Post-typhoon forest damage estimation using multiple vegetation indices and machine learning models
Weather and Climate Extremes(2022)
摘要
The frequency and intensity of typhoons have increased due to climate change. These climate change-induced disasters have caused widespread damage to forests. Evaluation of the effects of typhoons on forest ecosystems is often complex and challenging, mainly because of their sporadic nature. In this paper, we compared existing forest damage estimation techniques with the goal of identifying their respective advantages and suitable use cases. We considered Hokkaido in northern Japan as a case study, where three typhoons successively struck in 2016 and led to forest destruction. Forest damage was estimated from Landsat 8 imagery by three approaches, namely using vegetation damage indices (DVDI, DNDVI and ΔEVI), using supervised classification with Random Forest (RF) and Support Vector Machines (SVM) and finally by using the commercial CLASlite software with built-in methods to detect forest disturbance. Machine learning classifiers obtained the highest damage assessment accuracy, but intensive computation and complex processing steps were required. The RF and SVM classifiers gave the highest accuracies when using Fractional Cover as a predictor variable (Overall Accuracy = 80.36% in both cases, and ROC AUC values of 0.89 and 0.88, respectively.) Among the vegetation damage indices, DNDVI produced the highest accuracy (AUC = 0.85, OA = 77.68%).
更多查看译文
关键词
Forest damage,Remote sensing,Vegetation indices,Multispectral classification,CLASlite
AI 理解论文
溯源树
样例
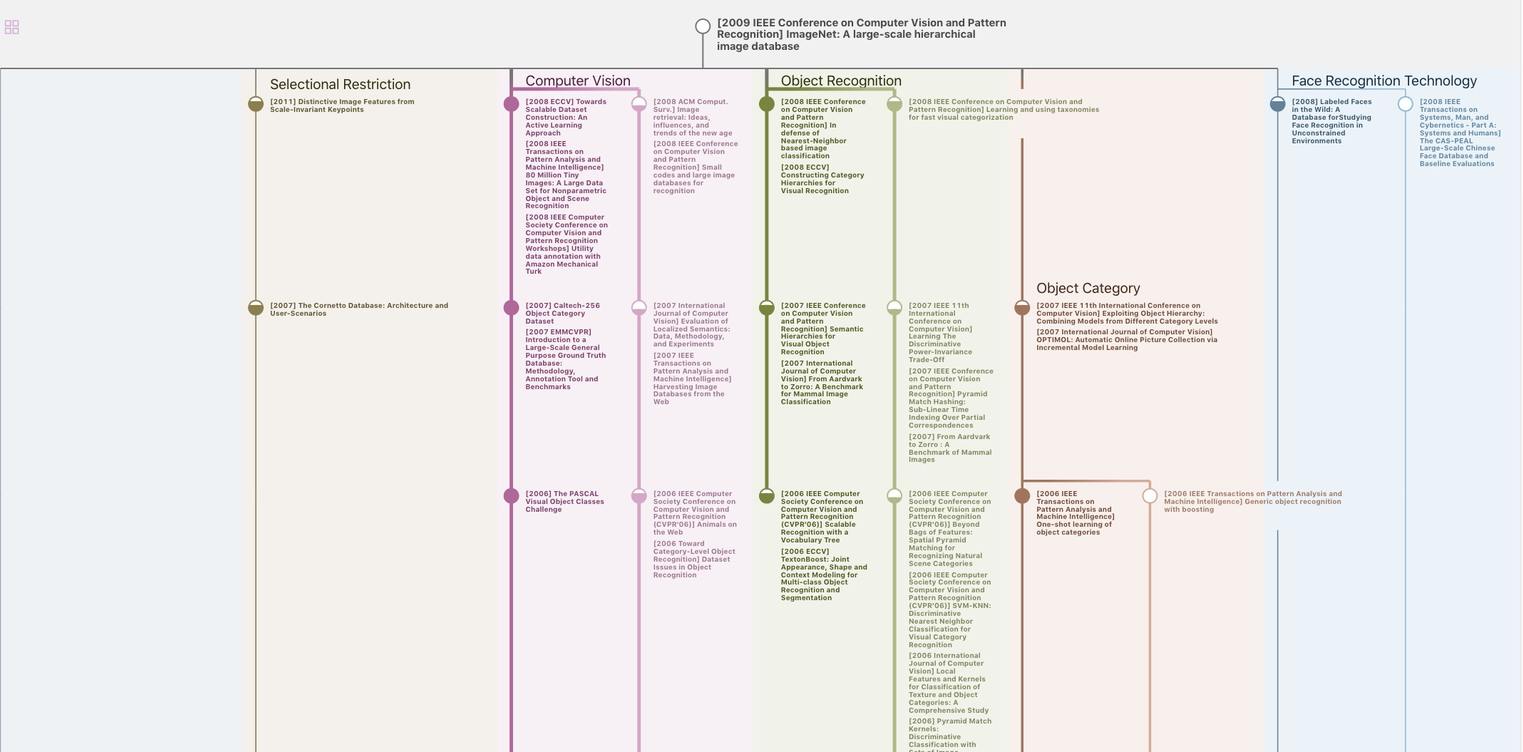
生成溯源树,研究论文发展脉络
Chat Paper
正在生成论文摘要