Learning user preferences through online conversations via personalized memory transfer
Information Retrieval Journal(2022)
摘要
Dialogue systems are becoming an increasingly common part of many users’ daily routines. Natural language serves as a convenient interface to express our preferences with the underlying systems. In this paper, we aim to learn user preferences through online conversations. Compared to the traditional collaborative filtering setting where feedback is provided quantitatively, conversational users may only indicate their preferences at a high level with inexact item mentions. To tackle the ambiguities in natural language conversations, we propose Personalized Memory Transfer which learns a personalized model in an online manner by leveraging a key-value memory structure to distill user feedback directly from conversations. This memory structure enables the integration of prior knowledge to transfer existing item representations/preferences and natural language representations. The experiments were conducted on two public datasets and the results demonstrate the effectiveness of the proposed approach.
更多查看译文
关键词
Conversational recommendation, Memory networks, Transfer learning
AI 理解论文
溯源树
样例
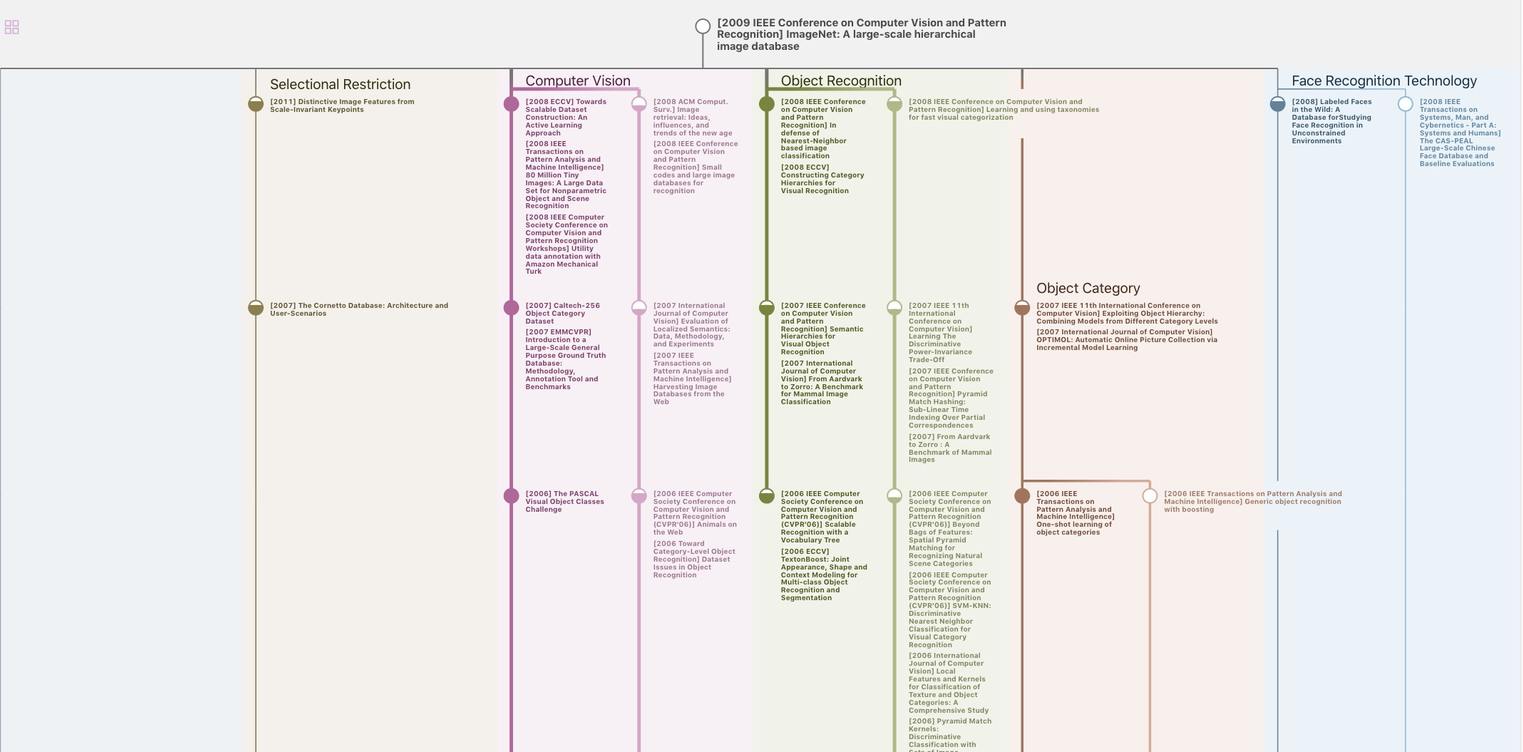
生成溯源树,研究论文发展脉络
Chat Paper
正在生成论文摘要