Adaptive Local Reinforce Attention Network for Few-shot Learning
2022 IEEE 10th Joint International Information Technology and Artificial Intelligence Conference (ITAIC)(2022)
摘要
In many real-world application scenarios, limited to the economic, time and labor factors, it is not feasible to collect a large-scale labeled dataset. Therefore, researchers proposed few-shot learning methods that do not rely on large-scale data training. However, existing few-shot learning methods only use the global feature map of an image to predict its class, ignoring the role of information of local details with category characteristics in the image for classification. In this paper, we propose an adaptive local reinforce attention network (ALRANet) that can capture and strengthen information of local details. By dividing the learned knowledge, different kinds of learned knowledge are used to guide the capture and enhancement of local details in the new sample, and the content of learned knowledge is dynamically updated according to the learning performance. Extensive experiments are conducted on two datasets, and the proposed ALRA model outperforms those existing model, demonstrating the effectiveness of our model.
更多查看译文
关键词
deep learning,few-shot learning,adaptive local reinforce attention network,local details,image classification
AI 理解论文
溯源树
样例
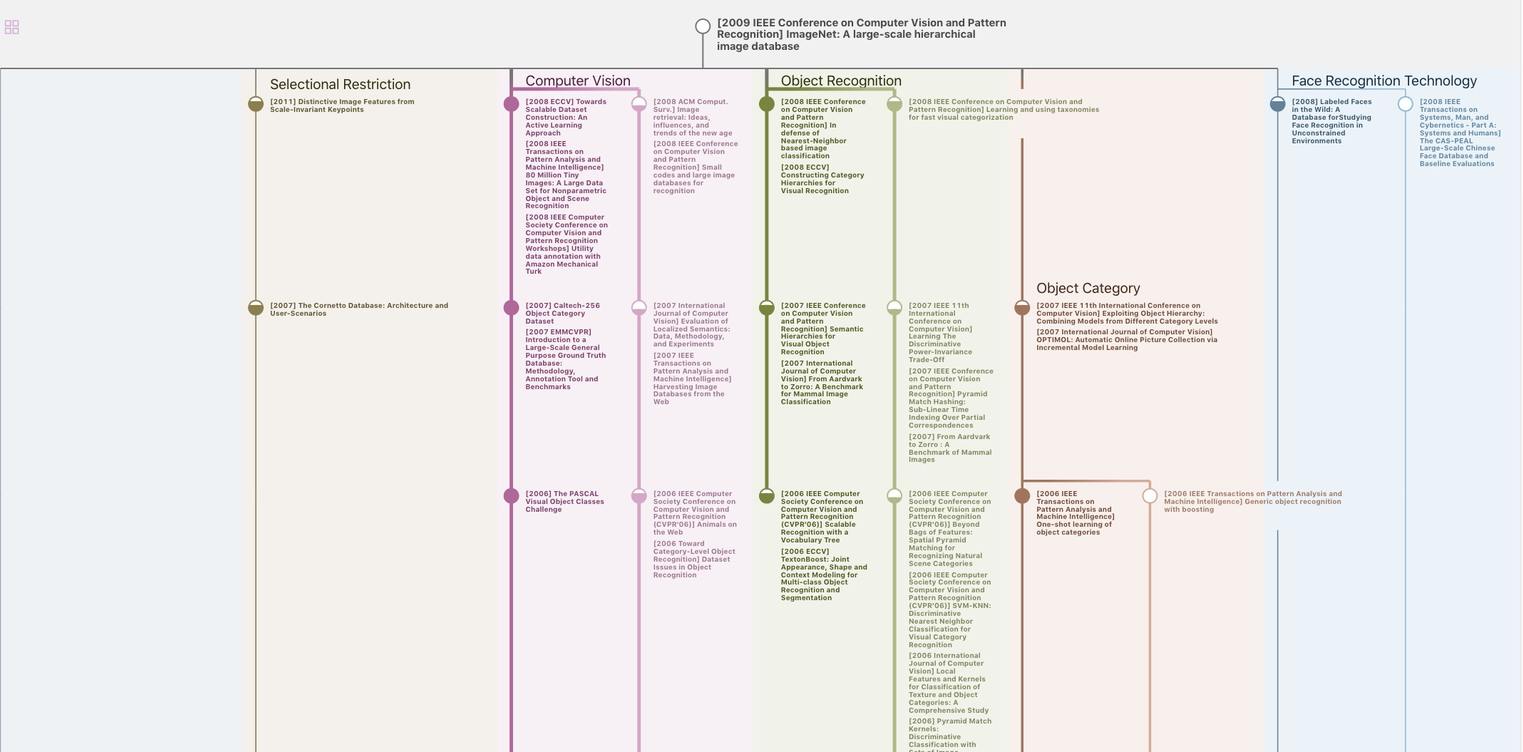
生成溯源树,研究论文发展脉络
Chat Paper
正在生成论文摘要