HAL-X: Scalable hierarchical clustering for rapid and tunable single-cell analysis.
PLoS computational biology(2022)
摘要
Data clustering plays a significant role in biomedical sciences, particularly in single-cell data analysis. Researchers use clustering algorithms to group individual cells into populations that can be evaluated across different levels of disease progression, drug response, and other clinical statuses. In many cases, multiple sets of clusters must be generated to assess varying levels of cluster specificity. For example, there are many subtypes of leukocytes (e.g. T cells), whose individual preponderance and phenotype must be assessed for statistical/functional significance. In this report, we introduce a novel hierarchical density clustering algorithm (HAL-x) that uses supervised linkage methods to build a cluster hierarchy on raw single-cell data. With this new approach, HAL-x can quickly predict multiple sets of labels for immense datasets, achieving a considerable improvement in computational efficiency on large datasets compared to existing methods. We also show that cell clusters generated by HAL-x yield near-perfect F1-scores when classifying different clinical statuses based on single-cell profiles. Our hierarchical density clustering algorithm achieves high accuracy in single cell classification in a scalable, tunable and rapid manner.
更多查看译文
关键词
scalable hierarchical clustering,single-cell
AI 理解论文
溯源树
样例
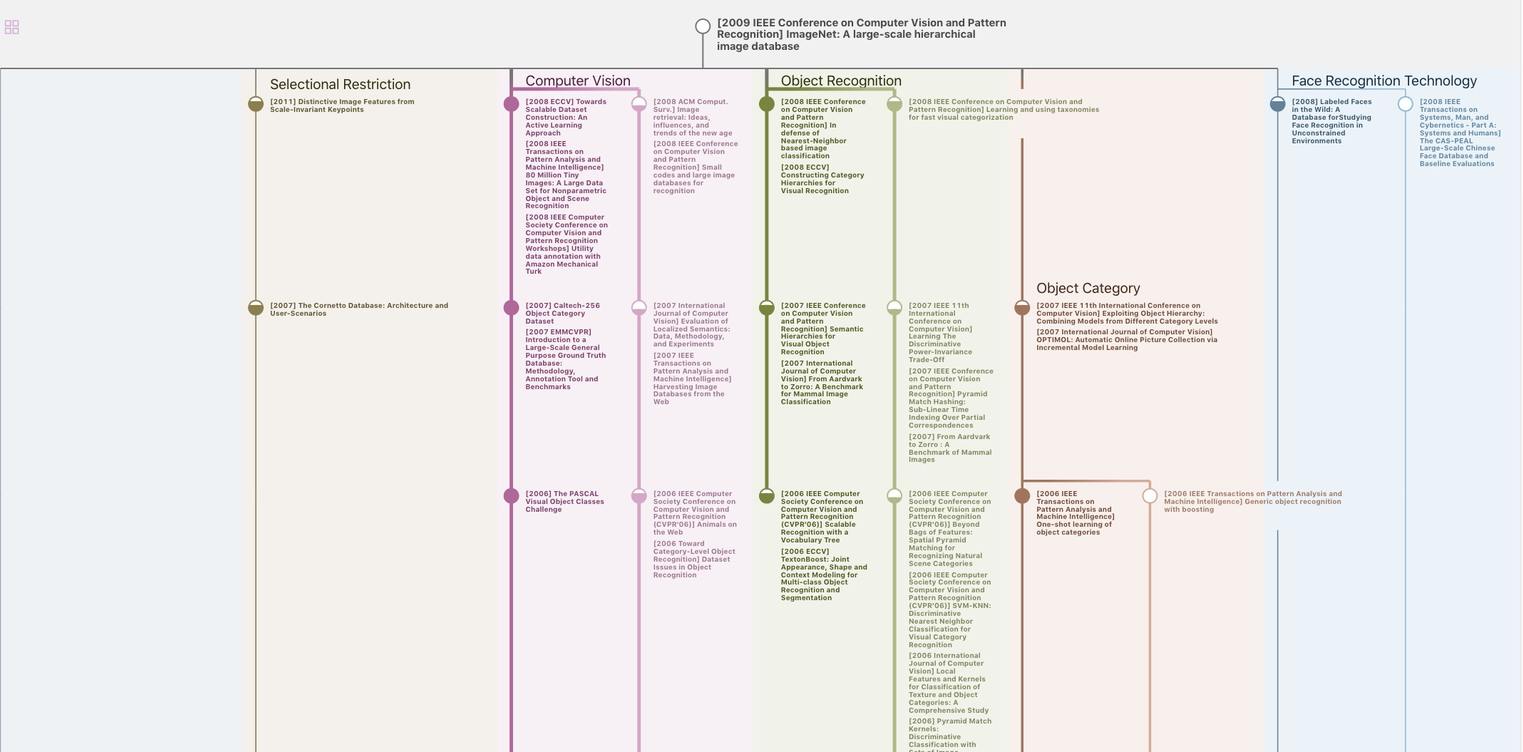
生成溯源树,研究论文发展脉络
Chat Paper
正在生成论文摘要