DCI-ES: An Extended Disentanglement Framework with Connections to Identifiability
ICLR 2023(2022)
摘要
In representation learning, a common approach is to seek representations which disentangle the underlying factors of variation. Eastwood & Williams (2018) proposed three metrics for quantifying the quality of such disentangled representations: disentanglement (D), completeness (C) and informativeness (I). In this work, we first connect this DCI framework to two common notions of linear and nonlinear identifiability, thus establishing a formal link between disentanglement and the closely-related field of independent component analysis. We then propose an extended DCI-ES framework with two new measures of representation quality - explicitness (E) and size (S) - and point out how D and C can be computed for black-box predictors. Our main idea is that the functional capacity required to use a representation is an important but thus-far neglected aspect of representation quality, which we quantify using explicitness or ease-of-use (E). We illustrate the relevance of our extensions on the MPI3D and Cars3D datasets.
更多查看译文
关键词
disentanglement,identifiability,representation learning
AI 理解论文
溯源树
样例
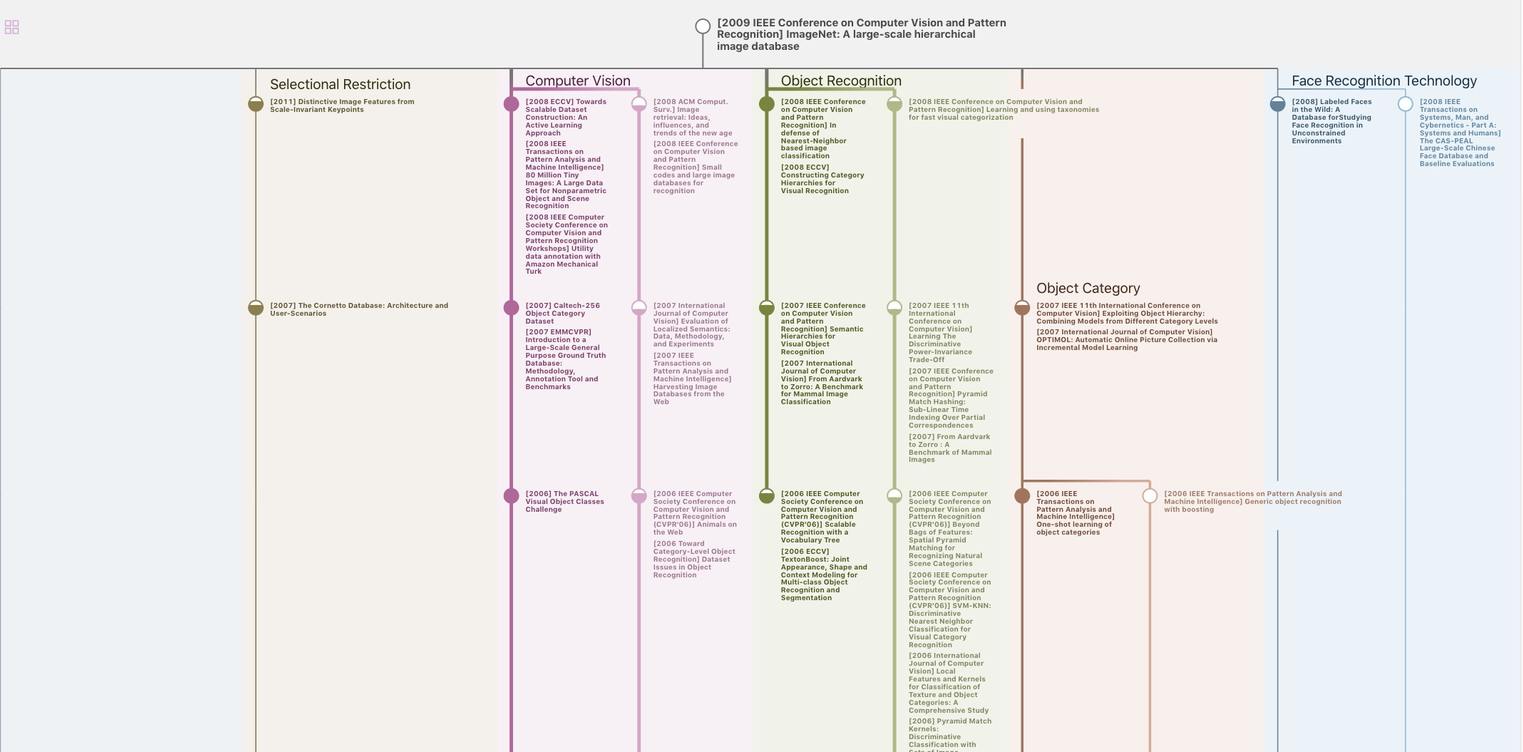
生成溯源树,研究论文发展脉络
Chat Paper
正在生成论文摘要