CI-OCM: Counterfactural Inference towards Unbiased Outfit Compatibility Modeling.
Proceedings of the 1st Workshop on Multimedia Computing towards Fashion Recommendation(2022)
摘要
As a key task to support intelligent fashion shop construction, outfit compatibility modeling, which aims to estimate whether the given set of fashion items makes a compatible outfit, has attracted much research attention. Although previous efforts have achieved compelling success, they still suffer from the spurious correlation between the category matching and outfit compatibility, which hurts the generalization of the model and misleads the model to be biased. To tackle this problem, we introduce the causal graph tool to analyze the causal relationship among variables of outfit compatibility modeling. In particular, we find that the spurious correlation is attributed to the direct effect of the category information on outfit compatibility prediction by the causal graph. To remove this bad effect from the category information, we present a novel counterfactual inference framework for outfit compatibility modeling, dubbed as CI-OCM. Thereinto, we capture the direct effect of the category information on model prediction in the training phase and then subtract it from the total effect in the testing phase to achieve debiased prediction. Extensive experiments on two splits of a widely-used dataset~(\ie under the independent identically distribution and out-of-distribution assumptions) clearly demonstrate that our CI-OCM can achieve significant improvement over the existing baselines. In addition, we released our code to facilitate the research community.
更多查看译文
AI 理解论文
溯源树
样例
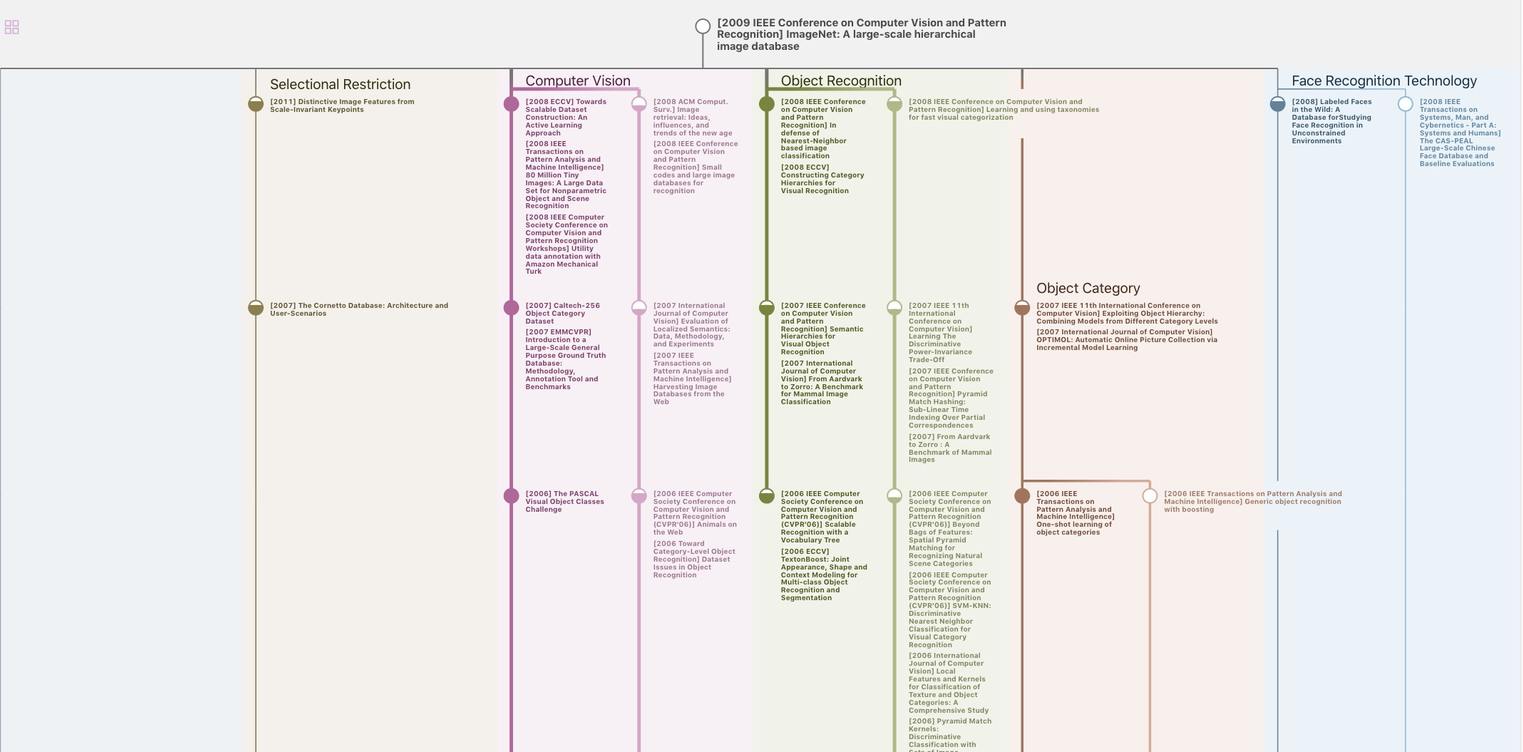
生成溯源树,研究论文发展脉络
Chat Paper
正在生成论文摘要