Effective Early Stopping of Point Cloud Neural Networks.
Modeling Decisions for Artificial Intelligence (MDAI)(2022)
摘要
Early stopping techniques can be utilized to decrease the time cost, however currently the ultimate goal of early stopping techniques is closely related to the accuracy upgrade or the ability of the neural network to generalize better on unseen data without being large or complex in structure and not directly with its efficiency. Time efficiency is a critical factor in neural networks, especially when dealing with the segmentation of 3D point cloud data, not only because a neural network itself is computationally expensive, but also because point clouds are large and noisy data, making learning processes even more costly. In this paper, we propose a new early stopping technique based on fundamental mathematics aiming to upgrade the trade-off between the learning efficiency and accuracy of neural networks dealing with 3D point clouds. Our results show that by employing our early stopping technique in four distinct and highly utilized neural networks in segmenting 3D point clouds, the training time efficiency of the models is greatly improved, with efficiency gain values reaching up to 94\%, while the models achieving in just a few epochs approximately similar segmentation accuracy metric values like the ones that are obtained in the training of the neural networks in 200 epochs. Also, our proposal outperforms four conventional early stopping approaches in segmentation accuracy, implying a promising innovative early stopping technique in point cloud segmentation.
更多查看译文
关键词
Deep learning,Point clouds,Segmentation,Efficiency,Early stopping
AI 理解论文
溯源树
样例
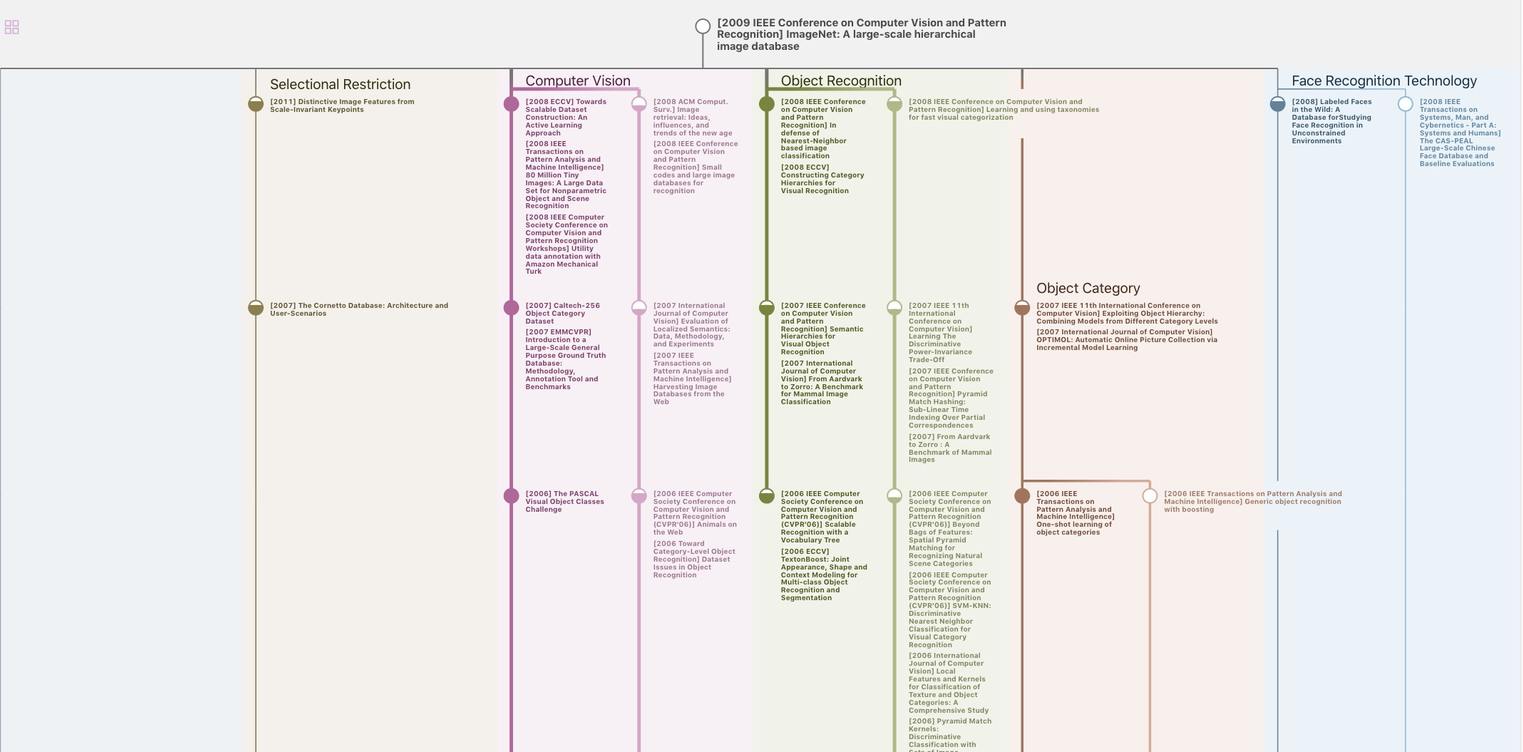
生成溯源树,研究论文发展脉络
Chat Paper
正在生成论文摘要