Generative Category-Level Shape and Pose Estimation with Semantic Primitives
arxiv(2023)
摘要
Empowering autonomous agents with 3D understanding for daily objects is a grand challenge in robotics applications. When exploring in an unknown environment, existing methods for object pose estimation are still not satisfactory due to the diversity of object shapes. In this paper, we propose a novel framework for category-level object shape and pose estimation from a single RGB-D image. To handle the intra-category variation, we adopt a semantic primitive representation that encodes diverse shapes into a unified latent space, which is the key to establish reliable correspondences between observed point clouds and estimated shapes. Then, by using a SIM(3)-invariant shape descriptor, we gracefully decouple the shape and pose of an object, thus supporting latent shape optimization of target objects in arbitrary poses. Extensive experiments show that the proposed method achieves SOTA pose estimation performance and better generalization in the real-world dataset. Code and video are available at https://zju3dv.github.io/gCasp.
更多查看译文
关键词
pose estimation,shape,primitives,category-level
AI 理解论文
溯源树
样例
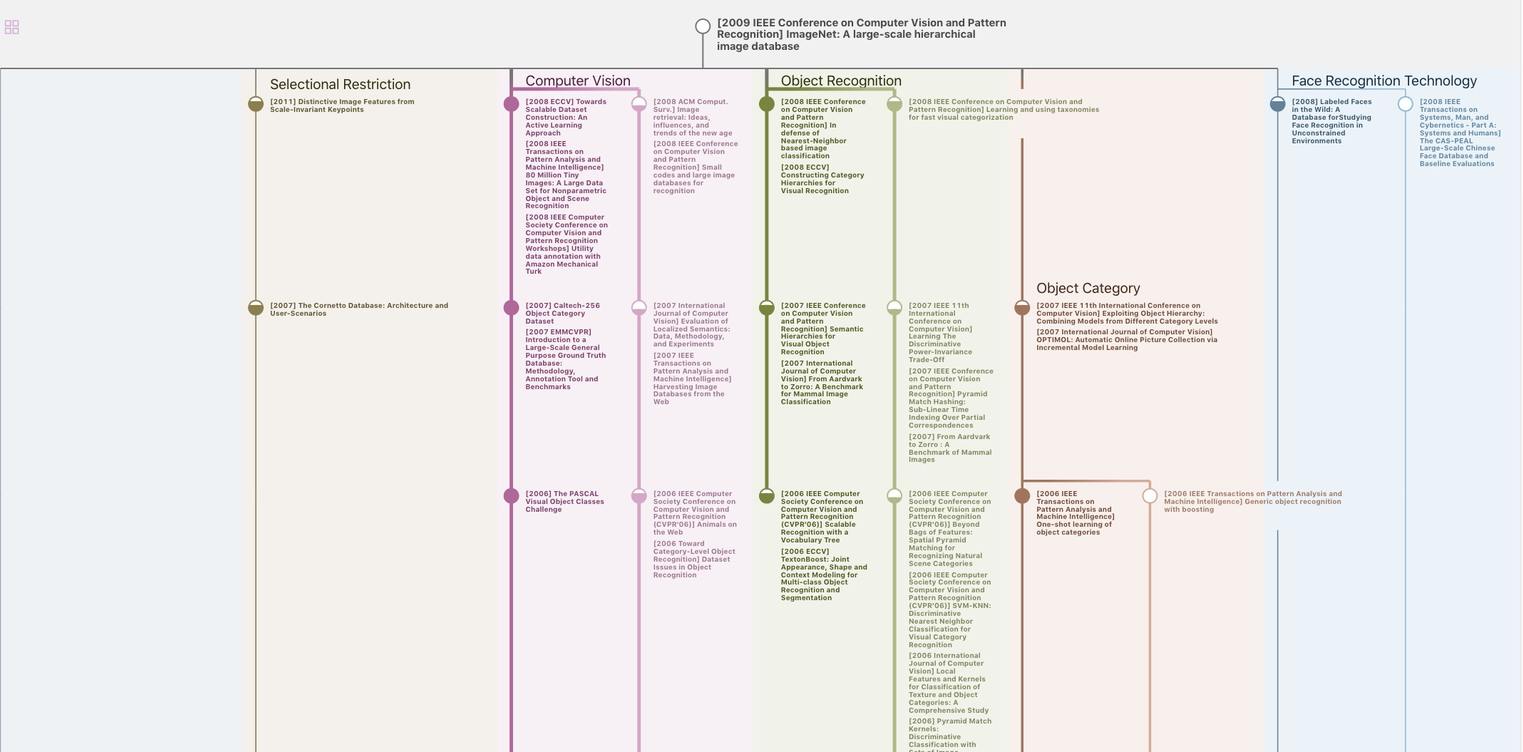
生成溯源树,研究论文发展脉络
Chat Paper
正在生成论文摘要