Multimodal System Framework for Feature Level Fusion based on CCA with SVM Classifier
2020 IEEE-HYDCON(2020)
摘要
In the biometric recognition, feature fusion is a critical step, especially in case of heterogeneous and incompatible feature representation. Most of the existing methods cannot extract features that are discriminative and at the same time affine invariant, which limits the classification performance. To address this issue, we propose an efficient feature level fusion approach using canonical correlation analysis (CCA) with SVM classification. The feature level fusion approach integrates different features extracted from different modalities into a more discriminative and compact feature representation, which can be further used for classification. We also propose an efficient algorithm that optimizes the whole framework of the multimodal system. The CCA + PCA subspace learning approach is presented to couple dimensionality reduction and feature fusion collectively, which can be used for both unsupervised and supervised learning. The experimental results on the SDUMLA multimodal database show that the proposed approach significantly outperforms than the prevalent feature fusion approaches. We demonstrate that an efficient multimodal classification can be achieved with a significant reduction in the number of feature dimensions by exploiting canonical correlation analysis on the extracted feature sets from iris and fingerprint modalities. We also demonstrate that subspace learning maximizes the correlation between Iris and Fingerprint modalities and excels the recognition performance of the individual modality.
更多查看译文
关键词
Feature Fusion,Multimodal Biometric System,Iris and Fingerprint Biometric,Canonical Correlation Analysis,Support Vector Machine
AI 理解论文
溯源树
样例
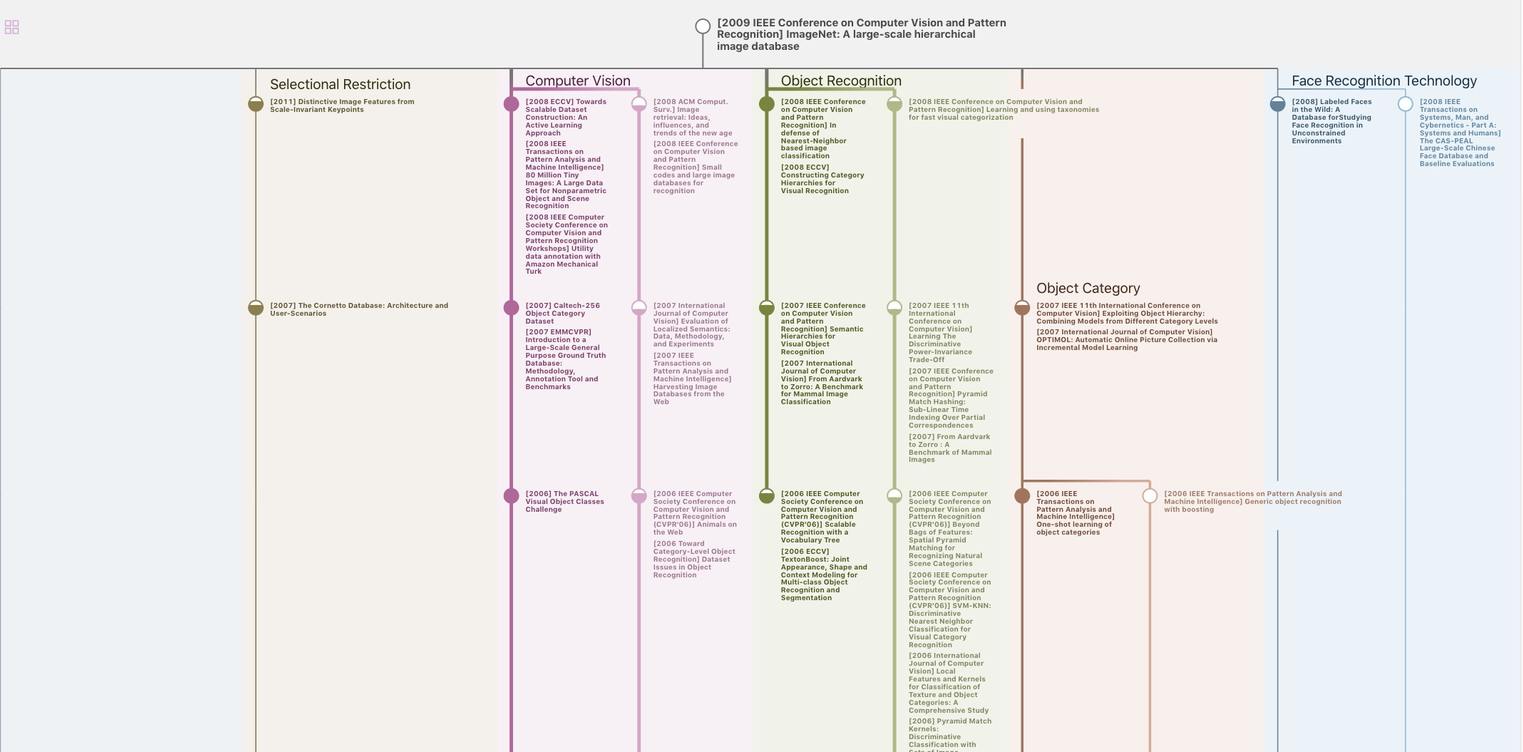
生成溯源树,研究论文发展脉络
Chat Paper
正在生成论文摘要