A differential evolution with reinforcement learning for multi-objective assembly line feeding problem.
Comput. Ind. Eng.(2022)
摘要
This paper studies a multi-objective assembly line feeding problem (MALFP), which is a new variant of the as-sembly line feeding problem in automobile manufacturers. In this problem, part families are delivered through five feeding policies to minimize three objectives simultaneously. To describe the problem, a novel multi -objective mathematical model is formulated. It not only overcomes the difficulty of determining perfect weights for objectives without prior knowledge, but also complements the traditional model by considering extended decisions on receiving warehouses, an extra cost item for policy switching, and a hybrid inventory strategy. To solve the problem, an innovative multi-objective differential evolution with a reinforcement learning (RL) based operator selection mechanism (MODE-RLOSM) is proposed. By solving MALFP with MODE-RLOSM, near-optimal candidate solutions that are suitable for different working conditions are provided to managers for making trade-offs and implementations. Compared with state-of-the-art optimization algorithms as well as a practical decision tree approach, the proposed algorithm shows superiority in cost saving, solution quality, and convergence efficiency. Through ablation study, sensitivity analysis, and RL behavior analysis, we investigate components in MODE-RLOSM and verify their effectiveness and robustness. In addition to bringing significant cost savings, the obtained solution also gives us production enlightenment and thus improves the decision -making efficiency of the enterprise. In our research, we illustrate the influence of part diversity on policy se-lection, give managers suggestions under different objective preferences, and find it uneconomical to pursue a specific objective excessively.
更多查看译文
关键词
Assembly line feeding problem,Multi-objective optimization,Differential evolution algorithm,Reinforcement learning
AI 理解论文
溯源树
样例
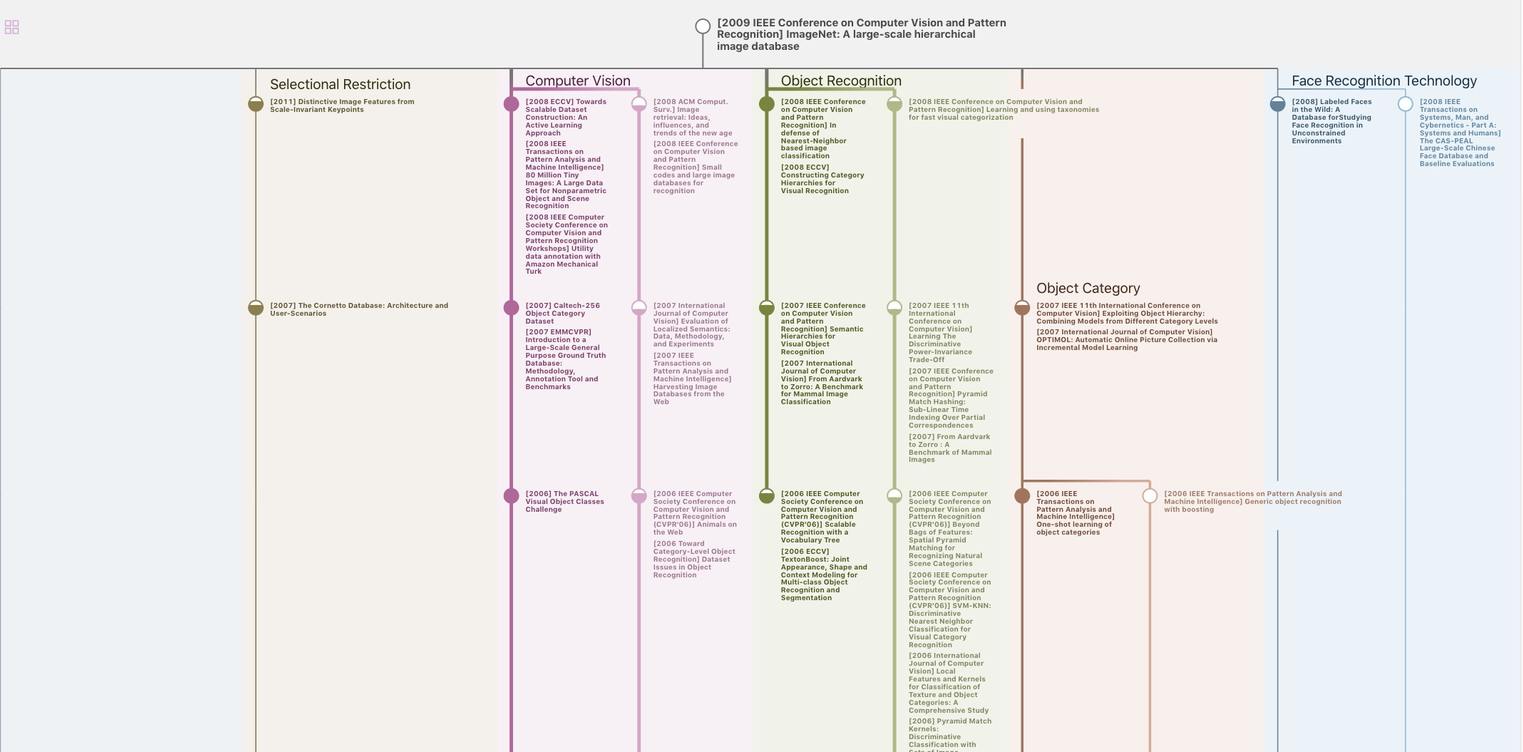
生成溯源树,研究论文发展脉络
Chat Paper
正在生成论文摘要