Interpreting convolutional neural networks' low dimensional approximation to quantum spin systems
arxiv(2022)
摘要
Convolutional neural networks (CNNs) have been employed along with Variational Monte Carlo methods for finding the ground state of quantum many-body spin systems with great success. In order to do so, however, a CNN with only linearly many variational parameters has to circumvent the ``curse of dimensionality'' and successfully approximate a wavefunction on an exponentially large Hilbert space. In our work, we provide a theoretical and experimental analysis of how the CNN optimizes learning for spin systems, and investigate the CNN's low dimensional approximation. We first quantify the role played by physical symmetries of the underlying spin system during training. We incorporate our insights into a new training algorithm and demonstrate its improved efficiency, accuracy and robustness. We then further investigate the CNN's ability to approximate wavefunctions by looking at the entanglement spectrum captured by the size of the convolutional filter. Our insights reveal the CNN to be an ansatz fundamentally centered around the occurrence statistics of $K$-motifs of the input strings. We use this motivation to provide the shallow CNN ansatz with a unifying theoretical interpretation in terms of other well-known statistical and physical ansatzes such as the maximum entropy (MaxEnt) and entangled plaquette correlator product states (EP-CPS). Using regression analysis, we find further relationships between the CNN's approximations of the different motifs' expectation values. Our results allow us to gain a comprehensive, improved understanding of how CNNs successfully approximate quantum spin Hamiltonians and to use that understanding to improve CNN performance.
更多查看译文
AI 理解论文
溯源树
样例
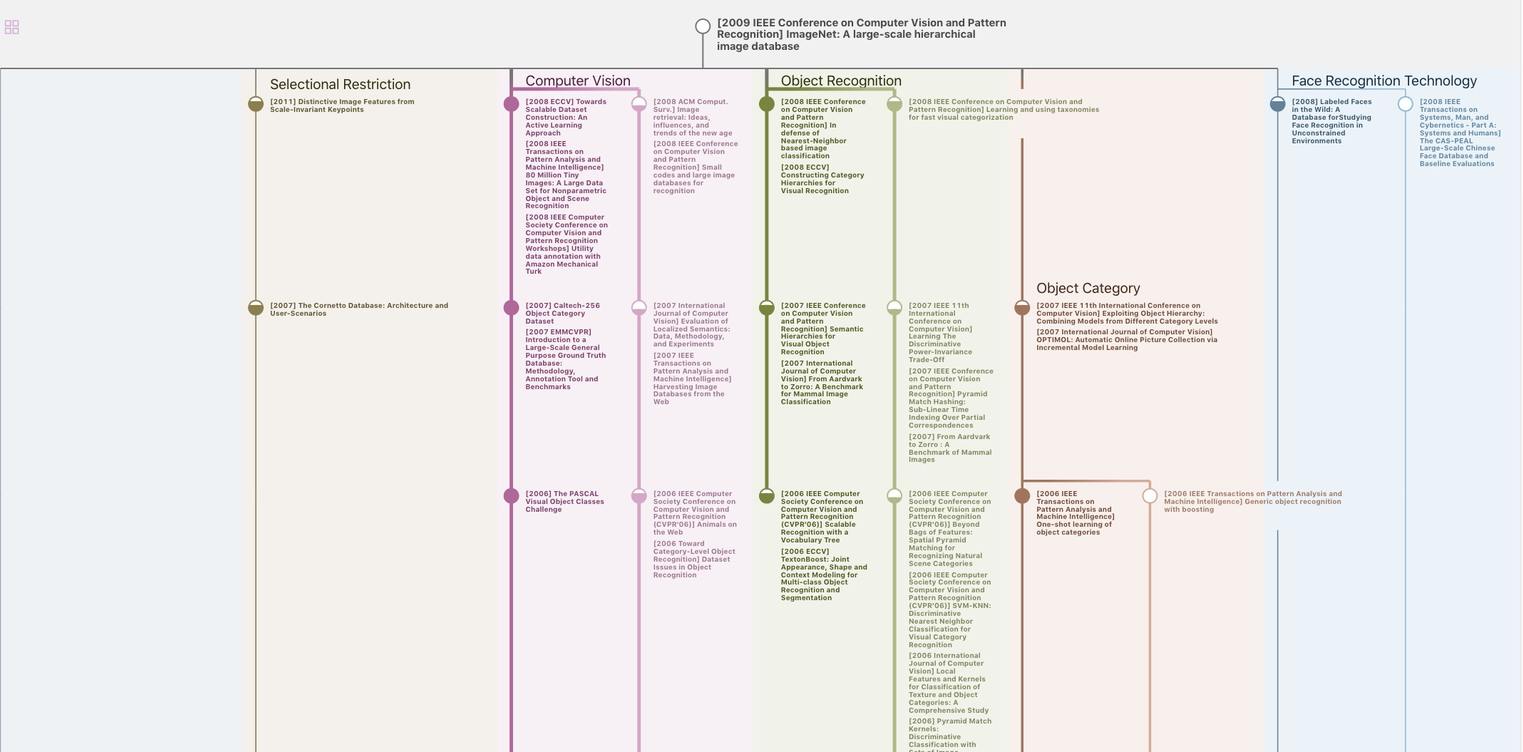
生成溯源树,研究论文发展脉络
Chat Paper
正在生成论文摘要