An XGBoost approach for industrial component degradation classification
2022 33rd Irish Signals and Systems Conference (ISSC)(2022)
摘要
This paper presents a machine learning algorithm that can classify discrete degradation states of an industrial component (a biopharma elastomer valve membrane) based on process data collected as part of a smart manufacturing process. The deterioration of the elastomer is driven by a combination of the effects of steam, chemical and hot water exposure - as well as the duration of exposure to each stressor. Failure of the elastomer can lead to complete valve failure and, in extreme circumstances, process contamination. Here, an XG Boost-based algorithm with the ability to serve as a predictive maintenance process, based on empirical process data, is portrayed. Recorded process-condition data gathered from elastomer routine preventative maintenance serves as an input dataset to train baseline predictive models in order to develop an algorithm with the capacity to forecast the remaining useful life (RUL) for the elastomer membrane. A correlation analysis was initially used to find the best candidate data streams to act as input features for a machine learning approach. Subsequent experimental analysis with the feature selection dataset concluded that an XG Boost-based algorithm yielded the required model performance for predicting the degree of depletion. Additionally, the developed model has the capacity to forecast the elemental state transition probability, where the elastomer transitions from class 1 ‘mildly degraded’ to class 2 ‘moderately degraded’. This trained algorithm can be used to perform classification on a new static dataset coming in to replace and / or supplement expert visual information.
更多查看译文
关键词
material degradation classification,valve elastomer diaphragm,XGBoost,predictive maintenance
AI 理解论文
溯源树
样例
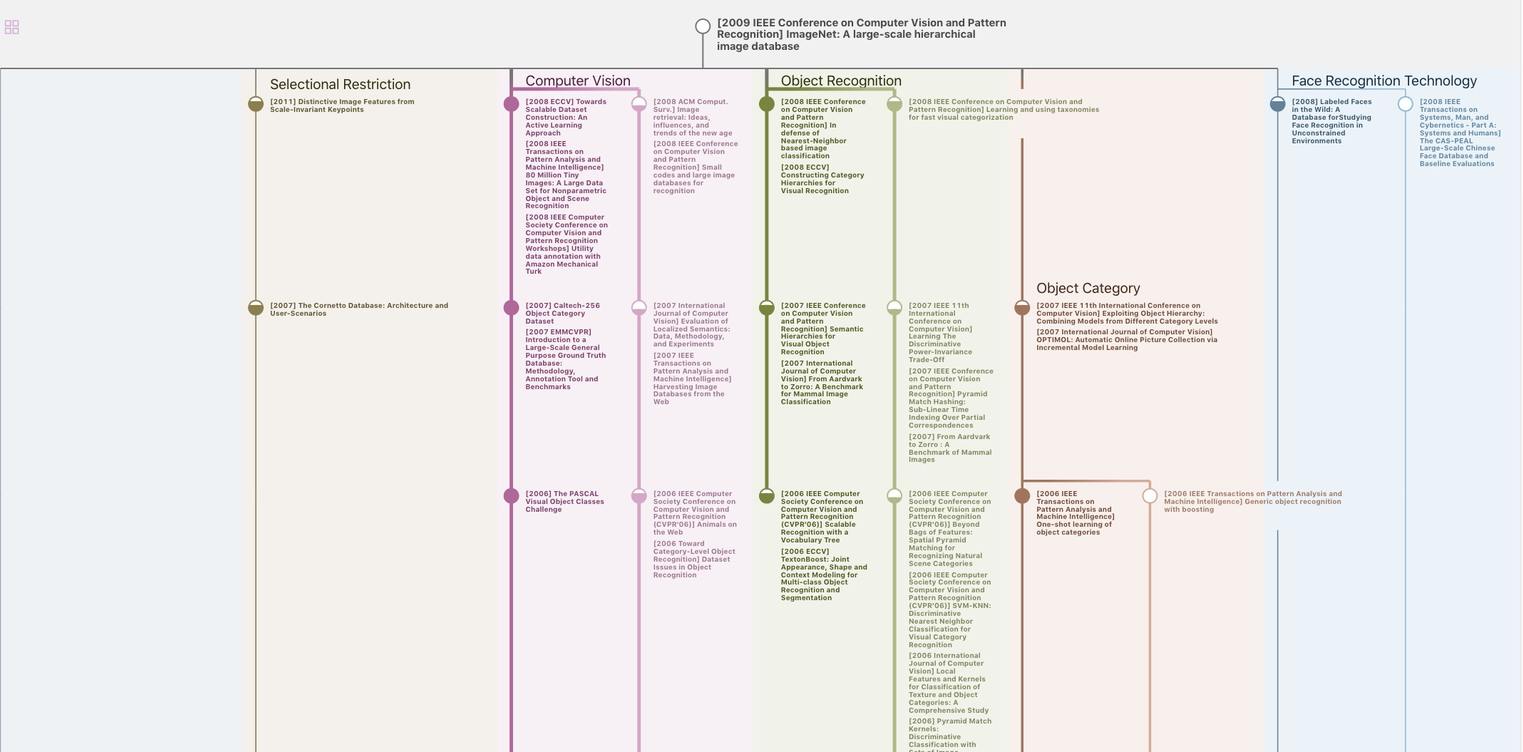
生成溯源树,研究论文发展脉络
Chat Paper
正在生成论文摘要