Regional neuroanatomic effects on brain age inferred using magnetic resonance imaging and ridge regression.
JOURNALS OF GERONTOLOGY SERIES A-BIOLOGICAL SCIENCES AND MEDICAL SCIENCES(2022)
摘要
The biological age of the brain differs from its chronological age and can be used as biomarker of neural/cognitive disease processes and as predictor of mortality. Brain age is often estimated from magnetic resonance images (MRIs) using machine learning (ML) that rarely indicates how regional brain features contribute to brain age. Leveraging an aggregate training sample of 3418 healthy controls (HCs), we describe a ridge regression model that quantifies each region's contribution to brain age. After model testing on an independent sample of 651 HCs, we compute the coefficient of partial determination Rp2 for each regional brain volume to quantify its contribution to brain age. Model performance is also evaluated using the correlation r between chronological and biological ages, the mean absolute error (MAE) and mean squared error (MSE) of brain age estimates. On training data, r=0.92, MSE=70.94 years (y), MAE=6.57 y, and R2=0.81; on test data, r=0.90, MSE=81.96 y, MAE=7.00 y, and R2=0.79. The regions whose volumes contribute most to brain age are the nucleus accumbens (Rp2=7.27%), inferior temporal gyrus (Rp2=4.03%), thalamus (Rp2=3.61%), brainstem (Rp2=3.29%), posterior lateral sulcus (Rp2=3.22%), caudate nucleus (Rp2=3.05%), orbital gyrus (Rp2=2.96%), and precentral gyrus (Rp2=2.80%). Our ridge regression, although outperformed by the most sophisticated ML approaches, identifies the importance and relative contribution of each brain structure to overall brain age. Aside from its interpretability and quasi-mechanistic insights, our model can be used to validate future ML approaches for brain age estimation.
更多查看译文
关键词
Brain Aging,Cognitive Decline,Human Aging,Imaging
AI 理解论文
溯源树
样例
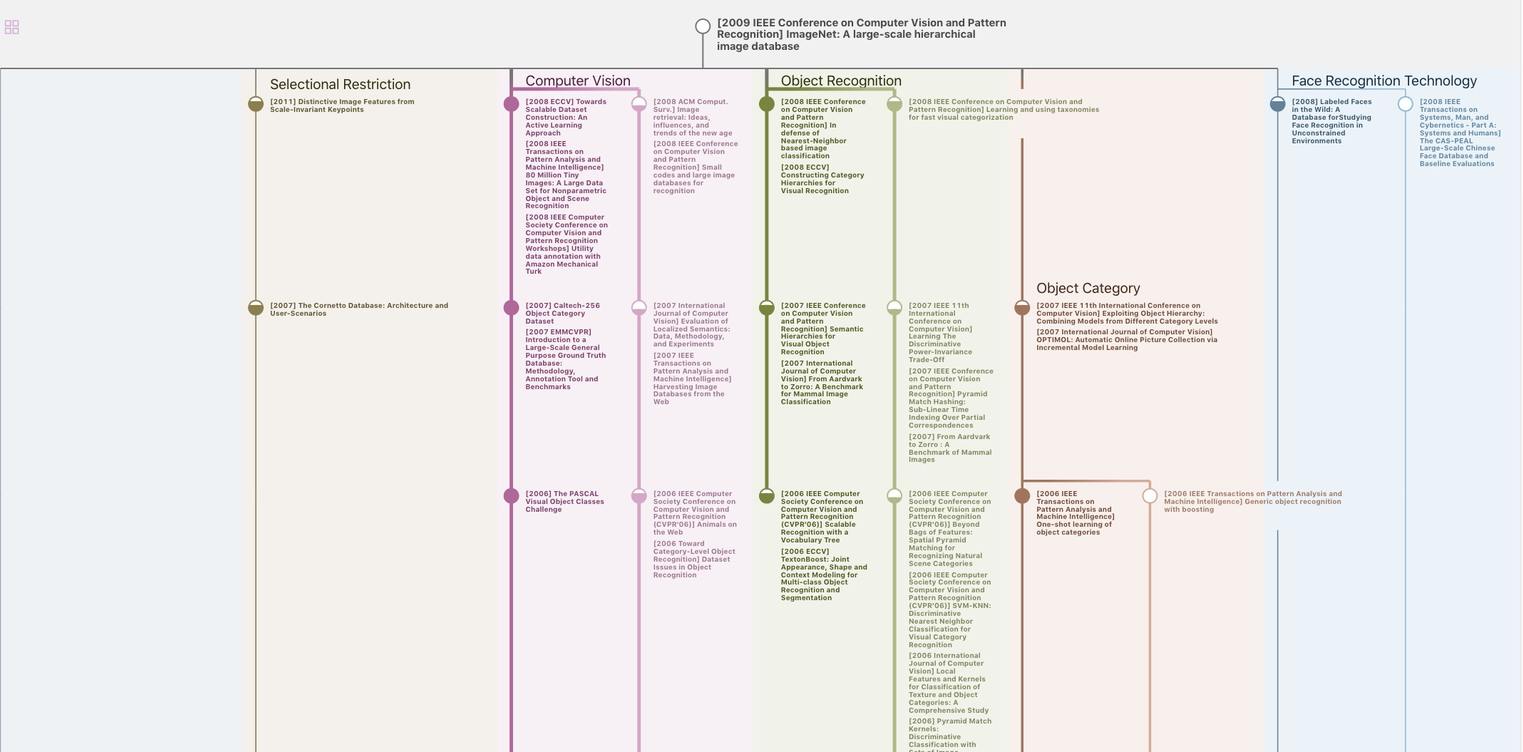
生成溯源树,研究论文发展脉络
Chat Paper
正在生成论文摘要