Policy Gradients for Probabilistic Constrained Reinforcement Learning
arxiv(2022)
摘要
This paper considers the problem of learning safe policies in the context of reinforcement learning (RL). In particular, a safe policy or controller is one that, with high probability, maintains the trajectory of the agent in a given safe set. We relate this notion of safety to the notion of average safety often considered in the literature by providing theoretical bounds in terms of their safety and performance. The challenge of working with the probabilistic notion of safety considered in this work is the lack of expressions for their gradients. Indeed, policy optimization algorithms rely on gradients of the objective function and the constraints. To the best of our knowledge, this work is the first one providing such explicit gradient expressions for probabilistic constraints. It is worth noting that such probabilistic gradients are naturally algorithm independent, which provides possibilities for them to be applied to various policy-based algorithms. In addition, we consider a continuous navigation problem to empirically illustrate the advantages (in terms of safety and performance) of working with probabilistic constraints as compared to average constraints.
更多查看译文
关键词
reinforcement learning,probabilistic constraint,safe policy,policy gradient
AI 理解论文
溯源树
样例
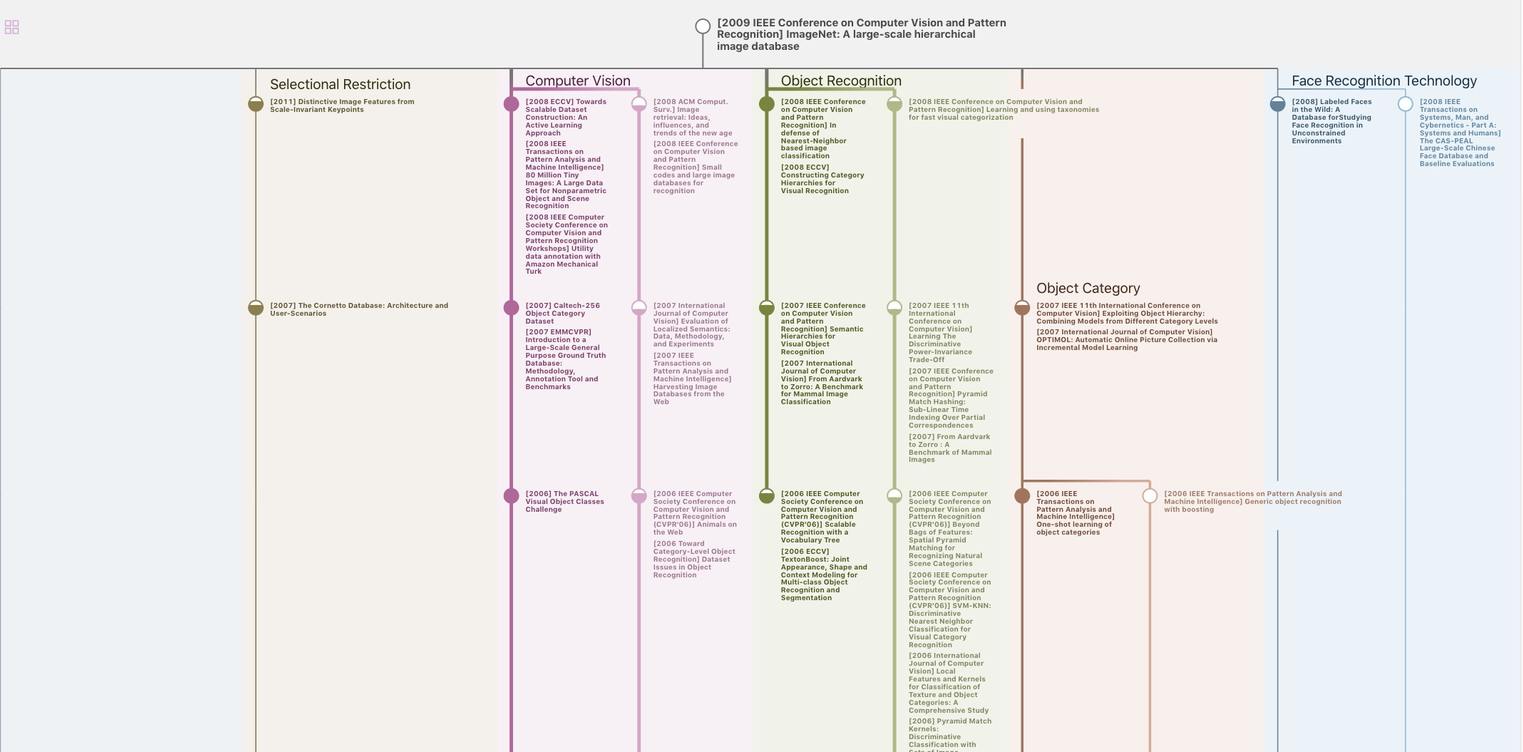
生成溯源树,研究论文发展脉络
Chat Paper
正在生成论文摘要