Fine-grained Contrastive Learning for Definition Generation.
AACL/IJCNLP (1)(2022)
摘要
Recently, pre-trained transformer-based models have achieved great success in the task of definition generation (DG). However, previous encoder-decoder models lack effective representation learning to contain full semantic components of the given word, which leads to generating under-specific definitions. To address this problem, we propose a novel contrastive learning method, encouraging the model to capture more detailed semantic representations from the definition sequence encoding. According to both automatic and manual evaluation, the experimental results on three mainstream benchmarks demonstrate that the proposed method could generate more specific and high-quality definitions compared with several state-of-the-art models.
更多查看译文
关键词
contrastive learning,definition generation,fine-grained
AI 理解论文
溯源树
样例
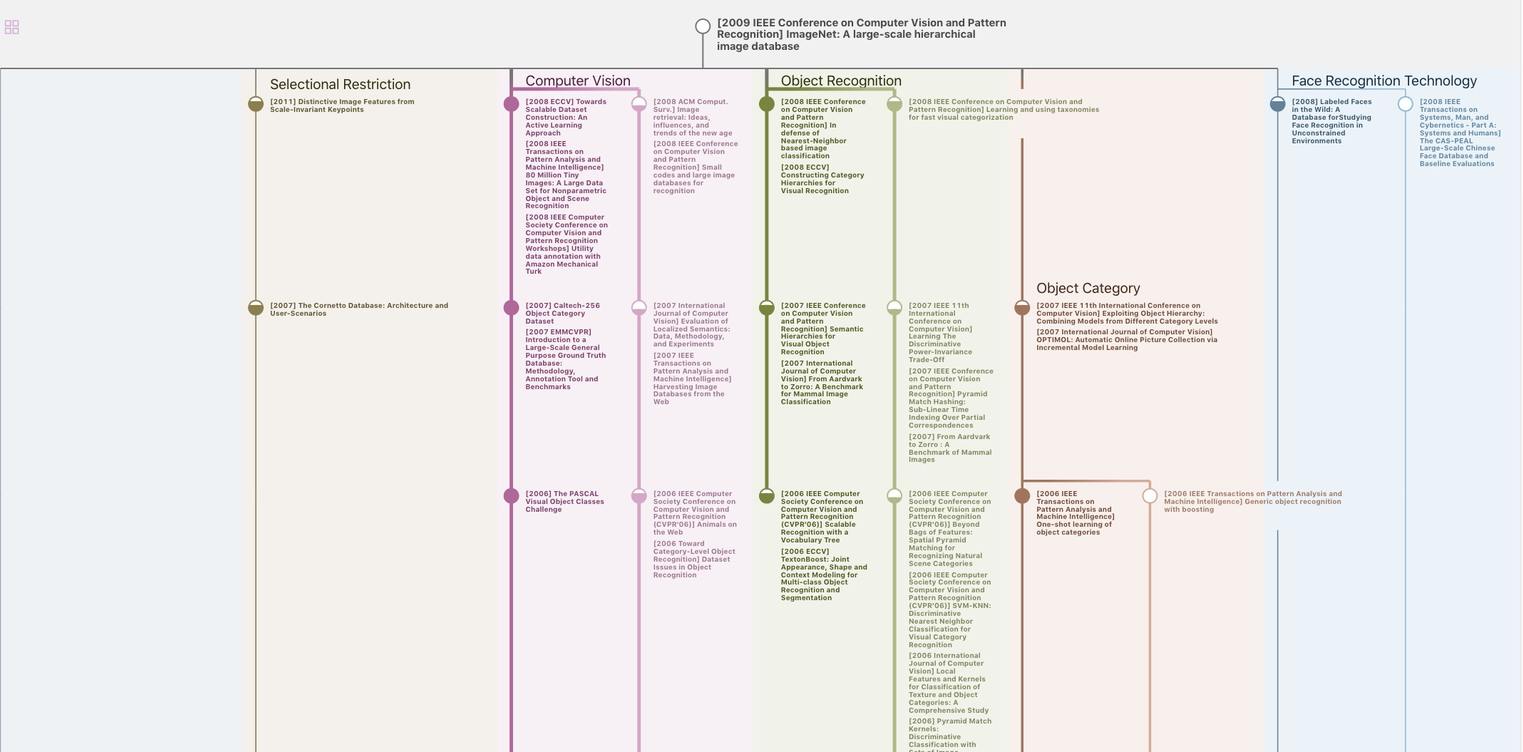
生成溯源树,研究论文发展脉络
Chat Paper
正在生成论文摘要