A Hybrid Multi-Step Sensitivity-Driven Evolutionary Polynomial Regression Enables Robust Model Structure Selection
Engineering applications of artificial intelligence(2022)
摘要
Evolutionary Polynomial Regression (EPR) has found widespread application and use for model structure development in engineering and science. This hybrid evolutionary approach merges real world data and explanatory variables to generate well-structured models in the form of polynomial equations. The simple and transparent models produced by this technique enable us to explore, via sensitivity analysis, the robustness of the derived models. Yet, existing EPR frameworks do not make explicit use of sensitivity analysis in the selection of robust and high-fidelity model structures. In this paper, we develop a multi-step sensitivity-driven method which combines the strengths of differential evolution and model selection via Monte Carlo simulation to explore the input–output relationships of model structures. In the first step, our hybrid approach automatically determines the optimum number of terms of the polynomial equations. In a subsequent step, our algorithm explores the mean parametric response of each explanatory variable used in the mathematical formulation to select a final model structure. Finally, in our selection of the most robust mathematical structure, we take explicit consideration of the prediction uncertainty of the simulated output. We illustrate and evaluate our EPR method for different engineering problems involving modeling and prediction of the moisture content and creep index of soils. Altogether, our results demonstrate that the use of sensitivity analysis as an integral part of model structure search and selection will lead to robust models with high predictive ability.
更多查看译文
关键词
Evolutionary polynomial regression,Model selection,Monte Carlo simulations,Sensitivity analysis,Optimum moisture content,Creep index
AI 理解论文
溯源树
样例
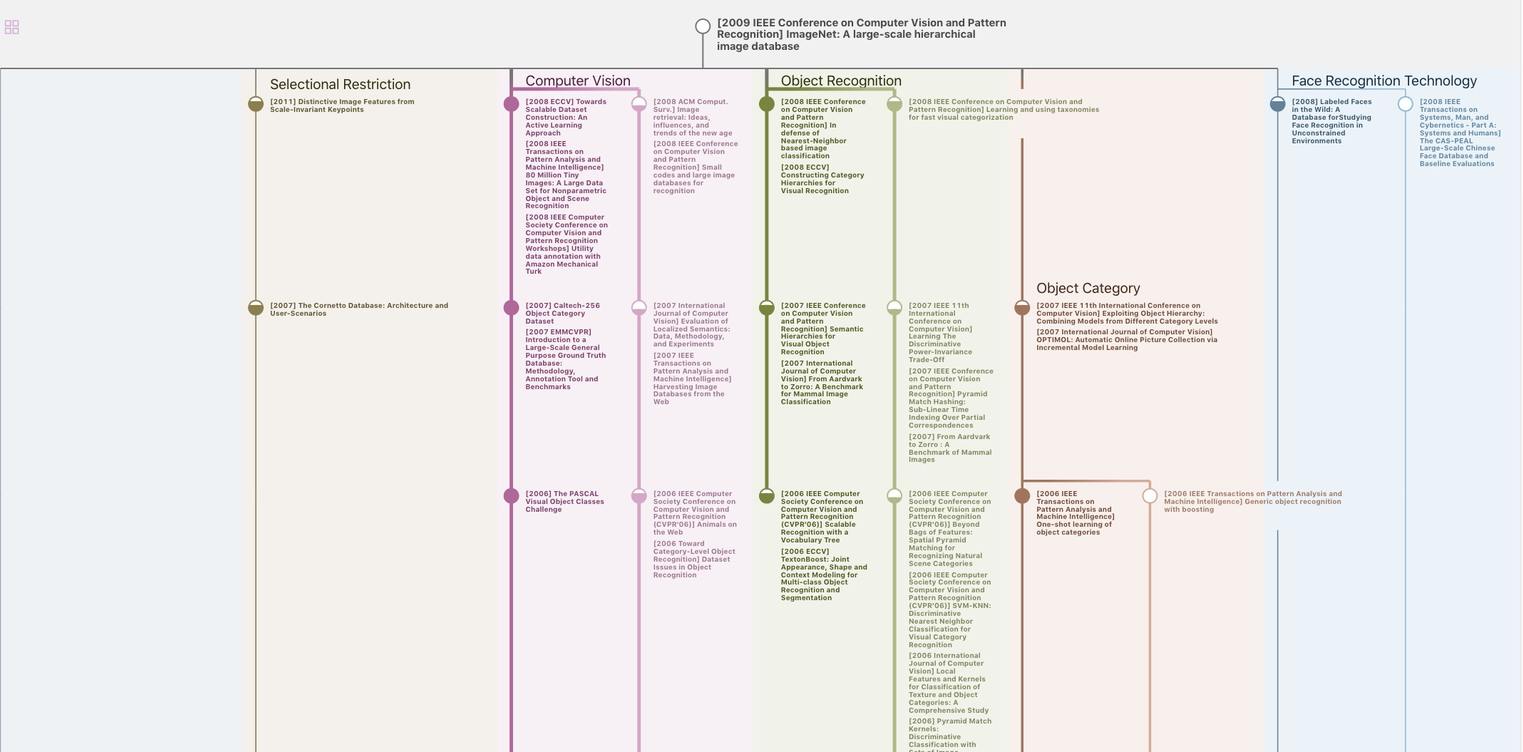
生成溯源树,研究论文发展脉络
Chat Paper
正在生成论文摘要