On the infinite-depth limit of finite-width neural networks
arxiv(2023)
摘要
In this paper, we study the infinite-depth limit of finite-width residual neural networks with random Gaussian weights. With proper scaling, we show that by fixing the width and taking the depth to infinity, the pre-activations converge in distribution to a zero-drift diffusion process. Unlike the infinite-width limit where the pre-activation converge weakly to a Gaussian random variable, we show that the infinite-depth limit yields different distributions depending on the choice of the activation function. We document two cases where these distributions have closed-form (different) expressions. We further show an intriguing change of regime phenomenon of the post-activation norms when the width increases from 3 to 4. Lastly, we study the sequential limit infinite-depth-then-infinite-width and compare it with the more commonly studied infinite-width-then-infinite-depth limit.
更多查看译文
关键词
neural networks,infinite-depth,finite-width
AI 理解论文
溯源树
样例
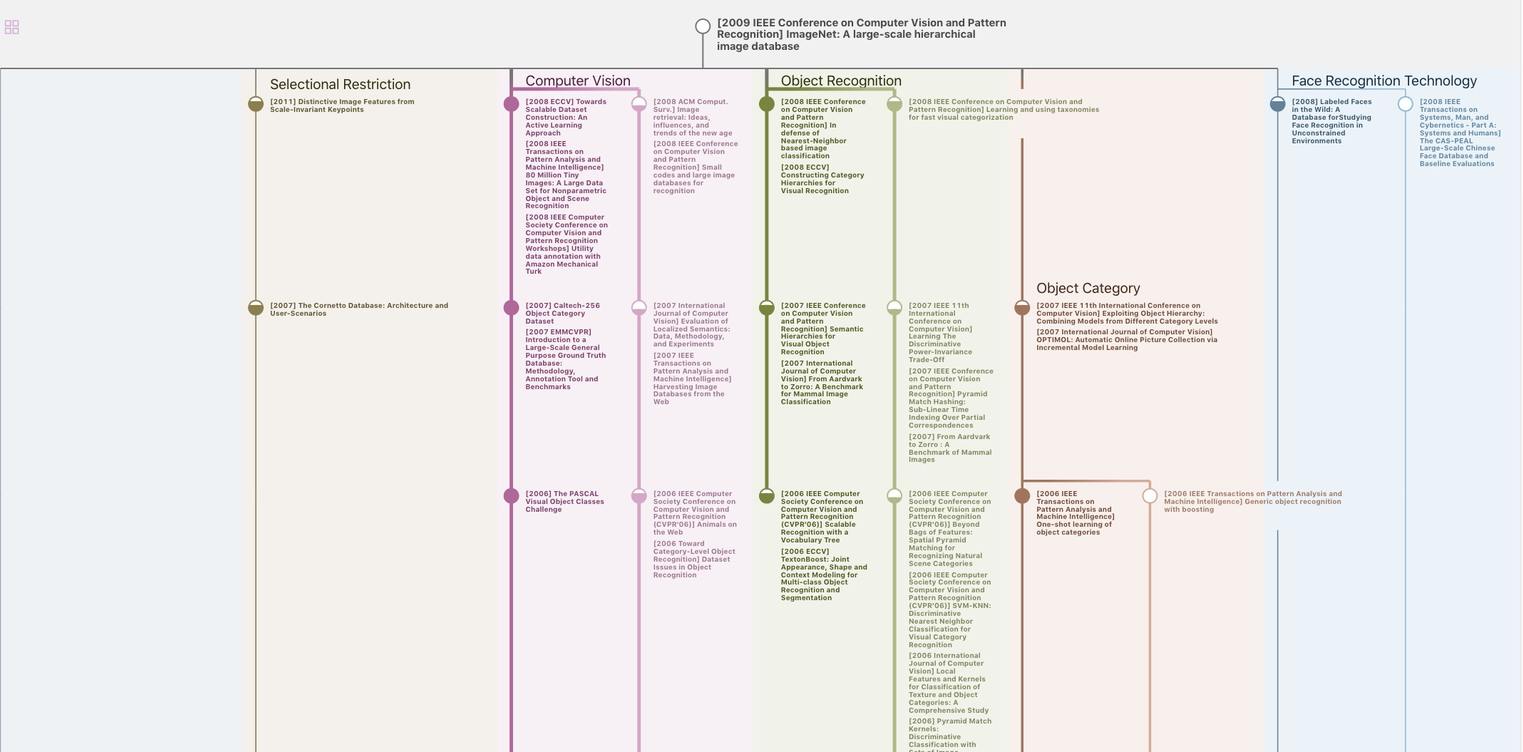
生成溯源树,研究论文发展脉络
Chat Paper
正在生成论文摘要