Observational Robustness and Invariances in Reinforcement Learning via Lexicographic Objectives
arxiv(2022)
摘要
Policy robustness in Reinforcement Learning (RL) may not be desirable at any price; the alterations caused by robustness requirements from otherwise optimal policies should be explainable and quantifiable. Policy gradient algorithms that have strong convergence guarantees are usually modified to obtain robust policies in ways that do not preserve algorithm guarantees, which defeats the purpose of formal robustness requirements. In this work we study a notion of robustness in partially observable MDPs where state observations are perturbed by a noise-induced stochastic kernel. We characterise the set of policies that are maximally robust by analysing how the policies are altered by this kernel. We then establish a connection between such robust policies and certain properties of the noise kernel, as well as with structural properties of the underlying MDPs, constructing sufficient conditions for policy robustness. We use these notions to propose a robustness-inducing scheme, applicable to any policy gradient algorithm, to formally trade off the reward achieved by a policy with its robustness level through lexicographic optimisation, which preserves convergence properties of the original algorithm. We test the the proposed approach through numerical experiments on safety-critical RL environments, and show how the proposed method helps achieve high robustness when state errors are introduced in the policy roll-out.
更多查看译文
关键词
Robust Reinforcement Learning,Safe Reinforcement Learning,Lexicographic Optimization
AI 理解论文
溯源树
样例
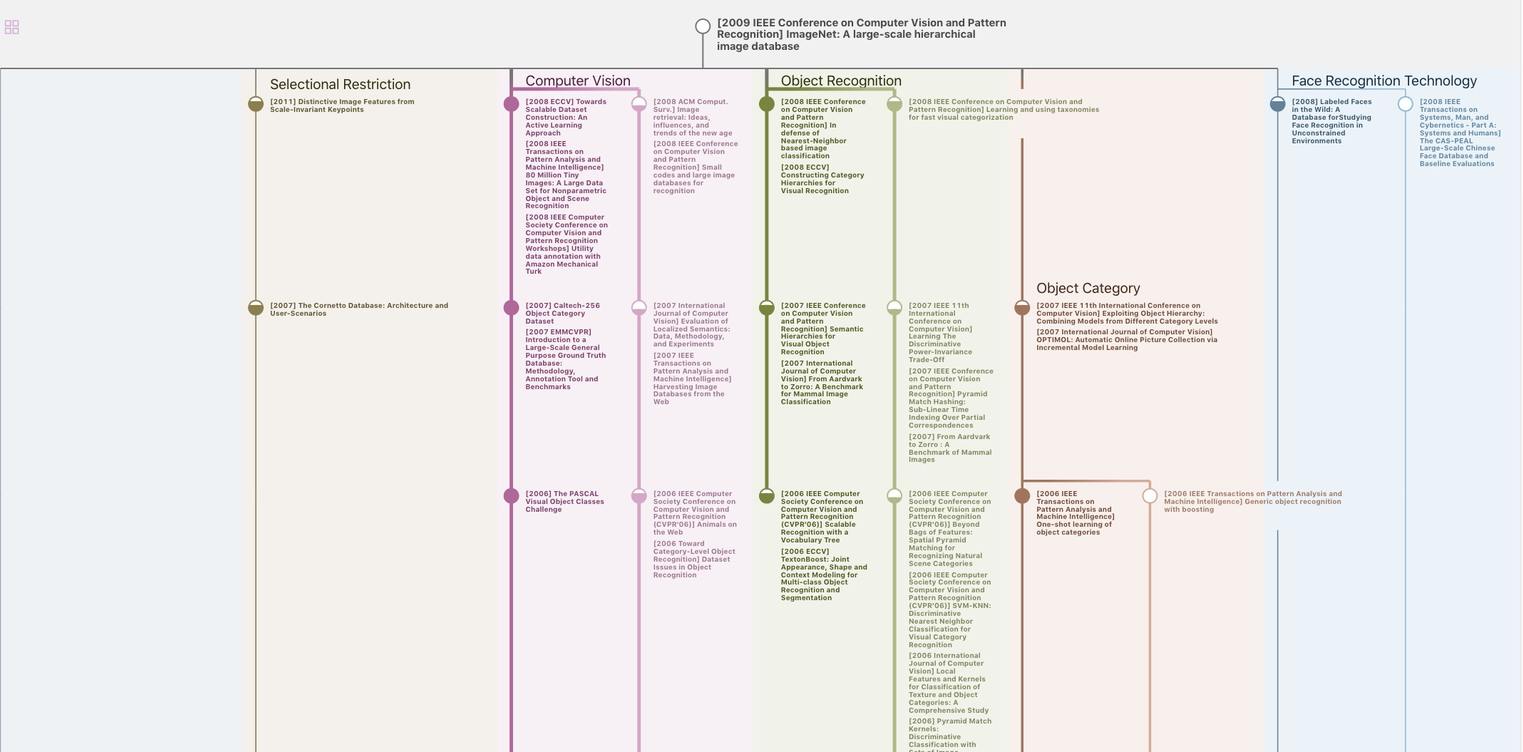
生成溯源树,研究论文发展脉络
Chat Paper
正在生成论文摘要