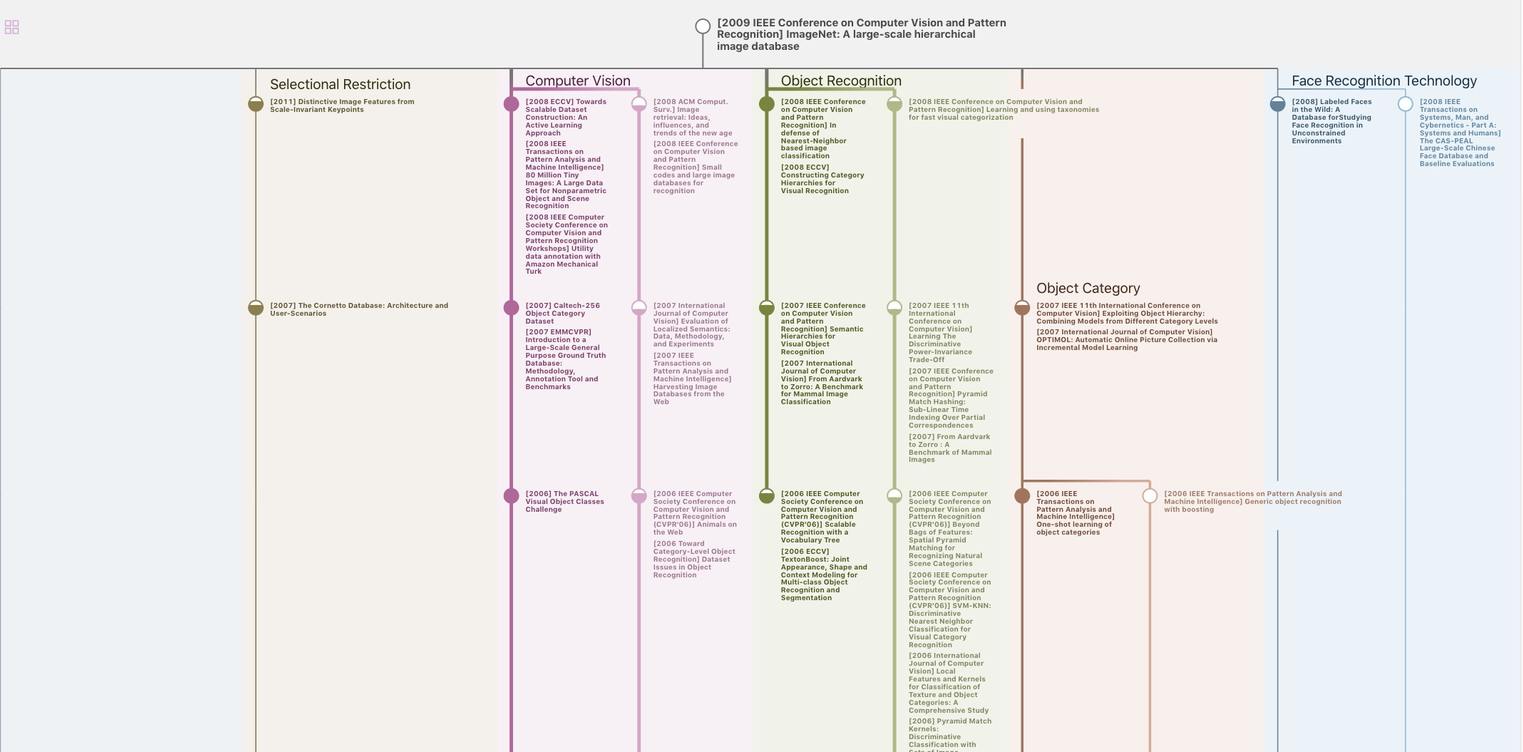
生成溯源树,研究论文发展脉络
Chat Paper
正在生成论文摘要