Evolving fuzzy predictor with multivariable Gaussian participatory learning and multi-innovations recursive weighted least squares: eFMI
Evolving Systems(2022)
摘要
This paper proposes a new evolving fuzzy model constructed with an unsupervised recursive clustering algorithm with participatory learning and multivariable Gaussian membership functions. The proposed model, called evolving fuzzy with multivariable Gaussian participatory learning and multi-innovations recursive weighted least squares, uses first-order Takagi-Sugeno functional rules. The rules are extracted by the clustering algorithm that can add a new cluster, delete, merge or update existing clusters. The clusters are created using a compatibility measure and an arousal mechanism. The compatibility measure is computed by Euclidian or Mahalanobis distance according to the cluster’s number of samples. The clusters exclusion method combines age and population to exclude inactive clusters. Redundant clusters are merged if there is a noticeable overlap between two clusters. The consequent parameters are updated by a multi-innovations weighted least squares recursive algorithm. The performance of the eFMI is evaluated and compared with alternative state-of-the-art evolving models in times series forecasting and non-linear system identification problems. Computational experiments and comparisons suggest the proposed model performs better or comparable than the alternative evolving models.
更多查看译文
关键词
Evolving systems,Adaptive models,Fuzzy systems,Multivariable Gaussian,Forecasting,Non-linear system identification
AI 理解论文
溯源树
样例
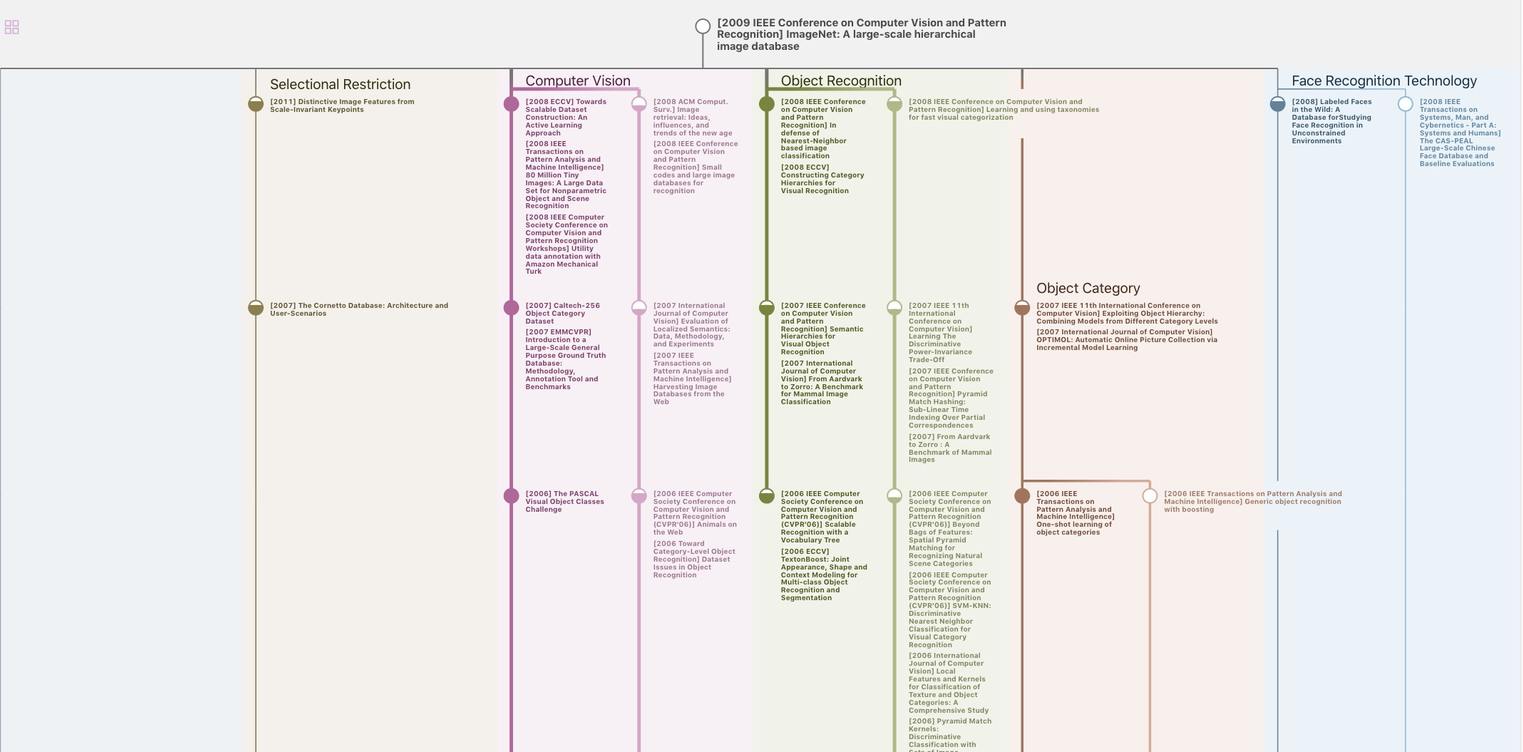
生成溯源树,研究论文发展脉络
Chat Paper
正在生成论文摘要