Shockwave: Fair and Efficient Cluster Scheduling for Dynamic Adaptation in Machine Learning
arxiv(2022)
摘要
Dynamic adaptation has become an essential technique in accelerating distributed machine learning (ML) training. Recent studies have shown that dynamically adjusting model structure (e.g., lottery ticket hypothesis) or hyperparameters (e.g., batch size) can significantly accelerate training without sacrificing accuracy. However, existing ML cluster schedulers are not designed to handle dynamic adaptation. We show that existing schemes fail to provide fairness and degrade system efficiency when the training throughput changes over time under dynamic adaptation. We design Shockwave, a scheduler with future planning that builds on two key ideas. First, Shockwave extends classic market theory from static settings to dynamic settings to co-optimize efficiency and fairness. Second, Shockwave utilizes stochastic dynamic programming to handle dynamic changes. We build a system for Shockwave and validate its performance with both trace-driven simulation and cluster experiments. Results show that for traces of ML jobs with dynamic adaptation, Shockwave improves makespan by 1.3X and fairness by 2X when compared with existing fair scheduling schemes.
更多查看译文
AI 理解论文
溯源树
样例
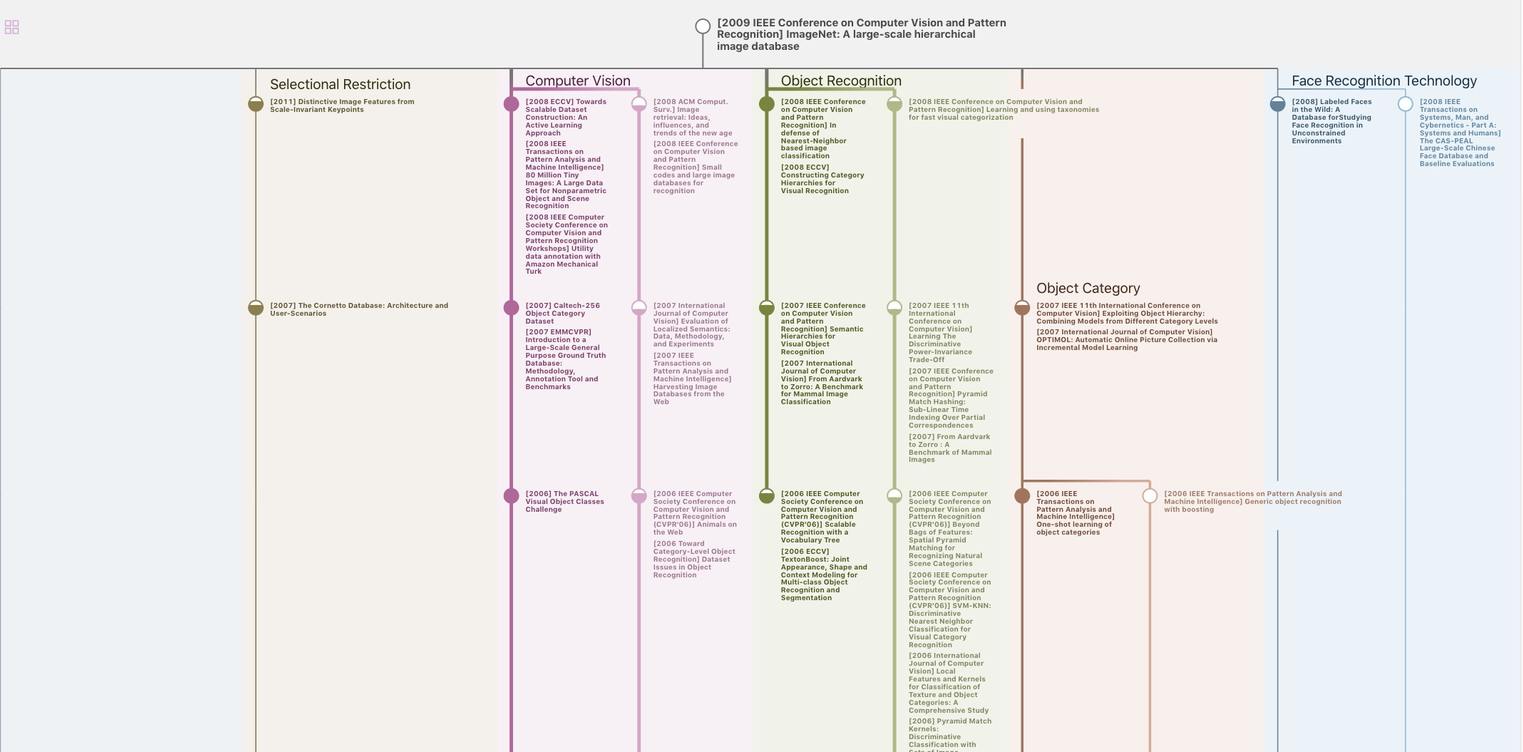
生成溯源树,研究论文发展脉络
Chat Paper
正在生成论文摘要