A flexible model for correlated count data, with application to multi-condition differential expression analyses of single-cell RNA sequencing data
arxiv(2022)
摘要
Detecting differences in gene expression is an important part of single-cell
RNA sequencing experiments, and many statistical methods have been developed
for this aim. Most differential expression analyses focus on comparing
expression between two groups (e.g., treatment vs. control). But there is
increasing interest in multi-condition differential expression analyses in
which expression is measured in many conditions, and the aim is to accurately
detect and estimate expression differences in all conditions. We show that
directly modeling single-cell RNA-seq counts in all conditions simultaneously,
while also inferring how expression differences are shared across conditions,
leads to greatly improved performance for detecting and estimating expression
differences compared to existing methods. We illustrate the potential of this
new approach by analyzing data from a single-cell experiment studying the
effects of cytokine stimulation on gene expression. We call our new method
"Poisson multivariate adaptive shrinkage", and it is implemented in an R
package available online at https://github.com/stephenslab/poisson.mash.alpha.
更多查看译文
关键词
gene expression differences,gene expression,count data,flexible model,multi-condition
AI 理解论文
溯源树
样例
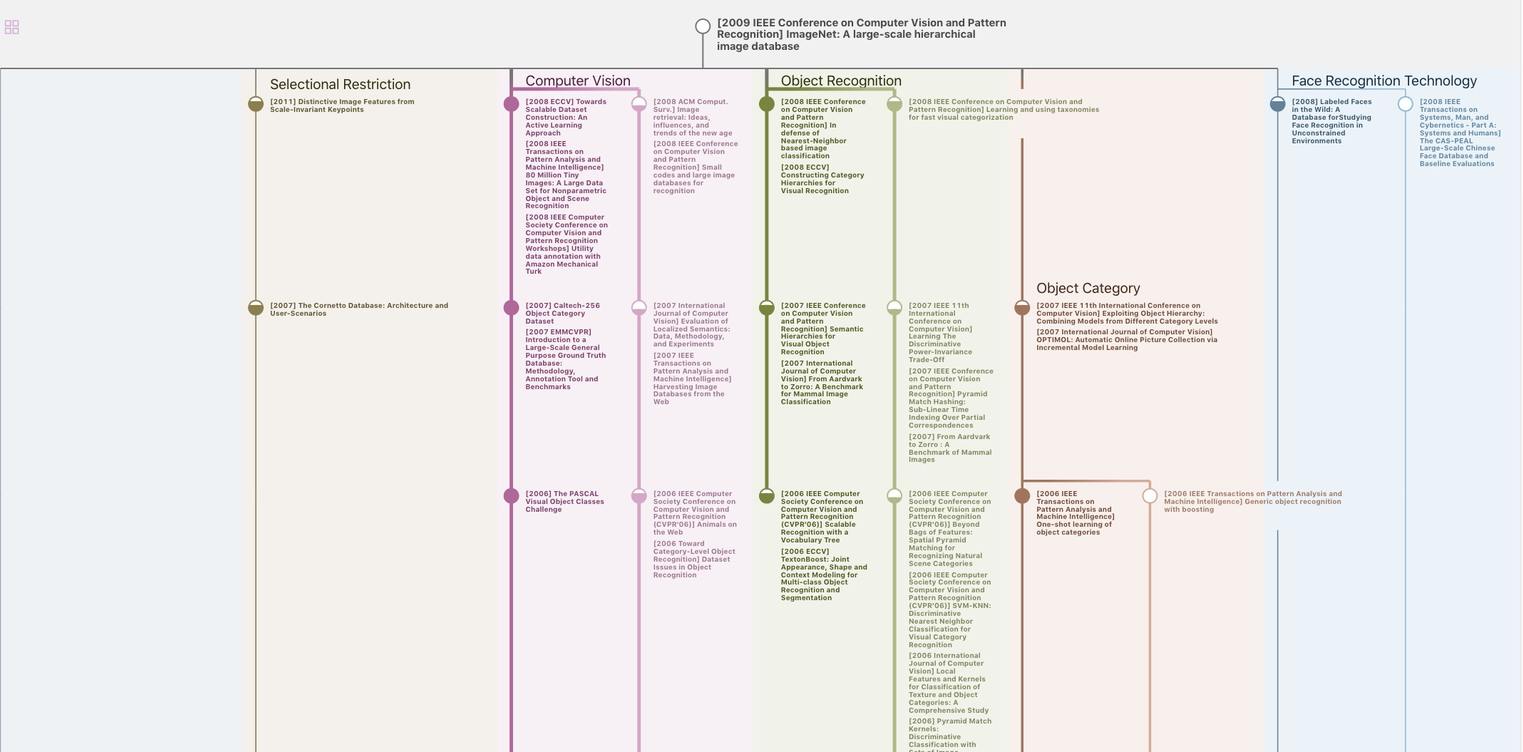
生成溯源树,研究论文发展脉络
Chat Paper
正在生成论文摘要